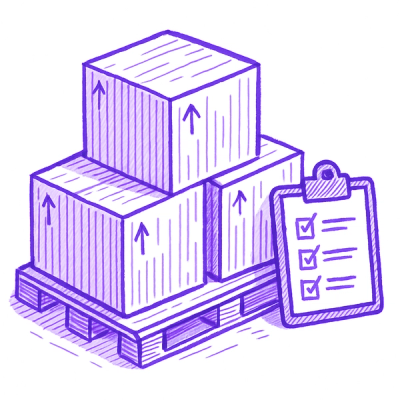
Security News
Open Source Maintainers Feeling the Weight of the EU’s Cyber Resilience Act
The EU Cyber Resilience Act is prompting compliance requests that open source maintainers may not be obligated or equipped to handle.
This is a python based camera calibration "library". Some things:
nn.module
in the modules.ipynb
notebook.
LBFGS
optimizer. The LBFGS
optimizer uses only the gradient to do a quasi second order optimization. However, I've noticed it's imperfect and can a take long time to converge in some cases.import camera_calib.api as api
Before calibration can be done, we need the following information:
import re
from pathlib import Path
files_img = list(Path('data/dot_vision_checker').glob('*.png'))
files_img
[PosixPath('data/dot_vision_checker/SERIAL_16276941_DATETIME_2019-06-07-00:38:48-109732_CAM_2_FRAMEID_0_COUNTER_2.png'),
PosixPath('data/dot_vision_checker/SERIAL_19061245_DATETIME_2019-06-07-00:38:19-438594_CAM_1_FRAMEID_0_COUNTER_1.png'),
PosixPath('data/dot_vision_checker/SERIAL_16276942_DATETIME_2019-06-07-00:38:19-438636_CAM_3_FRAMEID_0_COUNTER_1.png'),
PosixPath('data/dot_vision_checker/SERIAL_16276942_DATETIME_2019-06-07-00:38:48-109736_CAM_3_FRAMEID_0_COUNTER_2.png'),
PosixPath('data/dot_vision_checker/SERIAL_16276941_DATETIME_2019-06-07-00:38:19-438631_CAM_2_FRAMEID_0_COUNTER_1.png')]
def _parse_name(name_img):
match = re.match(r'''SERIAL_(?P<serial>.*)_
DATETIME_(?P<date>.*)_
CAM_(?P<cam>.*)_
FRAMEID_(?P<frameid>.*)_
COUNTER_(?P<counter>.*).png''',
name_img,
re.VERBOSE)
return match.groupdict()
imgs = []
for file_img in files_img:
dict_group = _parse_name(file_img.name)
img = api.File16bitImg(file_img)
img.idx_cam = int(dict_group['cam'])-1
img.idx_cb = int(dict_group['counter'])-1
imgs.append(img)
for img in imgs: print(f'{img.name} - cam: {img.idx_cam} - cb: {img.idx_cb}')
SERIAL_16276941_DATETIME_2019-06-07-00:38:48-109732_CAM_2_FRAMEID_0_COUNTER_2 - cam: 1 - cb: 1
SERIAL_19061245_DATETIME_2019-06-07-00:38:19-438594_CAM_1_FRAMEID_0_COUNTER_1 - cam: 0 - cb: 0
SERIAL_16276942_DATETIME_2019-06-07-00:38:19-438636_CAM_3_FRAMEID_0_COUNTER_1 - cam: 2 - cb: 0
SERIAL_16276942_DATETIME_2019-06-07-00:38:48-109736_CAM_3_FRAMEID_0_COUNTER_2 - cam: 2 - cb: 1
SERIAL_16276941_DATETIME_2019-06-07-00:38:19-438631_CAM_2_FRAMEID_0_COUNTER_1 - cam: 1 - cb: 0
The calibration board geometry specifies where fiducial markers and control points are located. For this example, my dot vision checker board is used.
h_cb = 50.8
w_cb = 50.8
h_f = 42.672
w_f = 42.672
num_c_h = 16
num_c_w = 16
spacing_c = 2.032
cb_geom = api.CbGeom(h_cb, w_cb,
api.CpCSRGrid(num_c_h, num_c_w, spacing_c),
api.FmCFPGrid(h_f, w_f))
cb_geom.plot()
from pathlib import Path
This fiducial detector will take in an image and return the locations of the fiducial markers. The detector in this example is a neural net trained specifically on my calibration board. More info available at:
file_model = Path('models/dot_vision_checker.pth')
detector = api.DotVisionCheckerDLDetector(file_model)
The refiner will take in an image, initial guesses for control points, and the boundaries around the control points, and return a refined point. The boundaries help determine how much neighboring info can be used to refine the control point.
refiner = api.OpenCVCheckerRefiner(hw_min=5, hw_max=15, cutoff_it=20, cutoff_norm=1e-3)
Now, we can calibrate
calib = api.multi_calib(imgs, cb_geom, detector, refiner)
Refining control points for: SERIAL_19061245_DATETIME_2019-06-07-00:38:19-438594_CAM_1_FRAMEID_0_COUNTER_1...
Refining single parameters...
- Iteration: 000 - Norm: 0.00492 - Loss: 5.36733
- Iteration: 001 - Norm: 0.14985 - Loss: 3.73449
- Iteration: 002 - Norm: 0.01378 - Loss: 3.72178
- Iteration: 003 - Norm: 3.80677 - Loss: 3.50140
- Iteration: 004 - Norm: 60.91136 - Loss: 1.69839
- Iteration: 005 - Norm: 0.00000 - Loss: 1.69839
Refining control points for: SERIAL_16276941_DATETIME_2019-06-07-00:38:48-109732_CAM_2_FRAMEID_0_COUNTER_2...
