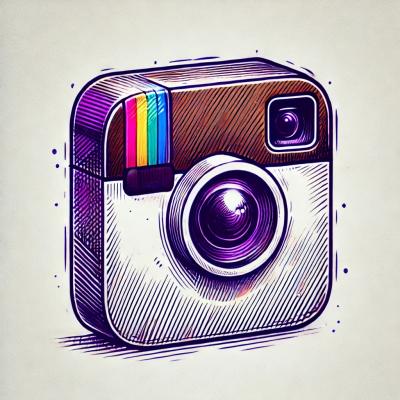
Research
PyPI Package Disguised as Instagram Growth Tool Harvests User Credentials
A deceptive PyPI package posing as an Instagram growth tool collects user credentials and sends them to third-party bot services.
Provided are some toy implementations for some basic computer science problems.
Node
classesNode
A Node provides a tree structure by assigning other Nodes to @children
.
Node
@value
@children
#[]
- child node at index#[]=
- set child node at indexdisplay
- display full tree with all descendentsKeyNode
A KeyNode adds @key
and allows a comparison based search on the key.
Binary search trees
are supported, and the @duplicated
flag determines whether duplicate keys
are allowed to be inserted. Any duplicates will not be returned from
#search
. A Ternary search tree is also supported, and it inherently allows
duplicates keys. #search
returns an array of KeyNode
, possibly empty.
KeyNode < Node
KeyNode.key_cmp_idx
- compare 2 keys to decide on a child slot@key
- any Comparable@duplicates
- boolean flag relevant for @children.size == 2#cidx
- calls KeyNode.key_cmp_idx
#insert
#search
ChildNode
A ChildNode adds reference to its @parent
.
ChildNode < Node
@parent
#gen
#siblings
CompleteTree
classesEfficient Array implementation of a complete tree uses arithmetic to determine parent/child relationships.
CompleteTree
CompleteTree.parent_idx
CompleteTree.children_idx
CompleteTree.gen
@array
@child_slots
#push
#pop
#size
#last_idx
#display
- alias #to_s
CompleteBinaryTree < CompleteTree
@child_slots = 2
CompleteTernaryTree < CompleteTree
@child_slots = 3
CompleteQuaternaryTree < CompleteTree
@child_slots = 4
Heap
classCompleteTree implementation. Both minheaps and maxheaps are
supported. Any number of children may be provided via child_slots
.
The primary operations are Heap#push
and Heap#pop
. My basic Vagrant VM
gets over 500k pushes per second, constant up past
1M pushes.
Heap < CompleteTree
#push
#pop
#sift_up
#sift_down
Fibonacci
moduleFibonacci.classic(n)
- naive, recursive
Fibonacci.cache_recursive(n)
- as above, caching already computed results
Fibonacci.cache_iterative(n)
- as above but iterative
Fibonacci.dynamic(n)
- as above but without a cache structure
Fibonacci.matrix(n)
- matrix is magic; beats dynamic around n=500
Timer
moduleTimer.now
- uses Process::CLOCK_MONOTONIC
if available
Timer.since
- provides the elapsed time since a prior time
Timer.elapsed
- provides the elapsed time to run a block
Timer.loop_avg
- loops a block; returns final value and mean elapsed time
require 'compsci/timer'
include CompSci
overall_start = Timer.now
start = Timer.now
print "running sleep 0.01 (50x): "
_answer, each_et = Timer.loop_avg(count: 50) {
print '.'
sleep 0.01
}
puts
puts "each: %0.3f" % each_et
puts "elapsed: %0.3f" % Timer.since(start)
puts "cumulative: %0.3f" % Timer.since(overall_start)
puts
start = Timer.now
print "running sleep 0.02 (0.3 s): "
_answer, each_et = Timer.loop_avg(seconds: 0.3) {
print '.'
sleep 0.02
}
puts
puts "each: %0.3f" % each_et
puts "elapsed: %0.3f" % Timer.since(start)
puts "cumulative: %0.3f" % Timer.since(overall_start)
puts
running sleep 0.01 (50x): ..................................................
each: 0.010
elapsed: 0.524
cumulative: 0.524
running sleep 0.02 (0.3 s): ...............
each: 0.020
elapsed: 0.304
cumulative: 0.828
Fit
moduleFit.sigma
- sums the result of a block applied to array values
Fit.error
- returns a generic r^2 value, the coefficient of determination
Fit.constant
- fits y = a + 0x
; returns the mean and variance
Fit.logarithmic
- fits y = a + b*ln(x)
; returns a, b, r^2
Fit.linear
- fits y = a + bx
; returns a, b, r^2
Fit.exponential
- fits y = ae^(bx)
; returns a, b, r^2
Fit.power
- fits y = ax^b
; returns a, b, r^2
Fit.best
- applies known fits; returns the fit with highest r^2
Names
moduleThis helps map a range of small integers to friendly names, typically in alphabetical order.
ENGLISH_UPPER
ENGLISH_LOWER
WW1
WW2
NATO
CRYPTO
PLANETS
SOLAR
Names.assign
Names::Greek
moduleUPPER
LOWER
SYMBOLS
CHAR_MAP
LATIN_SYMBOLS
SYMBOLS26
Names::Greek.upper
Names::Greek.lower
Names::Greek.sym
Names::Pokemon
moduleNames::Pokemon.array
Names::Pokemon.hash
Names::Pokemon.grep
Names::Pokemon.sample
Simplex
classThe Simplex algorithm is a technique for Linear programming. Typically the problem is to maximize some linear expression of variables given some constraints on those variables in terms of linear inequalities.
Simplex::Parse
moduleParse.tokenize
- convert a string to an array of tokens
Parse.term
- parse certain tokens into [coefficient, varname]
Parse.expression
- parse a string representing a sum of terms
Parse.inequality
- parse a string like "#{expression} <= #{const}"
With Simplex::Parse
, one can obtain solutions via:
Simplex.maximize
- takes an expression to maximize followed by a variable
number of constraints / inequalities; returns a solutionSimplex.problem
- a more general form of Simplex.maximize
; returns a
Simplex objectrequire 'compsci/simplex/parse'
include CompSci
Simplex.maximize('x + y',
'2x + y <= 4',
'x + 2y <= 3')
# => [1.6666666666666667, 0.6666666666666666]
s = Simplex.problem(maximize: 'x + y',
constraints: ['2x + y <= 4',
'x + 2y <= 3'])
# => #<CompSci::Simplex:0x0055b2deadbeef
# @max_pivots=10000,
# @num_non_slack_vars=2,
# @num_constraints=2,
# @num_vars=4,
# @c=[-1.0, -1.0, 0, 0],
# @a=[[2.0, 1.0, 1, 0], [1.0, 2.0, 0, 1]],
# @b=[4.0, 3.0],
# @basic_vars=[2, 3],
# @x=[0, 0, 4.0, 3.0]>
s.solution
# => [1.6666666666666667, 0.6666666666666666]
FAQs
Unknown package
We found that compsci demonstrated a not healthy version release cadence and project activity because the last version was released a year ago. It has 1 open source maintainer collaborating on the project.
Did you know?
Socket for GitHub automatically highlights issues in each pull request and monitors the health of all your open source dependencies. Discover the contents of your packages and block harmful activity before you install or update your dependencies.
Research
A deceptive PyPI package posing as an Instagram growth tool collects user credentials and sends them to third-party bot services.
Product
Socket now supports pylock.toml, enabling secure, reproducible Python builds with advanced scanning and full alignment with PEP 751's new standard.
Security News
Research
Socket uncovered two npm packages that register hidden HTTP endpoints to delete all files on command.