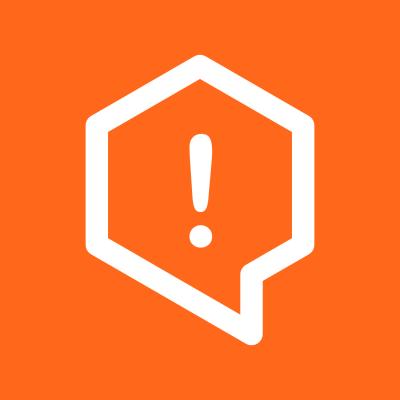
Security News
Fluent Assertions Faces Backlash After Abandoning Open Source Licensing
Fluent Assertions is facing backlash after dropping the Apache license for a commercial model, leaving users blindsided and questioning contributor rights.
.. image:: https://github.com/di/vladiate/actions/workflows/test.yml/badge.svg?query=branch%3Amaster+event%3Apush :target: https://github.com/di/vladiate/actions/workflows/test.yml?query=branch%3Amaster+event%3Apush
.. image:: https://coveralls.io/repos/di/vladiate/badge.svg?branch=master :target: https://coveralls.io/github/di/vladiate
Vladiate helps you write explicit assertions for every field of your CSV file.
Write validation schemas in plain-old Python No UI, no XML, no JSON, just code.
Write your own validators Vladiate comes with a few by default, but there's no reason you can't write your own.
Validate multiple files at once Either with the same schema, or different ones.
Installation
Installing:
::
$ pip install vladiate
Quickstart
~~~~~~~~~~
Below is an example of a ``vladfile.py``
.. code:: python
from vladiate import Vlad
from vladiate.validators import UniqueValidator, SetValidator
from vladiate.inputs import LocalFile
class YourFirstValidator(Vlad):
source = LocalFile('vampires.csv')
validators = {
'Column A': [
UniqueValidator()
],
'Column B': [
SetValidator(['Vampire', 'Not A Vampire'])
]
}
Here we define a number of validators for a local file ``vampires.csv``,
which would look like this:
::
Column A,Column B
Vlad the Impaler,Not A Vampire
Dracula,Vampire
Count Chocula,Vampire
We then run ``vladiate`` in the same directory as your ``.csv`` file:
::
$ vladiate
And get the following output:
::
Validating YourFirstValidator(source=LocalFile('vampires.csv'))
Passed! :)
Handling Changes
^^^^^^^^^^^^^^^^
Let's imagine that you've gotten a new CSV file,
``potential_vampires.csv``, that looks like this:
::
Column A,Column B
Vlad the Impaler,Not A Vampire
Dracula,Vampire
Count Chocula,Vampire
Ronald Reagan,Maybe A Vampire
If we were to update our first validator to use this file as follows:
::
- class YourFirstValidator(Vlad):
- source = LocalFile('vampires.csv')
+ class YourFirstFailingValidator(Vlad):
+ source = LocalFile('potential_vampires.csv')
we would get the following error:
::
Validating YourFirstFailingValidator(source=LocalFile('potential_vampires.csv'))
Failed :(
SetValidator failed 1 time(s) (25.0%) on field: 'Column B'
Invalid fields: ['Maybe A Vampire']
And we would know that we'd either need to sanitize this field, or add
it to the ``SetValidator``.
Starting from scratch
^^^^^^^^^^^^^^^^^^^^^
To make writing a new ``vladfile.py`` easy, Vladiate will give
meaningful error messages.
Given the following as ``real_vampires.csv``:
::
Column A,Column B,Column C
Vlad the Impaler,Not A Vampire
Dracula,Vampire
Count Chocula,Vampire
Ronald Reagan,Maybe A Vampire
We could write a bare-bones validator as follows:
.. code:: python
class YourFirstEmptyValidator(Vlad):
source = LocalFile('real_vampires.csv')
validators = {}
Running this with ``vladiate`` would give the following error:
::
Validating YourFirstEmptyValidator(source=LocalFile('real_vampires.csv'))
Missing...
