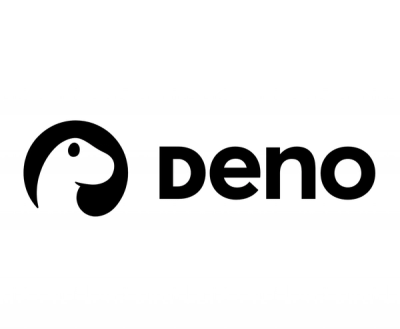
Security News
Deno 2.2 Improves Dependency Management and Expands Node.js Compatibility
Deno 2.2 enhances Node.js compatibility, improves dependency management, adds OpenTelemetry support, and expands linting and task automation for developers.
Install yolov5 using pip (for Python >=3.7)
pip install yolov5
Effortlessly explore and use finetuned YOLOv5 models with one line of code: awesome-yolov5-models
import yolov5
# load pretrained model
model = yolov5.load('yolov5s.pt')
# or load custom model
model = yolov5.load('train/best.pt')
# set model parameters
model.conf = 0.25 # NMS confidence threshold
model.iou = 0.45 # NMS IoU threshold
model.agnostic = False # NMS class-agnostic
model.multi_label = False # NMS multiple labels per box
model.max_det = 1000 # maximum number of detections per image
# set image
img = 'https://github.com/ultralytics/yolov5/raw/master/data/images/zidane.jpg'
# perform inference
results = model(img)
# inference with larger input size
results = model(img, size=1280)
# inference with test time augmentation
results = model(img, augment=True)
# parse results
predictions = results.pred[0]
boxes = predictions[:, :4] # x1, y1, x2, y2
scores = predictions[:, 4]
categories = predictions[:, 5]
# show detection bounding boxes on image
results.show()
# save results into "results/" folder
results.save(save_dir='results/')
from yolov5 import train, val, detect, export
# from yolov5.classify import train, val, predict
# from yolov5.segment import train, val, predict
train.run(imgsz=640, data='coco128.yaml')
val.run(imgsz=640, data='coco128.yaml', weights='yolov5s.pt')
detect.run(imgsz=640)
export.run(imgsz=640, weights='yolov5s.pt')
from yolov5 import detect
img_url = 'https://github.com/ultralytics/yolov5/raw/master/data/images/zidane.jpg'
detect.run(source=img_url, weights="yolov5s6.pt", conf_thres=0.25, imgsz=640)
You can call yolov5 train
, yolov5 detect
, yolov5 val
and yolov5 export
commands after installing the package via pip
:
data.yaml
:$ yolov5 train --data data.yaml --weights yolov5s.pt --batch-size 16 --img 640
yolov5m.pt 8
yolov5l.pt 4
yolov5x.pt 2
# data.yml
train_json_path: "train.json"
train_image_dir: "train_image_dir/"
val_json_path: "val.json"
val_image_dir: "val_image_dir/"
$ yolov5 train --data data.yaml --weights yolov5s.pt
$ yolov5 train --data DATASET_UNIVERSE_URL --weights yolov5s.pt --roboflow_token YOUR_ROBOFLOW_TOKEN
Where DATASET_UNIVERSE_URL
must be in https://universe.roboflow.com/workspace_name/project_name/project_version
format.
$ yolov5 train --data data.yaml --weights yolov5s.pt --neptune_project NAMESPACE/PROJECT_NAME --neptune_token YOUR_NEPTUNE_TOKEN
$ yolov5 train --data data.yaml --weights yolov5s.pt --hf_model_id username/modelname --hf_token YOUR-HF-WRITE-TOKEN
export AWS_ACCESS_KEY_ID=YOUR_KEY
export AWS_SECRET_ACCESS_KEY=YOUR_KEY
$ yolov5 train --data data.yaml --weights yolov5s.pt --s3_upload_dir YOUR_S3_FOLDER_DIRECTORY --upload_dataset
yolo_s3_data_dir
into data.yaml
to match Neptune dataset with a present dataset in S3.# data.yml
train_json_path: "train.json"
train_image_dir: "train_image_dir/"
val_json_path: "val.json"
val_image_dir: "val_image_dir/"
yolo_s3_data_dir: s3://bucket_name/data_dir/
yolov5 detect command runs inference on a variety of sources, downloading models automatically from the latest YOLOv5 release and saving results to runs/detect
.
$ yolov5 detect --source 0 # webcam
file.jpg # image
file.mp4 # video
path/ # directory
path/*.jpg # glob
rtsp://170.93.143.139/rtplive/470011e600ef003a004ee33696235daa # rtsp stream
rtmp://192.168.1.105/live/test # rtmp stream
http://112.50.243.8/PLTV/88888888/224/3221225900/1.m3u8 # http stream
You can export your fine-tuned YOLOv5 weights to any format such as torchscript
, onnx
, coreml
, pb
, tflite
, tfjs
:
$ yolov5 export --weights yolov5s.pt --include torchscript,onnx,coreml,pb,tfjs
Train/Val/Predict with YOLOv5 image classifier:
$ yolov5 classify train --img 640 --data mnist2560 --weights yolov5s-cls.pt --epochs 1
$ yolov5 classify predict --img 640 --weights yolov5s-cls.pt --source images/
Train/Val/Predict with YOLOv5 instance segmentation model:
$ yolov5 segment train --img 640 --weights yolov5s-seg.pt --epochs 1
$ yolov5 segment predict --img 640 --weights yolov5s-seg.pt --source images/
FAQs
Packaged version of the Yolov5 object detector
We found that yolov5 demonstrated a healthy version release cadence and project activity because the last version was released less than a year ago. It has 1 open source maintainer collaborating on the project.
Did you know?
Socket for GitHub automatically highlights issues in each pull request and monitors the health of all your open source dependencies. Discover the contents of your packages and block harmful activity before you install or update your dependencies.
Security News
Deno 2.2 enhances Node.js compatibility, improves dependency management, adds OpenTelemetry support, and expands linting and task automation for developers.
Security News
React's CRA deprecation announcement sparked community criticism over framework recommendations, leading to quick updates acknowledging build tools like Vite as valid alternatives.
Security News
Ransomware payment rates hit an all-time low in 2024 as law enforcement crackdowns, stronger defenses, and shifting policies make attacks riskier and less profitable.