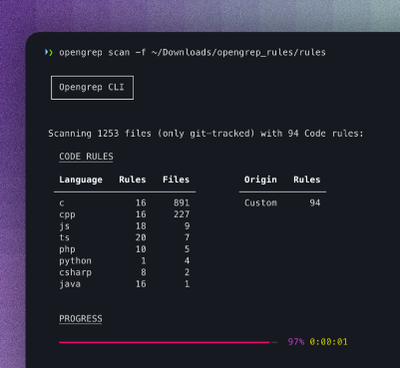
Security News
Opengrep Emerges as Open Source Alternative Amid Semgrep Licensing Controversy
Opengrep forks Semgrep to preserve open source SAST in response to controversial licensing changes.
Claim Processor provides automatic checking pipeline for detecting fine-grained hallucinations generated by Large Language Models.
TODO: Update the new features here
-------------------------------- ORIGINAL PACKAGE ---------------------------------
| 🔥 News | 🤖️ Demo | 🚀 Quick Start | 💾 Benchmark | 📖 Docs |
RefChecker provides a standardized assessment framework to identify subtle hallucinations present in the outputs of large language models (LLMs).
Figure: RefChecker Framework
You can explore RefChecker in the following ways:
Please check out the paper here: https://arxiv.org/pdf/2405.14486
If you use RefChecker in your work, please cite us:
@article{hu2024refchecker,
title={RefChecker: Reference-based Fine-grained Hallucination Checker and Benchmark for Large Language Models},
author={Xiangkun Hu and Dongyu Ru and Lin Qiu and Qipeng Guo and Tianhang Zhang and Yang Xu and Yun Luo and Pengfei Liu and Yue Zhang and Zheng Zhang},
year={2024},
eprint={2405.14486},
archivePrefix={arXiv},
primaryClass={cs.CL}
}
You can first setup a demo website and then use the web UI to try RefChecker as the animation shows above. There are four steps to perform hallucination detection in it:
Next Step
button on the right side. The checker will extract triplets in your text and show them in the bottom-left area.Next Step
button. If you don’t have reference text, leave the box empty and click the button anyway. We will retrieve some references with the text to be checked using search engines.Next Step
button and the checker will perform triplet localization. You can click the button on the left of each triplet to see the localization result.First create a python environment using conda or virtualenv. Clone this repo and change path into the root directory. Then install:
pip install -e .
python -m spacy download en_core_web_sm
Install optional dependencies to use open source extractors (Mistral, Mixtral) or enable acceleration for RepCChecker.
pip install -e .[open-extractor,repcex]
We use litellm as to invoke the LLMs. Please check the document for how to setup the model for different LLM providers: https://docs.litellm.ai/docs/providers . We give some examples below:
import os
from claim_processor import LLMExtractor, LLMChecker
# Setup the enviroment variables if you are not using AWS EC2 instance
# If you are using AWS EC2, make sure your region has the access to the model
os.environ["AWS_ACCESS_KEY_ID"] = "<your_aws_access_key_id>"
os.environ["AWS_SECRET_ACCESS_KEY"] = "<your_aws_secret_access_key>"
os.environ["AWS_REGION_NAME"] = "<your_aws_region_name>"
# Claude 3 Sonnet from Amazon Bedrock
model = 'bedrock/anthropic.claude-3-sonnet-20240229-v1:0'
extractor = LLMExtractor(model=model, batch_size=8)
checker = LLMChecker(model=model, batch_size=8)
You can also setup the enviroment variables in terminal to avoid disclosing these information in the code:
export AWS_ACCESS_KEY_ID=<your_aws_access_key_id>
export AWS_SECRET_ACCESS_KEY=<your_aws_secret_access_key>
export AWS_REGION_NAME=<your_aws_region_name>
import os
from claim_processor import LLMExtractor, LLMChecker
os.environ["OPENAI_API_KEY"] = "<your_openai_api_key>"
# GPT-4o from OpenAI
model = 'gpt-4o'
extractor = LLMExtractor(model=model, batch_size=8)
checker = LLMChecker(model=model, batch_size=8)
Please use vllm to setup the API server for open source LLMs. For example, use the following command to deploy a Llama 3 8B hosted on HuggingFace:
python -m vllm.entrypoints.openai.api_server \
--model meta-llama/Meta-Llama-3-8B-Instruct \
--tensor-parallel-size 8 \
--dtype auto \
--api-key sk-123456789 \
--gpu-memory-utilization 0.9 \
--port 5000
Then we can initilize the extractor and checker with api_base
:
import os
from claim_processor import LLMExtractor, LLMChecker
