
SuperTriplets
SuperTriplets is a toolbox for supervised online hard triplet learning, currently supporting different kinds of data: text, image, and even text + image (multimodal).
It doesn't try to automate the training and evaluation loop for you. Instead, it provides useful PyTorch-based utilities you can couple to your existing code, making the process as easy as performing other everyday supervised learning tasks, such as classification and regression.
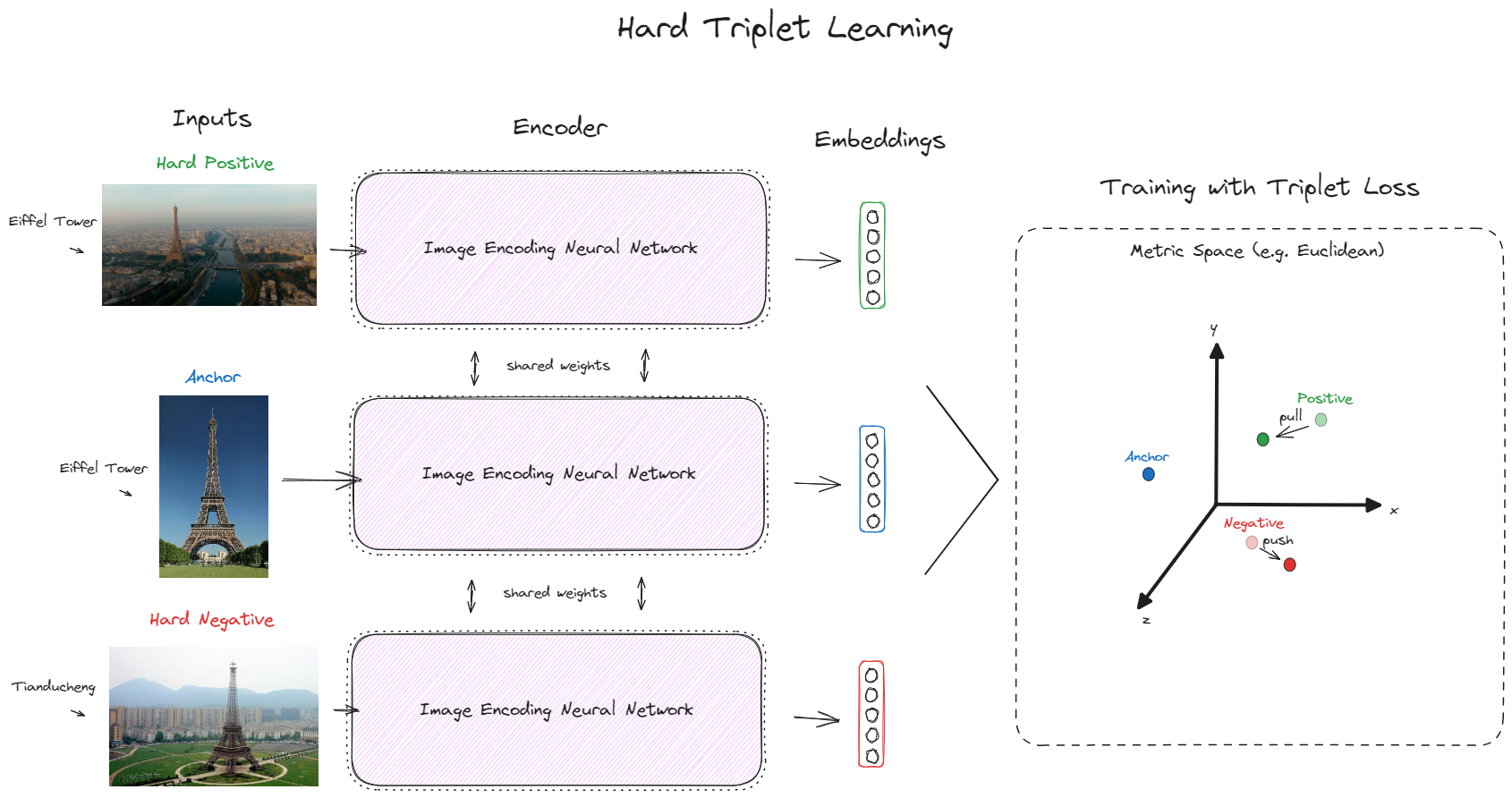
Installation and Supported Versions
SuperTriplets is available on PyPI:
$ pip install supertriplets
SuperTriplets officially supports Python 3.8+.