Missing validators for:
'Column A': [],
'Column B': [],
'Column C': [],
Vladiate expects something to be specified for every column, *even if it
is an empty list* (more on this later). We can easily copy and paste
from the error into our ``vladfile.py`` to make it:
.. code:: python
class YourFirstEmptyValidator(Vlad):
source = LocalFile('real_vampires.csv')
validators = {
'Column A': [],
'Column B': [],
'Column C': [],
}
When we run *this* with ``vladiate``, we get:
::
Validating YourSecondEmptyValidator(source=LocalFile('real_vampires.csv'))
Failed :(
EmptyValidator failed 4 time(s) (100.0%) on field: 'Column A'
Invalid fields: ['Dracula', 'Vlad the Impaler', 'Count Chocula', 'Ronald Reagan']
EmptyValidator failed 4 time(s) (100.0%) on field: 'Column B'
Invalid fields: ['Maybe A Vampire', 'Not A Vampire', 'Vampire']
EmptyValidator failed 4 time(s) (100.0%) on field: 'Column C'
Invalid fields: ['Real', 'Not Real']
This is because Vladiate interprets an empty list of validators for a
field as an ``EmptyValidator``, which expects an empty string in every
field. This helps us make meaningful decisions when adding validators to
our ``vladfile.py``. It also ensures that we are not forgetting about a
column or field which is not empty.
Built-in Validators
^^^^^^^^^^^^^^^^^^^
Vladiate comes with a few common validators built-in:
*class* ``Validator``
Generic validator. Should be subclassed by any custom validators. Not to
be used directly.
*class* ``CastValidator``
Generic "can-be-cast-to-x" validator. Should be subclassed by any
cast-test validator. Not to be used directly.
*class* ``IntValidator``
Validates whether a field can be cast to an ``int`` type or not.
:``empty_ok=False``:
Specify whether a field which is an empty string should be ignored.
*class* ``FloatValidator``
Validates whether a field can be cast to an ``float`` type or not.
:``empty_ok=False``:
Specify whether a field which is an empty string should be ignored.
*class* ``SetValidator``
Validates whether a field is in the specified set of possible fields.
:``valid_set=[]``:
List of valid possible fields
:``empty_ok=False``:
Implicity adds the empty string to the specified set.
:``ignore_case=False``:
Ignore the case between values in the column and valid set
*class* ``UniqueValidator``
Ensures that a given field is not repeated in any other column. Can
optionally determine "uniqueness" with other fields in the row as well via
``unique_with``.
:``unique_with=[]``:
List of field names to make the primary field unique with.
:``empty_ok=False``:
Specify whether a field which is an empty string should be ignored.
*class* ``RegexValidator``
Validates whether a field matches the given regex using `re.match()`.
:``pattern=r'di^'``:
The regex pattern. Fails for all fields by default.
:``full=False``:
Specify whether we should use a fullmatch() or match().
:``empty_ok=False``:
Specify whether a field which is an empty string should be ignored.
*class* ``RangeValidator``
Validates whether a field falls within a given range (inclusive). Can handle
integers or floats.
:``low``:
The low value of the range.
:``high``:
The high value of the range.
:``empty_ok=False``:
Specify whether a field which is an empty string should be ignored.
*class* ``EmptyValidator``
Ensure that a field is always empty. Essentially the same as an empty
``SetValidator``. This is used by default when a field has no
validators.
*class* ``NotEmptyValidator``
The opposite of an ``EmptyValidator``. Ensure that a field is never empty.
*class* ``Ignore``
Always passes validation. Used to explicity ignore a given column.
*class* ``RowValidator``
Generic row validator. Should be subclassed by any custom validators. Not
to be used directly.
*class* ``RowLengthValidator``
Validates that each row has the expected number of fields. The expected
number of fields is inferred from the CSV header row read by
``csv.DictReader``.
