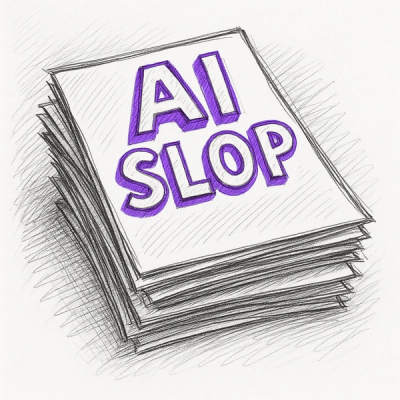
Security News
Django Joins curl in Pushing Back on AI Slop Security Reports
Django has updated its security policies to reject AI-generated vulnerability reports that include fabricated or unverifiable content.
Automatic Evaluation Metric described in the paper BERTScore: Evaluating Text Generation with BERT (ICLR 2020). We now support about 130 models (see this spreadsheet for their correlations with human evaluation). Currently, the best model is microsoft/deberta-xlarge-mnli
, please consider using it instead of the default roberta-large
in order to have the best correlation with human evaluation.
Updated to version 0.3.13
Updated to version 0.3.12
Updated to version 0.3.11
Updated to version 0.3.10
--use_fast_tokenizer
. Notably, you will get different scores because of the difference in the tokenizer implementations (#106).Updated to version 0.3.9
Updated to version 0.3.8
--model_type microsoft/deberta-xlarge-mnli
or --model_type microsoft/deberta-large-mnli
(faster) if you want the scores to correlate better with human scores.Updated to version 0.3.7
See #22 if you want to replicate our experiments on the COCO Captioning dataset.
For people in China, downloading pre-trained weights can be very slow. We provide copies of a few models on Baidu Pan.
Huggingface's datasets library includes BERTScore in their metric collection.
--rescale-with-baseline
is changed to --rescale_with_baseline
so that it is consistent with other options.BERTScorer
object that caches the model to avoid re-loading it multiple times. Please see our jupyter notebook example for the usage.score
function now can take a list of lists of strings as the references and return the score between the candidate sentence and its closest reference sentence.Please see release logs for older updates.
*: Equal Contribution
BERTScore leverages the pre-trained contextual embeddings from BERT and matches words in candidate and reference sentences by cosine similarity. It has been shown to correlate with human judgment on sentence-level and system-level evaluation. Moreover, BERTScore computes precision, recall, and F1 measure, which can be useful for evaluating different language generation tasks.
For an illustration, BERTScore recall can be computed as
If you find this repo useful, please cite:
@inproceedings{bert-score,
title={BERTScore: Evaluating Text Generation with BERT},
author={Tianyi Zhang* and Varsha Kishore* and Felix Wu* and Kilian Q. Weinberger and Yoav Artzi},
booktitle={International Conference on Learning Representations},
year={2020},
url={https://openreview.net/forum?id=SkeHuCVFDr}
}
Install from pypi with pip by
pip install bert-score
Install latest unstable version from the master branch on Github by:
pip install git+https://github.com/Tiiiger/bert_score
Install it from the source by:
git clone https://github.com/Tiiiger/bert_score
cd bert_score
pip install .
and you may test your installation by:
python -m unittest discover
On a high level, we provide a python function bert_score.score
and a python object bert_score.BERTScorer
.
The function provides all the supported features while the scorer object caches the BERT model to faciliate multiple evaluations.
Check our demo to see how to use these two interfaces.
Please refer to bert_score/score.py
for implementation details.
Running BERTScore can be computationally intensive (because it uses BERT :p). Therefore, a GPU is usually necessary. If you don't have access to a GPU, you can try our demo on Google Colab
We provide a command line interface (CLI) of BERTScore as well as a python module. For the CLI, you can use it as follows:
We provide example inputs under ./example
.
bert-score -r example/refs.txt -c example/hyps.txt --lang en
You will get the following output at the end:
roberta-large_L17_no-idf_version=0.3.0(hug_trans=2.3.0) P: 0.957378 R: 0.961325 F1: 0.959333
where "roberta-large_L17_no-idf_version=0.3.0(hug_trans=2.3.0)" is the hash code.
