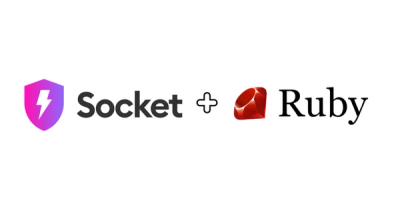
Product
Rubygems Ecosystem Support Now Generally Available
Socket's Rubygems ecosystem support is moving from beta to GA, featuring enhanced security scanning to detect supply chain threats beyond traditional CVEs in your Ruby dependencies.
A comprehensive library to post-train foundation models
TRL is a cutting-edge library designed for post-training foundation models using advanced techniques like Supervised Fine-Tuning (SFT), Proximal Policy Optimization (PPO), and Direct Preference Optimization (DPO). Built on top of the 🤗 Transformers ecosystem, TRL supports a variety of model architectures and modalities, and can be scaled-up across various hardware setups.
Trainers: Various fine-tuning methods are easily accessible via trainers like SFTTrainer
, GRPOTrainer
, DPOTrainer
, RewardTrainer
and more.
Efficient and scalable:
Command Line Interface (CLI): A simple interface lets you fine-tune with models without needing to write code.
Install the library using pip
:
pip install trl
If you want to use the latest features before an official release, you can install TRL from source:
pip install git+https://github.com/huggingface/trl.git
If you want to use the examples you can clone the repository with the following command:
git clone https://github.com/huggingface/trl.git
For more flexibility and control over training, TRL provides dedicated trainer classes to post-train language models or PEFT adapters on a custom dataset. Each trainer in TRL is a light wrapper around the 🤗 Transformers trainer and natively supports distributed training methods like DDP, DeepSpeed ZeRO, and FSDP.
SFTTrainer
Here is a basic example of how to use the SFTTrainer
:
from trl import SFTTrainer
from datasets import load_dataset
dataset = load_dataset("trl-lib/Capybara", split="train")
trainer = SFTTrainer(
model="Qwen/Qwen2.5-0.5B",
train_dataset=dataset,
)
trainer.train()
GRPOTrainer
GRPOTrainer
implements the Group Relative Policy Optimization (GRPO) algorithm that is more memory-efficient than PPO and was used to train Deepseek AI's R1.
from datasets import load_dataset
from trl import GRPOTrainer
dataset = load_dataset("trl-lib/tldr", split="train")
# Dummy reward function: count the number of unique characters in the completions
def reward_num_unique_chars(completions, **kwargs):
return [len(set(c)) for c in completions]
trainer = GRPOTrainer(
model="Qwen/Qwen2-0.5B-Instruct",
reward_funcs=reward_num_unique_chars,
train_dataset=dataset,
)
trainer.train()
DPOTrainer
DPOTrainer
implements the popular Direct Preference Optimization (DPO) algorithm that was used to post-train Llama 3 and many other models. Here is a basic example of how to use the DPOTrainer
:
from datasets import load_dataset
from transformers import AutoModelForCausalLM, AutoTokenizer
from trl import DPOConfig, DPOTrainer
model = AutoModelForCausalLM.from_pretrained("Qwen/Qwen2.5-0.5B-Instruct")
tokenizer = AutoTokenizer.from_pretrained("Qwen/Qwen2.5-0.5B-Instruct")
dataset = load_dataset("trl-lib/ultrafeedback_binarized", split="train")
training_args = DPOConfig(output_dir="Qwen2.5-0.5B-DPO")
trainer = DPOTrainer(
model=model,
args=training_args,
train_dataset=dataset,
processing_class=tokenizer
)
trainer.train()
RewardTrainer
Here is a basic example of how to use the RewardTrainer
:
from trl import RewardConfig, RewardTrainer
from datasets import load_dataset
from transformers import AutoModelForSequenceClassification, AutoTokenizer
tokenizer = AutoTokenizer.from_pretrained("Qwen/Qwen2.5-0.5B-Instruct")
model = AutoModelForSequenceClassification.from_pretrained(
"Qwen/Qwen2.5-0.5B-Instruct", num_labels=1
)
model.config.pad_token_id = tokenizer.pad_token_id
dataset = load_dataset("trl-lib/ultrafeedback_binarized", split="train")
training_args = RewardConfig(output_dir="Qwen2.5-0.5B-Reward", per_device_train_batch_size=2)
trainer = RewardTrainer(
args=training_args,
model=model,
processing_class=tokenizer,
train_dataset=dataset,
)
trainer.train()
You can use the TRL Command Line Interface (CLI) to quickly get started with post-training methods like Supervised Fine-Tuning (SFT) or Direct Preference Optimization (DPO):
SFT:
trl sft --model_name_or_path Qwen/Qwen2.5-0.5B \
--dataset_name trl-lib/Capybara \
--output_dir Qwen2.5-0.5B-SFT
DPO:
trl dpo --model_name_or_path Qwen/Qwen2.5-0.5B-Instruct \
--dataset_name argilla/Capybara-Preferences \
--output_dir Qwen2.5-0.5B-DPO
Read more about CLI in the relevant documentation section or use --help
for more details.
If you want to contribute to trl
or customize it to your needs make sure to read the contribution guide and make sure you make a dev install:
git clone https://github.com/huggingface/trl.git
cd trl/
pip install -e .[dev]
@misc{vonwerra2022trl,
author = {Leandro von Werra and Younes Belkada and Lewis Tunstall and Edward Beeching and Tristan Thrush and Nathan Lambert and Shengyi Huang and Kashif Rasul and Quentin Gallouédec},
title = {TRL: Transformer Reinforcement Learning},
year = {2020},
publisher = {GitHub},
journal = {GitHub repository},
howpublished = {\url{https://github.com/huggingface/trl}}
}
This repository's source code is available under the Apache-2.0 License.
FAQs
Train transformer language models with reinforcement learning.
We found that trl demonstrated a healthy version release cadence and project activity because the last version was released less than a year ago. It has 5 open source maintainers collaborating on the project.
Did you know?
Socket for GitHub automatically highlights issues in each pull request and monitors the health of all your open source dependencies. Discover the contents of your packages and block harmful activity before you install or update your dependencies.
Product
Socket's Rubygems ecosystem support is moving from beta to GA, featuring enhanced security scanning to detect supply chain threats beyond traditional CVEs in your Ruby dependencies.
Research
The Socket Research Team investigates a malicious npm package that appears to be an Advcash integration but triggers a reverse shell during payment success, targeting servers handling transactions.
Security Fundamentals
The Socket Threat Research Team uncovers how threat actors weaponize shell techniques across npm, PyPI, and Go ecosystems to maintain persistence and exfiltrate data.