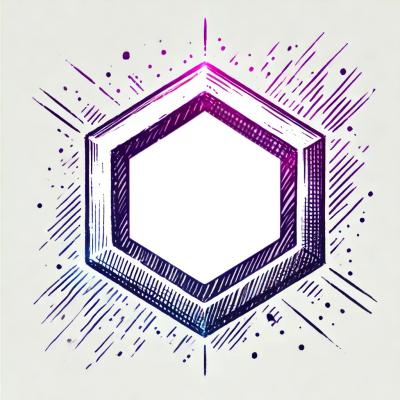
Security News
Maven Central Adds Sigstore Signature Validation
Maven Central now validates Sigstore signatures, making it easier for developers to verify the provenance of Java packages.
fast-langdetect
is an ultra-fast and highly accurate language detection library based on FastText, a library developed by Facebook. Its incredible speed and accuracy make it 80x faster than conventional methods and deliver up to 95% accuracy.
3.9
to 3.13
.numpy
required (thanks to @dalf).Background
This project builds upon zafercavdar/fasttext-langdetect with enhancements in packaging. For more information about the underlying model, see the official FastText documentation: Language Identification.
Possible memory usage
This library requires at least 200MB memory in low-memory mode.
To install fast-langdetect, you can use either pip
or pdm
:
pip install fast-langdetect
pdm add fast-langdetect
In scenarios where accuracy is important, you should not rely on the detection results of small models, use low_memory=False
to download larger models!
/tmp/fasttext-langdetect
directory upon first use.from fast_langdetect import detect, detect_multilingual
# Single language detection
print(detect("Hello, world!"))
# Output: {'lang': 'en', 'score': 0.12450417876243591}
# `use_strict_mode` determines whether the model loading process should enforce strict conditions before using fallback options.
# If `use_strict_mode` is set to True, we will load only the selected model, not the fallback model.
print(detect("Hello, world!", low_memory=False, use_strict_mode=True))
# How to deal with multiline text
multiline_text = """
Hello, world!
This is a multiline text.
But we need remove `\n` characters or it will raise an ValueError.
REMOVE \n
"""
multiline_text = multiline_text.replace("\n", "")
print(detect(multiline_text))
# Output: {'lang': 'en', 'score': 0.8509423136711121}
print(detect("Привет, мир!")["lang"])
# Output: ru
# Multi-language detection with low memory mode enabled
# The accuracy is not as good as it should be
print(detect_multilingual("Hello, world!你好世界!Привет, мир!"))
# Output: [{'lang': 'ja', 'score': 0.32009604573249817}, {'lang': 'uk', 'score': 0.27781224250793457}, {'lang': 'zh', 'score': 0.17542070150375366}, {'lang': 'sr', 'score': 0.08751443773508072}, {'lang': 'bg', 'score': 0.05222449079155922}]
# Multi-language detection with low memory mode disabled
print(detect_multilingual("Hello, world!你好世界!Привет, мир!", low_memory=False))
# Output: [{'lang': 'ru', 'score': 0.39008623361587524}, {'lang': 'zh', 'score': 0.18235979974269867}, {'lang': 'ja', 'score': 0.08473210036754608}, {'lang': 'sr', 'score': 0.057975586503744125}, {'lang': 'en', 'score': 0.05422825738787651}]
We provide a fallback mechanism: when use_strict_mode=False
, if the program fails to load the large model (low_memory=False
), it will fall back to the offline small model to complete the prediction task.
detect_language
Functionfrom fast_langdetect import detect_language
# Single language detection
print(detect_language("Hello, world!"))
# Output: EN
print(detect_language("Привет, мир!"))
# Output: RU
print(detect_language("你好,世界!"))
# Output: ZH
For text splitting based on language, please refer to the split-lang repository.
For detailed benchmark results, refer to zafercavdar/fasttext-langdetect#benchmark.
[1] A. Joulin, E. Grave, P. Bojanowski, T. Mikolov, Bag of Tricks for Efficient Text Classification
@article{joulin2016bag,
title={Bag of Tricks for Efficient Text Classification},
author={Joulin, Armand and Grave, Edouard and Bojanowski, Piotr and Mikolov, Tomas},
journal={arXiv preprint arXiv:1607.01759},
year={2016}
}
[2] A. Joulin, E. Grave, P. Bojanowski, M. Douze, H. Jégou, T. Mikolov, FastText.zip: Compressing text classification models
@article{joulin2016fasttext,
title={FastText.zip: Compressing text classification models},
author={Joulin, Armand and Grave, Edouard and Bojanowski, Piotr and Douze, Matthijs and J{\'e}gou, H{\'e}rve and Mikolov, Tomas},
journal={arXiv preprint arXiv:1612.03651},
year={2016}
}
FAQs
Quickly detect text language and segment language
We found that fast-langdetect demonstrated a healthy version release cadence and project activity because the last version was released less than a year ago. It has 1 open source maintainer collaborating on the project.
Did you know?
Socket for GitHub automatically highlights issues in each pull request and monitors the health of all your open source dependencies. Discover the contents of your packages and block harmful activity before you install or update your dependencies.
Security News
Maven Central now validates Sigstore signatures, making it easier for developers to verify the provenance of Java packages.
Security News
CISOs are racing to adopt AI for cybersecurity, but hurdles in budgets and governance may leave some falling behind in the fight against cyber threats.
Research
Security News
Socket researchers uncovered a backdoored typosquat of BoltDB in the Go ecosystem, exploiting Go Module Proxy caching to persist undetected for years.