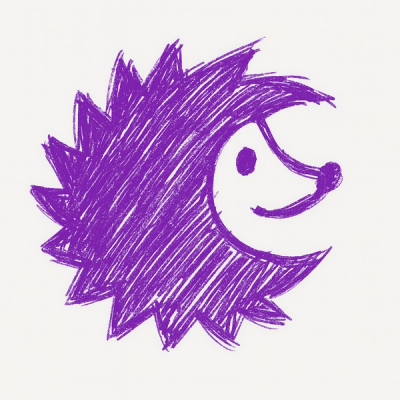
Security News
Browserslist-rs Gets Major Refactor, Cutting Binary Size by Over 1MB
Browserslist-rs now uses static data to reduce binary size by over 1MB, improving memory use and performance for Rust-based frontend tools.
Pydantic models for STAC Catalogs, Collections, Items, and the STAC API spec. Initially developed by arturo-ai.
The main purpose of this library is to provide reusable request/response models for tools such as fastapi. For more comprehensive schema validation and robust extension support, use pystac.
python -m pip install stac-pydantic
# or
python -m pip install stac-pydantic["validation"]
For local development:
python -m pip install -e '.[dev,lint]'
stac-pydantic | STAC Version | STAC API Version | Pydantic Version |
---|---|---|---|
1.2.x | 1.0.0-beta.1 | <1* | ^1.6 |
1.3.x | 1.0.0-beta.2 | <1* | ^1.6 |
2.0.x | 1.0.0 | <1* | ^1.6 |
3.0.x | 1.0.0 | 1.0.0 | ^2.4 |
3.1.x | 1.0.0 | 1.0.0 | ^2.4 |
* various beta releases, specs not fully implemented
Install the pre-commit hooks:
pre-commit install
Ensure you have all Python versions installed that the tests will be run against. If using pyenv, run:
pyenv install 3.8.18
pyenv install 3.9.18
pyenv install 3.10.13
pyenv install 3.11.5
pyenv local 3.8.18 3.9.18 3.10.13 3.11.5
Run the entire test suite:
tox
Run a single test case using the standard pytest convention:
python -m pytest -v tests/test_models.py::test_item_extensions
Load data into models with standard pydantic:
from stac_pydantic import Catalog
stac_catalog = {
"type": "Catalog",
"stac_version": "1.0.0",
"id": "sample",
"description": "This is a very basic sample catalog.",
"links": [
{
"href": "item.json",
"rel": "item"
}
]
}
catalog = Catalog(**stac_catalog)
assert catalog.id == "sample"
assert catalog.links[0].href == "item.json"
STAC defines many extensions which let the user customize the data in their catalog. stac-pydantic.extensions.validate_extensions
gets the JSON schemas from the URLs provided in the stac_extensions
property (caching the last fetched ones), and will validate a dict
, Item
, Collection
or Catalog
against those fetched schemas:
from stac_pydantic import Item
from stac_pydantic.extensions import validate_extensions
stac_item = {
"id": "12345",
"type": "Feature",
"stac_extensions": [
"https://stac-extensions.github.io/eo/v1.0.0/schema.json"
],
"geometry": { "type": "Point", "coordinates": [0, 0] },
"bbox": [0.0, 0.0, 0.0, 0.0],
"properties": {
"datetime": "2020-03-09T14:53:23.262208+00:00",
"eo:cloud_cover": 25,
},
"links": [],
"assets": {},
}
model = Item(**stac_item)
validate_extensions(model, reraise_exception=True)
assert getattr(model.properties, "eo:cloud_cover") == 25
The complete list of current STAC Extensions can be found here.
The same procedure described above works for any STAC Extension schema as long as it can be loaded from a public url.
The STAC API Specs extent the core STAC specification for implementing dynamic catalogs. STAC Objects used in an API context should always import models from the api
subpackage. This package extends
Catalog, Collection, and Item models with additional fields and validation rules and introduces Collections and ItemCollections models and Pagination/ Search Links.
It also implements models for defining ItemSeach queries.
from stac_pydantic.api import Item, ItemCollection
stac_item = Item(**{
"id": "12345",
"type": "Feature",
"stac_extensions": [],
"geometry": { "type": "Point", "coordinates": [0, 0] },
"bbox": [0.0, 0.0, 0.0, 0.0],
"properties": {
"datetime": "2020-03-09T14:53:23.262208+00:00",
},
"collection": "CS3",
"links": [
{
"rel": "self",
"href": "http://stac.example.com/catalog/collections/CS3-20160503_132130_04/items/CS3-20160503_132130_04.json"
},
{
"rel": "collection",
"href": "http://stac.example.com/catalog/CS3-20160503_132130_04/catalog.json"
},
{
"rel": "root",
"href": "http://stac.example.com/catalog"
}],
"assets": {},
})
stac_item_collection = ItemCollection(**{
"type": "FeatureCollection",
"features": [stac_item],
"links": [
{
"rel": "self",
"href": "http://stac.example.com/catalog/search?collection=CS3",
"type": "application/geo+json"
},
{
"rel": "root",
"href": "http://stac.example.com/catalog",
"type": "application/json"
}],
})
Most STAC extensions are namespaced with a colon (ex eo:gsd
) to keep them distinct from other extensions. Because
Python doesn't support the use of colons in variable names, we use Pydantic aliasing
to add the namespace upon model export. This requires exporting
the model with the by_alias = True
parameter. Export methods (model_dump()
and model_dump_json()
) for models in this library have by_alias
and exclude_unset
st to True
by default:
item_dict = item.model_dump()
assert item_dict['properties']['landsat:row'] == item.properties.row == 250
Usage: stac-pydantic [OPTIONS] COMMAND [ARGS]...
stac-pydantic cli group
Options:
--help Show this message and exit.
Commands:
validate-item Validate STAC Item
FAQs
Pydantic data models for the STAC spec
We found that stac-pydantic demonstrated a healthy version release cadence and project activity because the last version was released less than a year ago. It has 4 open source maintainers collaborating on the project.
Did you know?
Socket for GitHub automatically highlights issues in each pull request and monitors the health of all your open source dependencies. Discover the contents of your packages and block harmful activity before you install or update your dependencies.
Security News
Browserslist-rs now uses static data to reduce binary size by over 1MB, improving memory use and performance for Rust-based frontend tools.
Research
Security News
Eight new malicious Firefox extensions impersonate games, steal OAuth tokens, hijack sessions, and exploit browser permissions to spy on users.
Security News
The official Go SDK for the Model Context Protocol is in development, with a stable, production-ready release expected by August 2025.