# Use the same API key in the above command
os.environ["OPENAI_API_KEY"] = "sk-123456789"
# Note the prefix "openai/" here
model = "openai/meta-llama/Meta-Llama-3-8B-Instruct"
api_base = "http://0.0.0.0:5000/v1"
extractor = LLMExtractor(model=model, batch_size=8, api_base=api_base)
checker = LLMChecker(model=model, batch_size=8, api_base=api_base)
We fine-tuned a Mistral 7B model for claim extraction. Deploy it with vllm:
python -m vllm.entrypoints.openai.api_server \
--model dongyru/Mistral-7B-Claim-Extractor \
--tensor-parallel-size 8 \
--dtype auto \
--api-key sk-123456789 \
--gpu-memory-utilization 0.9 \
--port 5000
Then we can initilize the extractor as follows:
extractor = LLMExtractor(
model="openai/dongyru/Mistral-7B-Claim-Extractor",
batch_size=8,
api_base="http://0.0.0.0:5000/v1"
)
We also offer non-LLM checker for efficent checking:
from claim_processor import AlignScoreChecker, NLIChecker
# Details see paper: https://arxiv.org/abs/2305.16739
checker = AlignScoreChecker(device=0, batch_size=128)
# See https://huggingface.co/ynie/roberta-large-snli_mnli_fever_anli_R1_R2_R3-nli
checker = NLIChecker(device=0, batch_size=128)
Both the extractor and checker takes a batch of inputs:
# Batch of questions (optional)
questions = ['question 1', 'question 2']
# Batch of model responses
responses = ['response 1', 'response 2']
extraction_results = extractor.extract(
batch_responses=responses,
batch_questions=questions,
max_new_tokens=1000
)
batch_claims = [[c.content for c in res.claims] for res in extraction_results]
references = ['reference 1', 'reference 2']
batch_labels = checker.check(
batch_claims=batch_claims,
batch_references=references,
max_reference_segment_length=0
)
The extraction_results
is a list of RCClaim
objects defined in refchecker/base.py.
We provide a command-line interface to run RefChecker in a console:
usage: refchecker-cli [-h] --input_path INPUT_PATH --output_path OUTPUT_PATH
[--cache_dir CACHE_DIR]
[--extractor_name EXTRACTOR_NAME]
[--extractor_max_new_tokens EXTRACTOR_MAX_NEW_TOKENS]
[--claim_format {triplet, subsentence}]
[--checker_name CHECKER_NAME]
[--extractor_api_base EXTRACTOR_API_BASE]
[--checker_api_base CHECKER_API_BASE]
[--repc_classifier_name {svm,svm_ensemble,nn,nn_ensemble}]
[--retriever_name {google}]
[--aggregator_name {strict,soft,major}]
[--use_retrieval]
[--batch_size_extractor BATCH_SIZE_EXTRACTOR]
[--batch_size_checker BATCH_SIZE_CHECKER]
[{extract,check,extract-check}]
positional arguments:
{extract,check,extract-check}
extract: Extract claims from provided responses.
check: Check whether the provided claims are factual.
extract-check: Extract claims and check whether they are factual.
options:
-h, --help show this help message and exit
--input_path INPUT_PATH
Input path to the json file.
--output_path OUTPUT_PATH
Output path to the result json file.
--cache_dir CACHE_DIR
Path to the cache directory. Default: ./.cache.
--extractor_name EXTRACTOR_NAME
Model used for extracting claims. Default: bedrock/anthropic.claude-3-sonnet-20240229-v1:0
--extractor_max_new_tokens EXTRACTOR_MAX_NEW_TOKENS
Max generated tokens of the extractor, set a larger value for longer documents. Default: 500
--claim_format {triplet, subsentence}
The format of the extracted claims. Default: triplet
--checker_name CHECKER_NAME
Model used for checking whether the claims are factual. Default: bedrock/anthropic.claude-3-sonnet-20240229-v1:0
--extractor_api_base EXTRACTOR_API_BASE
API base URL if using vllm for deploying the extractor.
--checker_api_base CHECKER_API_BASE
API base URL if using vllm for deploying the checker
--repc_classifier_name {svm,svm_ensemble,nn,nn_ensemble}
Classifier Model used for RepC checker, only valid when RepC checker is used.
Default: nn_ensemble, neural network classifier with layer ensemble.
--retriever_name {google}
Model used for retrieving reference (currently only google is supported).
Default: google.
--aggregator_name {strict,soft,major}
Aggregator used for aggregating the results from multiple triplets.