Refining control points for: SERIAL_16276941_DATETIME_2019-06-07-00:38:19-438631_CAM_2_FRAMEID_0_COUNTER_1...
Refining single parameters...
- Iteration: 000 - Norm: 0.04150 - Loss: 145.18373
- Iteration: 001 - Norm: 0.13431 - Loss: 83.63355
- Iteration: 002 - Norm: 0.84358 - Loss: 3.92886
- Iteration: 003 - Norm: 0.27788 - Loss: 3.59249
- Iteration: 004 - Norm: 27.32694 - Loss: 2.63209
- Iteration: 005 - Norm: 0.01238 - Loss: 2.63208
- Iteration: 006 - Norm: 0.00000 - Loss: 2.63208
Refining control points for: SERIAL_16276942_DATETIME_2019-06-07-00:38:19-438636_CAM_3_FRAMEID_0_COUNTER_1...
Refining control points for: SERIAL_16276942_DATETIME_2019-06-07-00:38:48-109736_CAM_3_FRAMEID_0_COUNTER_2...
Refining single parameters...
- Iteration: 000 - Norm: 0.04606 - Loss: 59.69785
- Iteration: 001 - Norm: 0.18309 - Loss: 23.21653
- Iteration: 002 - Norm: 0.19523 - Loss: 10.38509
- Iteration: 003 - Norm: 0.09765 - Loss: 10.04688
- Iteration: 004 - Norm: 1.24157 - Loss: 9.89971
- Iteration: 005 - Norm: 104.59411 - Loss: 1.76128
- Iteration: 006 - Norm: 0.29888 - Loss: 1.76086
- Iteration: 007 - Norm: 0.00000 - Loss: 1.76086
Refining multi parameters...
- Iteration: 000 - Norm: 0.00057 - Loss: 10.14000
- Iteration: 001 - Norm: 0.00077 - Loss: 8.43795
- Iteration: 002 - Norm: 0.00093 - Loss: 8.04904
- Iteration: 003 - Norm: 0.00117 - Loss: 7.83528
- Iteration: 004 - Norm: 0.00270 - Loss: 7.61741
- Iteration: 005 - Norm: 0.00085 - Loss: 7.56616
- Iteration: 006 - Norm: 0.00390 - Loss: 7.39859
- Iteration: 007 - Norm: 0.00385 - Loss: 7.29511
- Iteration: 008 - Norm: 0.00106 - Loss: 7.28492
- Iteration: 009 - Norm: 0.00278 - Loss: 7.27331
- Iteration: 010 - Norm: 0.00804 - Loss: 7.24146
- Iteration: 011 - Norm: 0.00827 - Loss: 7.21109
- Iteration: 012 - Norm: 0.00414 - Loss: 7.20269
- Iteration: 013 - Norm: 0.00452 - Loss: 7.19479
- Iteration: 014 - Norm: 0.00009 - Loss: 7.19475
- Iteration: 015 - Norm: 0.01420 - Loss: 7.17619
- Iteration: 016 - Norm: 0.00618 - Loss: 7.17040
- Iteration: 017 - Norm: 0.01975 - Loss: 7.15089
- Iteration: 018 - Norm: 0.00002 - Loss: 7.15089
- Iteration: 019 - Norm: 0.00000 - Loss: 7.15089
From Bo Li's calibration paper, we know the coordinate graph of calibration board poses and cameras forms a bipartite graph. For debugging purposes this is displayed below.
api.plot_bipartite(calib)
Plot residuals
api.plot_residuals(calib);
Plot extrinsics; note that %matplotlib notebook
can be used to make the plot interactive
import matplotlib.pyplot as plt
fig = plt.figure(figsize=(20,20))
ax = fig.add_subplot(2, 2, 1, projection='3d')
api.plot_extrinsics(calib, ax=ax)
ax.view_init(elev=90, azim=-90)
ax = fig.add_subplot(2, 2, 2, projection='3d')
api.plot_extrinsics(calib, ax=ax)
ax.view_init(elev=45, azim=-45)
ax = fig.add_subplot(2, 2, 3, projection='3d')
api.plot_extrinsics(calib, ax=ax)
ax.view_init(elev=0, azim=-90)
ax = fig.add_subplot(2, 2, 4, projection='3d')
api.plot_extrinsics(calib, ax=ax)
ax.view_init(elev=0, azim=0)
plt.subplots_adjust(wspace=0, hspace=0)
This matches pretty closely to my camera rig
Save
api.save(calib, '/tmp/calib.pth')
Load
del calib
calib = api.load('/tmp/calib.pth')
from camera_calib.utils import convert_notebook
convert_notebook()
FAQs
Camera Calibration with pytorch
We found that camera-calib demonstrated a healthy version release cadence and project activity because the last version was released less than a year ago. It has 1 open source maintainer collaborating on the project.
Did you know?
Socket for GitHub automatically highlights issues in each pull request and monitors the health of all your open source dependencies. Discover the contents of your packages and block harmful activity before you install or update your dependencies.
Security News
The EU Cyber Resilience Act is prompting compliance requests that open source maintainers may not be obligated or equipped to handle.
Security News
Crates.io adds Trusted Publishing support, enabling secure GitHub Actions-based crate releases without long-lived API tokens.
Research
/Security News
Undocumented protestware found in 28 npm packages disrupts UI for Russian-language users visiting Russian and Belarusian domains.