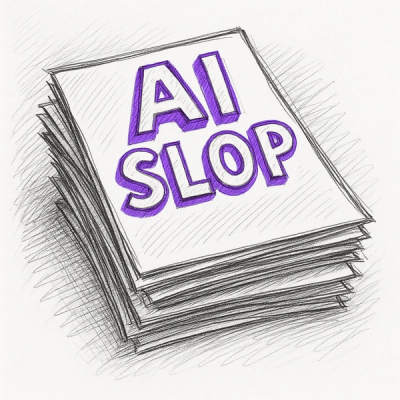
Security News
Django Joins curl in Pushing Back on AI Slop Security Reports
Django has updated its security policies to reject AI-generated vulnerability reports that include fabricated or unverifiable content.
Intelligent Market Monitoring
The pipeline for monitoring the market has the folling main steps:
python3.11 -m venv .venv
source .venv/bin/activate
pip install vianu-fraudcrawler
.env
fileMake sure to create an .env
file with the necessary API keys and credentials (c.f. .env.example
file).
python -m fraudcrawler.launch_demo_pipeline
Start by initializing the client
from fraudcrawler import FraudCrawlerClient
# Initialize the client
client = FraudCrawlerClient()
For setting up the search we need 5 main objects.
search_term: str
The search term for the query (similar to search terms used within major search providers).
language: Language
The language used in SerpAPI ('hl' parameter), as well as for the optional search term enrichement (e.g. finding similar and related search terms). language=Language('German')
creates an object having a language name and a language code as: Language(name='German', code='de')
.
location: Location
The location used in SerpAPI ('gl' parameter). location=Location('Switzerland')
creates an object having a location name and a location code as Location(name='Switzerland', code='ch')
.
deepness: Deepness
Defines the search depth with the number of results to retrieve and optional enrichment parameters.
prompts: List[Prompt]
The list of prompts to classify a given product with (multiple) LLM calls. Each prompt object has a name
, a context
(used for defining the user prompt), a system_prompt
(for defining the classification task), allowed_classes
(a list of possible classes) and optionally default_if_missing
(a default class if anything goes wrong).
from fraudcrawler import Language, Location, Deepness, Prompt
# Setup the search
search_term = "sildenafil"
language = Language(name="German")
location = Location(name="Switzerland")
deepness = Deepness(num_results=50)
prompts = [
Prompt(
name="relevance",
context="This organization is interested in medical products and drugs.",
system_prompt=(
"You are a helpful and intelligent assistant. Your task is to classify any given product "
"as either relevant (1) or not relevant (0), strictly based on the context and product details provided by the user. "
"You must consider all aspects of the given context and make a binary decision accordingly. "
"If the product aligns with the user's needs, classify it as 1 (relevant); otherwise, classify it as 0 (not relevant). "
"Respond only with the number 1 or 0."
),
allowed_classes=[0, 1],
)
]
(Optional) Add search term enrichement. This will find related search terms (in a given language) and search for these as well.
from fraudcrawler import Enrichment
deepness.enrichment = Enrichment(
additional_terms=5,
additional_urls_per_term=10
)
(Optional) Add marketplaces where we explicitely want to look for (this will focus your search as the :site parameter for a google search)
from fraudcrawler import Host
marketplaces = [
Host(name="International", domains="zavamed.com,apomeds.com"),
Host(name="National", domains="netdoktor.ch, nobelpharma.ch"),
]
(Optional) Exclude urls (where you don't want to find products)
excluded_urls = [
Host(name="Compendium", domains="compendium.ch"),
]
And finally run the pipeline
# Execute the pipeline
client.execute(
search_term=search_term,
language=language,
location=location,
deepness=deepness,
prompts=prompts,
# marketplaces=marketplaces, # Uncomment this for using marketplaces
# excluded_urls=excluded_urls # Uncomment this for using excluded_urls
)
This creates a file with name pattern <search_term>_<language.code>_<location.code>_<datetime[%Y%m%d%H%M%S]>.csv
inside the folder data/results/
.
Once the pipeline terminated the results can be loaded and examined as follows:
df = client.load_results()
print(df.head(n=10))
If the client has been used to run multiple pipelines, an overview of the available results (for a given instance of
FraudCrawlerClient
) can be obtained with
client.print_available_results()
see CONTRIBUTING.md
The following image provides a schematic representation of the package's async setup.
FAQs
Intelligent Market Monitoring
We found that vianu-fraudcrawler demonstrated a healthy version release cadence and project activity because the last version was released less than a year ago. It has 2 open source maintainers collaborating on the project.
Did you know?
Socket for GitHub automatically highlights issues in each pull request and monitors the health of all your open source dependencies. Discover the contents of your packages and block harmful activity before you install or update your dependencies.
Security News
Django has updated its security policies to reject AI-generated vulnerability reports that include fabricated or unverifiable content.
Security News
ECMAScript 2025 introduces Iterator Helpers, Set methods, JSON modules, and more in its latest spec update approved by Ecma in June 2025.
Security News
A new Node.js homepage button linking to paid support for EOL versions has sparked a heated discussion among contributors and the wider community.