Default: soft.
* strict: If any of the triplets is Contradiction, the response is
Contradiction. If all of the triplets are Entailment, the response is
Entailment. Otherwise, the response is Neutral.
* soft: The ratio of each category is calculated.
* major: The category with the most votes is selected.
--use_retriever
Whether to use retrieval to find the reference for checking. Required
if the reference field in input data is not provided.
--serper_api_key SERPER_API_KEY
Path to the serper api key file. Required if the google retriever is
used.
--batch_size_extractor BATCH_SIZE_EXTRACTOR
Batch size for batching inference of eatractor. Default: 16.
--batch_size_checker BATCH_SIZE_CHECKER
Batch size for batching inference of checker. Default: 16.
To extract claim triplets from LLM-generated responses, do:
claim_processor-cli extract \
--input_path {INPUT_PATH} \
--output_path {OUTPUT_PATH} \
--extractor_name {EXTRACTOR_NAME} \
--extractor_api_base {EXTRACTOR_API_BASE}
The input json file contains a list of
{
"response": "", # required, the response to be checked
"question": "", # optional if the question is not important (e.g., in summarization)
"reference": "", # required, the reference for checking
...
}
In the output json file, each item is added with a claims
field, containing a list of [head, relation, tail]
.
To check hallucinations at triplet level, do:
claim_processor-cli check \
--input_path {INPUT_PATH} \
--output_path {OUTPUT_PATH} \
--checker_name {CHECKER_NAME} \
--checker_api_base {CHECKER_API_BASE} \
--aggregator_name {strict,soft,major}
The input json file contains a list of
{
"response": "", # required, the response to be checked
"claims": [
["head1", "relation1", "tail1"],
["head2", "relation2", "tail2"],
...
] # required, the corresponding triplets of the response
"reference": "", # optional if a retriever is used to get reference
...
}
In the output json file, each item is added with the following fields:
{
"Y": Union[str, dict], # aggregated predictions on the whole response
"ys": [
"Entailment",
"Neutral",
"Contradiction",
...
] # checker predictions on each triplet
"reference": "", # added if a retriever is used to get reference
...
}
The format of aggregated predictions Y
depends on the selected aggregator. It is a str
as “Entailment”, “Neutral”, or “Contradiction” if strict
or major
aggregators are used. It is a dict
containing ratios of each category if the soft
aggregator is used. We additionally include a special category “Abstain” introduced in Evaluation Metric.
Note that the retriever is required in the zero-context setting, where no reference is provided by users. You can activate it by adding the --use_retriever
flag and specifying --retriever_name
. Currently we only support a google-based retriever. Feel free to try your own retrieval system and welcome to contribute.
For using the google retriever and/or the OpenAI models, you should provide corresponding API keys by specifying --serper_api_key
and/or --openai_key
.
Finally, you can use the whole extraction and checking pipeline by:
claim_processor-cli extract-check \
--input_path {INPUT_PATH} \
--output_path {OUTPUT_PATH} \
--extractor_name {EXTRACTOR_NAME} \
--checker_name {CHECKER_NAME} \
--extractor_api_base {EXTRACTOR_API_BASE} \
--checker_api_base {CHECKER_API_BASE} \
--aggregator_name {strict,soft,major} \
<other optional flags>
You can try the command-line with example scripts and example input data here.
LLMs exhibit a susceptibility to generate hallucinated contents that can be challenging to discern, possibly leading users astray. To address this, this project centers on the construction of a standardized assessment framework aimed at identifying such hallucinations present in the outputs of LLMs. Specifically, we try to answer the the following questions:
Table of Contents
Hallucinations are claims made by LLMs not supported by factual knowledge, which we refer to as references; detecting hallucinations involves comparing the claims against the references. This process depends on tasks, contexts, granularity of checking and how we categorize them. We will discuss them in turn.
When LLM generates a response, there is a great deal of variations as how contexts are provided which, in turn, determines where and how to identify the reference:
For both Noisy Context and Accurate Context tasks, we take the documents in the prompt as the references. The following figure illustrate the differences of the three settings.
Illustration of three settings of context, tasks and references.
Hallucination detection is challenging because non-factualness often creeps in and corrupts parts of a response subtly. Rather than declaring the entire response as hallucinatory or not, in this work we inspect claims that are embedded in a response. With that, the basic relationship between a response and its corresponding references can be visualized as a Venn diagram shown below.
These three categories align closely with the concepts of support
, refute
, and not enough information
within the fact-checking literature, and they are commonly used in Natural Language Inference (NLI).
