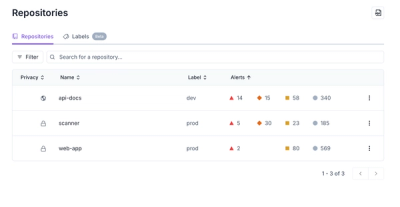
Product
Redesigned Repositories Page: A Faster Way to Prioritize Security Risk
Our redesigned Repositories page adds alert severity, filtering, and tabs for faster triage and clearer insights across all your projects.
A pure python bloom filter (low storage requirement, probabilistic set datastructure) is provided. It is known to work on CPython 3.x, Pypy, and Jython.
Includes mmap, in-memory and disk-seek backends.
This project builds on drs-bloom-filter
and bloom_filter_mod
.
Credits and links can be found in AUTHORS.md.
The user specifies the desired maximum number of elements and the desired maximum false positive probability, and the module calculates the rest.
::
from bloom_filter2 import BloomFilter
# instantiate BloomFilter with custom settings,
# max_elements is how many elements you expect the filter to hold.
# error_rate defines accuracy; You can use defaults with
# `BloomFilter()` without any arguments. Following example
# is same as defaults:
bloom = BloomFilter(max_elements=10000, error_rate=0.1)
# Test whether the bloom-filter has seen a key:
assert "test-key" not in bloom
# Mark the key as seen
bloom.add("test-key")
# Now check again
assert "test-key" in bloom
FAQs
Pure Python Bloom Filter module
We found that bloom-filter2 demonstrated a healthy version release cadence and project activity because the last version was released less than a year ago. It has 1 open source maintainer collaborating on the project.
Did you know?
Socket for GitHub automatically highlights issues in each pull request and monitors the health of all your open source dependencies. Discover the contents of your packages and block harmful activity before you install or update your dependencies.
Product
Our redesigned Repositories page adds alert severity, filtering, and tabs for faster triage and clearer insights across all your projects.
Security News
Slopsquatting is a new supply chain threat where AI-assisted code generators recommend hallucinated packages that attackers register and weaponize.
Security News
Multiple deserialization flaws in PyTorch Lightning could allow remote code execution when loading untrusted model files, affecting versions up to 2.4.0.