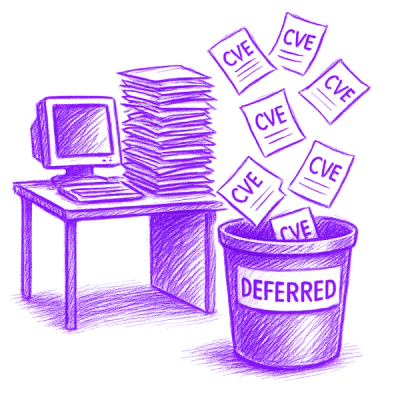
Security News
NVD Quietly Sweeps 100K+ CVEs Into a “Deferred” Black Hole
NVD now marks all pre-2018 CVEs as "Deferred," signaling it will no longer enrich older vulnerabilities, further eroding trust in its data.
|build| |rtd| |codecov| |pypi| |pyversions|
.. |build| image:: https://github.com/crflynn/stochastic/actions/workflows/build.yml/badge.svg :target: https://github.com/crflynn/stochastic/actions
.. |rtd| image:: https://img.shields.io/readthedocs/stochastic.svg :target: http://stochastic.readthedocs.io/en/latest/
.. |codecov| image:: https://codecov.io/gh/crflynn/stochastic/branch/master/graphs/badge.svg :target: https://codecov.io/gh/crflynn/stochastic
.. |pypi| image:: https://img.shields.io/pypi/v/stochastic.svg :target: https://pypi.python.org/pypi/stochastic
.. |pyversions| image:: https://img.shields.io/pypi/pyversions/stochastic.svg :target: https://pypi.python.org/pypi/stochastic
A python package for generating realizations of stochastic processes.
The stochastic
package is available on pypi and can be installed using pip
.. code-block:: shell
pip install stochastic
Dependencies
Stochastic uses ``numpy`` for many calculations and ``scipy`` for sampling
specific random variables.
Processes
---------
This package offers a number of common discrete-time, continuous-time, and
noise process objects for generating realizations of stochastic processes as
``numpy`` arrays.
The diffusion processes are approximated using the Euler–Maruyama method.
Here are the currently supported processes and their class references within
the package.
* stochastic.processes
* continuous
* BesselProcess
* BrownianBridge
* BrownianExcursion
* BrownianMeander
* BrownianMotion
* CauchyProcess
* FractionalBrownianMotion
* GammaProcess
* GeometricBrownianMotion
* InverseGaussianProcess
* MixedPoissonProcess
* MultifractionalBrownianMotion
* PoissonProcess
* SquaredBesselProcess
* VarianceGammaProcess
* WienerProcess
* diffusion
* DiffusionProcess (generalized)
* ConstantElasticityVarianceProcess
* CoxIngersollRossProcess
* ExtendedVasicekProcess
* OrnsteinUhlenbeckProcess
* VasicekProcess
* discrete
* BernoulliProcess
* ChineseRestaurantProcess
* DirichletProcess
* MarkovChain
* MoranProcess
* RandomWalk
* noise
* BlueNoise
* BrownianNoise
* ColoredNoise
* PinkNoise
* RedNoise
* VioletNoise
* WhiteNoise
* FractionalGaussianNoise
* GaussianNoise
Usage patterns
--------------
Sampling
~~~~~~~~
To use ``stochastic``, import the process you want and instantiate with the
required parameters. Every process class has a ``sample`` method for generating
realizations. The ``sample`` methods accept a parameter ``n`` for the quantity
of steps in the realization, but others (Poisson, for instance) may take
additional parameters. Parameters can be accessed as attributes of the
instance.
.. code-block:: python
from stochastic.processes.discrete import BernoulliProcess
bp = BernoulliProcess(p=0.6)
s = bp.sample(16)
success_probability = bp.p
Continuous processes provide a default parameter, ``t``, which indicates the
maximum time of the process realizations. The default value is 1. The sample
method will generate ``n`` equally spaced increments on the
interval ``[0, t]``.
Sampling at specific times
Some continuous processes also provide a sample_at()
method, in which a
sequence of time values can be passed at which the object will generate a
realization. This method ignores the parameter, t
, specified on
instantiation.
.. code-block:: python
from stochastic.processes.continuous import BrownianMotion
bm = BrownianMotion(drift=1, scale=1, t=1)
times = [0, 3, 10, 11, 11.2, 20]
s = bm.sample_at(times)
Sample times
Continuous processes also provide a method ``times()`` which generates the time
values (using ``numpy.linspace``) corresponding to a realization of ``n``
steps. This is particularly useful for plotting your samples.
.. code-block:: python
import matplotlib.pyplot as plt
from stochastic.processes.continuous import FractionalBrownianMotion
fbm = FractionalBrownianMotion(hurst=0.7, t=1)
s = fbm.sample(32)
times = fbm.times(32)
plt.plot(times, s)
plt.show()
Specifying an algorithm
Some processes provide an optional parameter algorithm
, in which one can
specify which algorithm to use to generate the realization using the
sample()
or sample_at()
methods. See the documentation for
process-specific implementations.
.. code-block:: python
from stochastic.processes.noise import FractionalGaussianNoise
fgn = FractionalGaussianNoise(hurst=0.6, t=1)
s = fgn.sample(32, algorithm='hosking')
FAQs
Generate realizations of stochastic processes
We found that stochastic demonstrated a healthy version release cadence and project activity because the last version was released less than a year ago. It has 1 open source maintainer collaborating on the project.
Did you know?
Socket for GitHub automatically highlights issues in each pull request and monitors the health of all your open source dependencies. Discover the contents of your packages and block harmful activity before you install or update your dependencies.
Security News
NVD now marks all pre-2018 CVEs as "Deferred," signaling it will no longer enrich older vulnerabilities, further eroding trust in its data.
Research
Security News
Lazarus-linked threat actors expand their npm malware campaign with new RAT loaders, hex obfuscation, and over 5,600 downloads across 11 packages.
Security News
Safari 18.4 adds support for Iterator Helpers and two other TC39 JavaScript features, bringing full cross-browser coverage to key parts of the ECMAScript spec.