Definition of Hallucinations.
Moving on, the remaining claims, represented by blue stars, contain information that is not addressed in the response. Whether this information should be incorporated into the response depends on the specific task at hand. In tasks like machine translation, omitting this information can result in a loss of information and should be considered a form of hallucination. In this release, we concentrate on question answering (QA) tasks, as well as text summarization and information extraction. In these tasks, missing facts are not regarded as hallucinations, their relevance may or may not be important. For example, in QA tasks the missing claims reflect retrieval quality instead; we leave this for future work.
The Granularity of Claim
Informally, claims are the unit for the checking. Previous works use sentences in the response as claims (SelfCheckGPT), or generate short phrases (i.e. sub-sentences) as the claims produced by LLM’s in-context learning (FActScore, FACTOOL). This work explores the approach of representing claims with knowledge triplets. This concept is inspired by the field of knowledge graph studies, where triplets are employed to encapsulate factual knowledge units. Knowledge triplets adopt a (subject, predicate, object)
structure to capture fine-grained information within the response. We call the triplet represented claims as Claim-Triplets. Here is an example of model response and the claim-triplets extracted by Claude 2 (see Triplet Extraction for the details of claim-triplet extraction):
Richard Mulligan played Mr. Kincaid on The Partridge Family.
Subject | Predicate | Object |
---|---|---|
Richard Mulligan | played | Mr. Kincaid |
Mr. Kincaid | chracter in | The Partridge Family |
We have assembled a benchmark dataset comprising 300 examples, with 100 examples for each of the three settings mentioned earlier. The examples are randomly sampled from the data sources listed in the following table:
Setting | Data Source | Task | References |
---|---|---|---|
Zero Context | NaturalQuestions (dev set) | Closed Book QA | Annotated Long Answer |
Noisy Context | MS MARCO (dev set) | RAG | Retrieved Passages |
Accurate Context | databricks-dolly-15k | Summarization, Closed QA, Information Extraction | Input Context |
We collect responses from 7 LLMs on the benchmark: GPT4, GPT-3.5-Turbo, InstructGPT, Falcon (Falcon-40B-Instruct), Alpaca (Alpaca-7B), LLaMA2(70B-Chat) and Claude 2. The code and examples for response collection can be found in response_collection.
We performed a human evaluation of responses generated by seven LLMs on this benchmark dataset. The process is shown in the figure below which involved three steps: gathering responses, extracting claim-triplets, and asking human annotators to evaluate these claims. 23% of the claim-triplets were double annotated, with 95.0% Inter-Annotator Agreement. We employ Claude 2 for the claim-triplet extraction for human evaluations, but it can be replaced with any triplet extraction model. During the annotation, we also ask the annotators to mark low quality triplets. These identified low quality triplets are then removed from the benchmark dataset. We will release the data and results upon approval.
The evaluation process under the Zero Context setting as an example.
We evaluate using the frequency of each hallucination label. For example, if there are 3 Entailment, 5 Neutral, and 2 Contradiction claims out of 10 in a specific response, the rates for Entailment, Neutral, and Contradiction in this response are 0.3, 0.5, and 0.2 respectively. The overall rates for each label are averaged across all responses in the benchmark to obtain the final evaluation metrics. Alternatively, we can aggregate at response level according to some rules, the most aggressive is to classify a response as hallucination if it contains at least one hallucinated claim triplet.
Sometimes, the LLM declines to answer questions by generating responses such as I'm sorry, I can't provide an accurate answer without more context. Could you please provide more information? When this happens, the claim-triplet extractor fails to get triplets from these responses. So, we introduce a new label called Abstain
to for such cases. We treat these responses as individual claims, and in such cases, the Abstain Rate is 1 for that specific response. In practice, if the claim-triplet extractor doesn't give any triplets for a response, we treat this response as Abstain.
Using automatic hallucination checker is a more scalable way for evaluating LLMs. Our automatic hallucination checker is a 3-stage pipeline, consisting of a knowledge extraction model $E$, a checker $C$, and aggregation rules $\tau$. Initially, we utilize $E$ to decompose the input text $T$ into a set of knowledge triplets $k_{1:N}$. Each of these triplets undergoes verification by $C$. Subsequently, based on predefined rules $\tau$, the individual results $y_{1:N}$ are aggregated to determine the overall hallucination label $Y$ for the given text. We delve into the specifics of each component in the subsequent parts and also elaborate on our strategies for enhancing the checker's performance through self-supervision.
