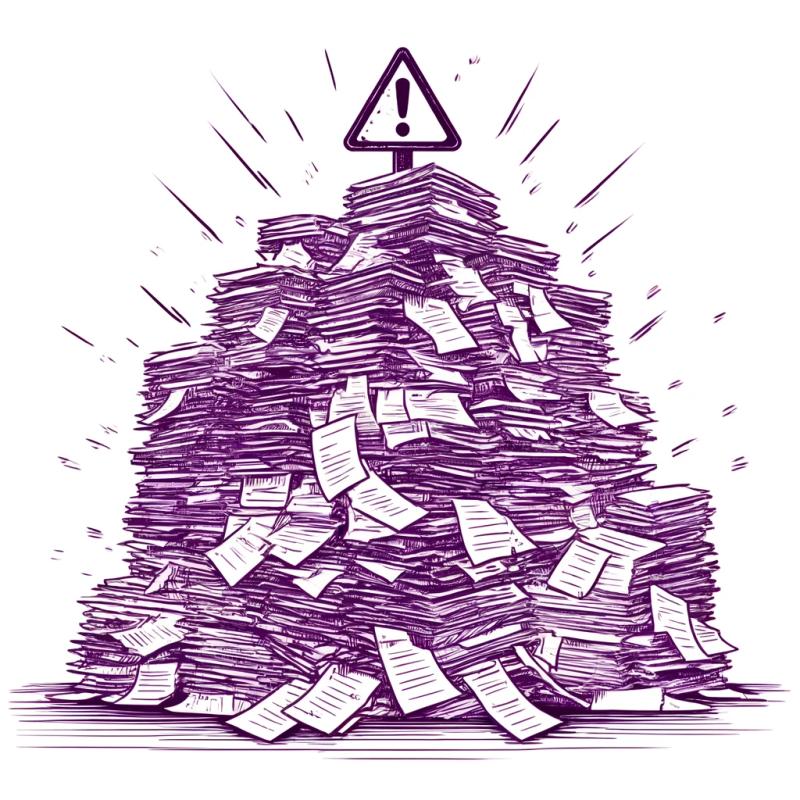
Security News
CISA Launches Vulnrichment Project as NVD Backlog Hits 10,000
CISA launched a new project called Vulnrichment to enrich CVEs with details that help prioritize patching and mitigation efforts, as the NVD backlog of unenriched CVEs awaiting analysis surpasses 10,000.