Built-in Input Types
^^^^^^^^^^^^^^^^^^^^
Vladiate comes with the following input types:
*class* ``VladInput``
Generic input. Should be subclassed by any custom inputs. Not to be used
directly.
*class* ``LocalFile``
Read from a file local to the filesystem.
:``filename``:
Path to a local CSV file.
*class* ``S3File``
Read from a file in S3. Optionally can specify either a full path, or a
bucket/key pair.
Requires the `boto <https://github.com/boto/boto>`_ library, which should be
installed via ``pip install vladiate[s3]``.
:``path=None``:
A full S3 filepath (e.g., ``s3://foo.bar/path/to/file.csv``)
:``bucket=None``:
S3 bucket. Must be specified with a ``key``.
:``key=None``:
S3 key. Must be specified with a ``bucket``.
*class* ``String``
Read CSV from a string. Can take either an ``str`` or a ``StringIO``.
:``string_input=None``
Regular Python string input.
:``string_io=None``
``StringIO`` input.
Running Vlads Programatically
^^^^^^^^^^^^^^^^^^^^^^^^^^^^^
*class* ``Vlad``
Initialize a Vlad programatically
:``source``:
Required. Any `VladInput`.
:``validators={}``:
List of validators. Optional, defaults to the class variable `validators`
if set, otherwise uses `EmptyValidator` for all fields.
:``delimiter=','``:
The delimiter used within your csv source. Optional, defaults to `,`.
:``ignore_missing_validators=False``:
Whether to fail validation if there are fields in the file for which the
`Vlad` does not have validators. Optional, defaults to `False`.
:``quiet=False``:
Whether to disable log output generated by validations.
Optional, defaults to `False`.
:``file_validation_failure_threshold=None``:
Stops validating the file after this failure threshold is reached.
Input a value between `0.0` and `1.0`. `1.0`(100%) validates the entire file.
Optional, defaults to `None`.
For example:
.. code:: python
from vladiate import Vlad
from vladiate.inputs import LocalFile
Vlad(source=LocalFile('path/to/local/file.csv')).validate()
Testing
~~~~~~~
To run the tests:
::
make test
To run the linter:
::
make lint
Command Line Arguments
.. code:: bash
Usage: vladiate [options] [VladClass [VladClass2 ... ]]
Options:
-h, --help show this help message and exit
-f VLADFILE, --vladfile=VLADFILE
Python module file to import, e.g. '../other.py'.
Default: vladfile
-l, --list Show list of possible vladiate classes and exit
-V, --version show version number and exit
-p PROCESSES, --processes=PROCESSES
attempt to use this number of processes, Default: 1
-q, --quiet disable console log output generated by validations
Dustin Ingram <https://github.com/di>
__Clara Bennett <https://github.com/csojinb>
__Aditya Natraj <https://github.com/adityanatra>
__Sterling Petersen <https://github.com/sterlingpetersen>
__Aleix <https://github.com/maleix>
__Bob Lannon <https://github.com/boblannon>
__Santi <https://github.com/santilytics>
__David Park <https://github.com/dp247>
__Jon Bonafato <https://github.com/jonafato>
__haritha-ravi <https://github.com/haritha-ravi>
__Open source MIT license.
FAQs
Vladiate is a strict validation tool for CSV files
We found that vladiate demonstrated a healthy version release cadence and project activity because the last version was released less than a year ago. It has 2 open source maintainers collaborating on the project.
Did you know?
Socket for GitHub automatically highlights issues in each pull request and monitors the health of all your open source dependencies. Discover the contents of your packages and block harmful activity before you install or update your dependencies.
Security News
Fluent Assertions is facing backlash after dropping the Apache license for a commercial model, leaving users blindsided and questioning contributor rights.
Research
Security News
Socket researchers uncover the risks of a malicious Python package targeting Discord developers.
Security News
The UK is proposing a bold ban on ransomware payments by public entities to disrupt cybercrime, protect critical services, and lead global cybersecurity efforts.