Our checking framework hinges on a key assumption: the decomposition of the original text into triplets facilitates finer-grained detection and more accurate evaluation. The extraction of these triplets plays a pivotal role in achieving this objective. We use LLMs to extract knowledge triplets from the given text. See refchecker/extractor for further details and the usage of the knowledge extraction model.
With triplets extracted for the given text, the checker $C$ in the second stage needs to predict hallucination labels on all extracted triplets. We are able to employ numerous existing zero-shot checkers without additional training. We mainly consider two varieties: LLM-based checkers and NLI-based checkers. LLM-based checkers query LLMs to obtain predictions, exhibiting noteworthy success in recent studies. NLI-based checkers adopts much smaller pre-trained language models (such as RoBERTa) to perform text classification, originating from the natural language inference task. See refchecker/checker for more details.
After collecting fine-grained individual results at triplet-level, we aggregate them to get the overall hallucination label for the entire input text. Based on the definition of the three categories, we define and apply the following intuitive and rigorous aggregation rules in our work.
\tau:Y=\begin{cases} \text{Entailment}&\text{if all } y_i \text{ are Entailment}, \\ \text{Contradiction}&\text{if there exists } y_i \text{ that is Contradiction},\\ \text{Neutral}& \text{otherwise}.\end{cases}
There are many other choices for the aggregation stage. For example, we can count the number of "Neutral" and "Contradiction" triplets, then identify those with the ratio beyond a pre-defined threshold as hallucinated responses. The aggregation rules τ can be customized according to different applications with distinct hallucination tolerance.
Hallucination detection results can be inferred from certain text spans in the reference, which refers to "evidence". After we obtain the hallucination predictions on triplets, it is meaningful to locate the corresponding evidence in the reference and triplets in the checked text. As explanations for checking results, they provide signals for hallucination mitigation solutions. We use a simple embedding-based method to locate them as certain text spans. It offers an effective baseline for straightforward scenarios where checkers require only a single directly matched evidence for predictions.
We split reference or the checked text into spans and compare their similarity with elements in triplets. For spans or elements in triplets, we obtain corresponding embeddings as average-pooled token representations by a RoBERTa model. We then compute the pairwise similarity as the cosine distance between a pair of embeddings. Spans matched to elements in triplets with high similarities are considered as localized evidence or triplets.
Localization in Reference
Intuitively, for an entailed claim triplet, we should find all of its constituents in reference. On the other hand, we expect none of them will appear for neutral claim triplets. Finally, contradictory triplets often have missing components. These cases are shown blow:
Localization Hallucination in Response
Localization in response provides hints for which parts in the response are problematic. We can either conservatively suppress (for "Neutral" triplets) or modify them (for "Contradictory"). Here is an example:
Limitations
Localization is the reverse problem to extraction, and from our experience, making it robust can be a non-trivial research problem. Here are some challenges:
The example below showcases both issues above:
Reference: Bob got his first son Clark in 1982. Two little sisters of Clark were born after 4 years. And he welcomed his youngest son last year.
Triplet: Bob has three children.
In this example, almost the whole reference should be regarded as the evidence. Besides, it requires an implicit addition operation to get the implicit evidence "Bob has four children" that can be directly compared with the triplet.
Our RefChecker currently has certain limitations that we are actively addressing to enhance the framework. We invite more contributors to join the discussion and share their perspectives on these issues. There are improvements needed for all the components; the RefChecker architecture has a modular architecture that allows independent updates. Here are some helps we felt needed (We welcome contributions through pull requests):
Then there is an array of related problems:
Finally, while RefChecker is in the category of checkers that offer more explainability, it will be very interesting to compare with other approaches that rely on uncertainty at generation time (e.g. SelfCheckGPT).
See CONTRIBUTING for more information.
The code in this project is licensed under the Apache-2.0 License.
FAQs
Claim Processor provides automatic checking pipeline for detecting fine-grained hallucinations generated by Large Language Models.
We found that claim-processor demonstrated a healthy version release cadence and project activity because the last version was released less than a year ago. It has 1 open source maintainer collaborating on the project.
Did you know?
Socket for GitHub automatically highlights issues in each pull request and monitors the health of all your open source dependencies. Discover the contents of your packages and block harmful activity before you install or update your dependencies.
Security News
Opengrep forks Semgrep to preserve open source SAST in response to controversial licensing changes.
Security News
Critics call the Node.js EOL CVE a misuse of the system, sparking debate over CVE standards and the growing noise in vulnerability databases.
Security News
cURL and Go security teams are publicly rejecting CVSS as flawed for assessing vulnerabilities and are calling for more accurate, context-aware approaches.