Starting from version 0.3.0, we support rescaling the scores with baseline scores
bert-score -r example/refs.txt -c example/hyps.txt --lang en --rescale_with_baseline
You will get:
roberta-large_L17_no-idf_version=0.3.0(hug_trans=2.3.0)-rescaled P: 0.747044 R: 0.770484 F1: 0.759045
This makes the range of the scores larger and more human-readable. Please see this post for details.
When having multiple reference sentences, please use
bert-score -r example/refs.txt example/refs2.txt -c example/hyps.txt --lang en
where the -r
argument supports an arbitrary number of reference files. Each reference file should have the same number of lines as your candidate/hypothesis file. The i-th line in each reference file corresponds to the i-th line in the candidate file.
We currently support the 104 languages in multilingual BERT (full list).
Please specify the two-letter abbreviation of the language. For instance, using --lang zh
for Chinese text.
See more options by bert-score -h
.
--model
and --num_layers
.bert-score -r example/refs.txt -c example/hyps.txt --model path_to_my_bert --num_layers 9
bert-score-show --lang en -r "There are two bananas on the table." -c "On the table are two apples." -f out.png
The figure will be saved to out.png.
Some weights of the model checkpoint at roberta-large were not used when initializing RobertaModel: ['lm_head.decoder.weight', 'lm_head.layer_norm.weight', 'lm_head.dense.bias', 'lm_head.layer_norm.bias', 'lm_head.bias', 'lm_head.dense.weight']
- This IS expected if you are initializing RobertaModel from the checkpoint of a model trained on another task or with another architecture (e.g. initializing a BertForSequenceClassification model from a BertForPreTraining model).
- This IS NOT expected if you are initializing RobertaModel from the checkpoint of a model that you expect to be exactly identical (initializing a BertForSequenceClassification model from a BertForSequenceClassification model).
roberta-large_L17_no-idf_version=0.3.0(hug_trans=2.3.0)-rescaled
) in your paper so that people know what setting you use. This is inspired by sacreBLEU. Changes in huggingface's transformers version may also affect the score (See issue #46).sent = re.sub(r' +', ' ', sent)
or sent = re.sub(r'\s+', ' ', sent)
.--idf
when using the CLI tool or
idf=True
when calling bert_score.score
function.batch_size
when calling
bert_score.score
function.-m MODEL_TYPE
when using the CLI tool
or model_type=MODEL_TYPE
when calling bert_score.score
function.-l LAYER
or num_layers=LAYER
. To tune the best layer for your custom model, please follow the instructions in tune_layers folder.Language | Model |
---|---|
en | roberta-large |
en-sci | allenai/scibert_scivocab_uncased |
zh | bert-base-chinese |
tr | dbmdz/bert-base-turkish-cased |
others | bert-base-multilingual-cased |
Please see this Google sheet for the supported models and their performance.
This repo wouldn't be possible without the awesome bert, fairseq, and transformers.
FAQs
PyTorch implementation of BERT score
We found that bert-score demonstrated a healthy version release cadence and project activity because the last version was released less than a year ago. It has 2 open source maintainers collaborating on the project.
Did you know?
Socket for GitHub automatically highlights issues in each pull request and monitors the health of all your open source dependencies. Discover the contents of your packages and block harmful activity before you install or update your dependencies.
Security News
Django has updated its security policies to reject AI-generated vulnerability reports that include fabricated or unverifiable content.
Security News
ECMAScript 2025 introduces Iterator Helpers, Set methods, JSON modules, and more in its latest spec update approved by Ecma in June 2025.
Security News
A new Node.js homepage button linking to paid support for EOL versions has sparked a heated discussion among contributors and the wider community.