Quick Start
Training
Update your model weights with batch hard triplet losses over label balanced batches:
import torch
from supertriplets.dataset import OnlineTripletsDataset
from supertriplets.distance import EuclideanDistance
from supertriplets.loss import BatchHardTripletLoss
from supertriplets.sample import TextImageSample
device = 'cuda:0' if torch.cuda.is_available() else 'cpu'
train_examples = [
TextImageSample(text=text, image_path=image_path, label=label)
for text, image_path, label in zip(train_df['text'], train_df['image_path'], train_df['label'])
]
def my_sample_loading_func(text, image_path, label, *args, **kwargs):
loaded_sample = {"text_input": prep_text, "image_input": prep_image, "label": prep_label}
return loaded_sample
train_dataset = OnlineTripletsDataset(
examples=train_examples,
in_batch_num_samples_per_label=2,
batch_size=32,
sample_loading_func=my_sample_loading_func,
sample_loading_kwargs={}
)
train_dataloader = DataLoader(dataset=train_dataset, batch_size=32, num_workers=0, drop_last=True)
criterion = BatchHardTripletLoss(distance=EuclideanDistance(squared=False), margin=5)
model =
optimizer =
num_epochs =
for epoch in range(1, num_epochs + 1):
for batch in train_dataloader:
data = batch["samples"]
labels = move_tensors_to_device(obj=data.pop("label"), device=device)
inputs = move_tensors_to_device(obj=data, device=device)
optimizer.zero_grad()
embeddings = model(**inputs)
loss = criterion(embeddings=embeddings, labels=labels)
loss.backward()
optimizer.step()
Evaluation
Mine hard triplets with pretrained models to construct your static testing dataset:
import torch
from supertriplets.sample import TextImageSample
from supertriplets.encoder import PretrainedSampleEncoder
from supertriplets.evaluate import HardTripletsMiner
from supertriplets.dataset import StaticTripletsDataset
device = 'cuda:0' if torch.cuda.is_available() else 'cpu'
test_examples = [
TextImageSample(text=text, image_path=image_path, label=label)
for text, image_path, label in zip(test_df['text'], test_df['image_path'], test_df['label'])
]
pretrained_encoder = PretrainedSampleEncoder(modality="text_english-image")
test_embeddings = pretrained_encoder.encode(examples=test_examples, device=device, batch_size=32)
hard_triplet_miner = HardTripletsMiner(use_gpu_powered_index_if_available=True)
test_anchors, test_positives, test_negatives = hard_triplet_miner.mine(
examples=test_examples, embeddings=test_embeddings, normalize_l2=True, sample_from_topk_hardest=10
)
def my_sample_loading_func(text, image_path, label, *args, **kwargs):
loaded_sample = {"text_input": prep_text, "image_input": prep_image, "label": prep_label}
return loaded_sample
test_dataset = StaticTripletsDataset(
anchor_examples=test_anchor_examples,
positive_examples=test_positive_examples,
negative_examples=test_negative_examples,
sample_loading_func=my_sample_loading_func,
sample_loading_kwargs={}
)
Easily create a good baseline with pretrained models and utilities to measure model accuracies on triplets using a diverse set of distance measurements:
from torch.utils.data import DataLoader
from tqdm import tqdm
from supertriplets.evaluate import TripletEmbeddingsEvaluator
from supertriplets.models import load_pretrained_model
from supertriplets.utils import move_tensors_to_device
model = load_pretrained_model(model_name="CLIPViTB32EnglishEncoder")
model.to(device)
model.eval()
test_dataloader = DataLoader(dataset=test_dataset, batch_size=32, shuffle=False, num_workers=0, drop_last=False)
def get_triplet_embeddings(dataloader, model, device):
model.eval()
embeddings = {"anchors": [], "positives": [], "negatives": []}
with torch.no_grad():
for batch in tqdm(dataloader, total=len(dataloader)):
for input_type in ["anchors", "positives", "negatives"]:
inputs = {k: v for k, v in batch[input_type].items() if k != "label"}
inputs = move_tensors_to_device(obj=inputs, device=device)
batch_embeddings = model(**inputs).cpu()
embeddings[input_type].append(batch_embeddings)
embeddings = {k: torch.cat(v, dim=0).numpy() for k, v in embeddings.items()}
return embeddings
triplet_embeddings_evaluator = TripletEmbeddingsEvaluator(
calculate_by_cosine=True, calculate_by_manhattan=True, calculate_by_euclidean=True
)
test_triplet_embeddings = get_triplet_embeddings(dataloader=test_dataloader, model=model, device=device)
test_baseline_accuracies = triplet_embeddings_evaluator.evaluate(
embeddings_anchors=test_triplet_embeddings["anchors"],
embeddings_positives=test_triplet_embeddings["positives"],
embeddings_negatives=test_triplet_embeddings["negatives"],
)
Local Development
Make sure you have python3, python3-venv and make installed.
Create a virtual environment with an editable installation of SuperTriplets and development specific dependencies by running:
$ make install
Activate .venv
:
$ source .venv/bin/activate
Now you can make changes and test them with pytest.
Testing without a GPU:
$ python -m pytest -k "not test_tinymmimdb_convergence"
With a GPU:
$ python -m pytest
Changelog
See CHANGELOG.md for news on all SuperTriplets versions.
License
See LICENSE for the current license.