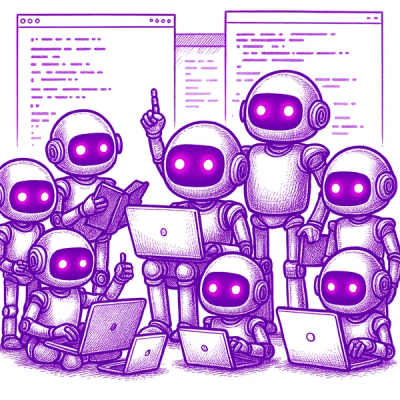
Security News
Open Source CAI Framework Handles Pen Testing Tasks up to 3,600Ć Faster Than Humans
CAI is a new open source AI framework that automates penetration testing tasks like scanning and exploitation up to 3,600Ć faster than humans.
graphblas-algorithms
Advanced tools
graphblas-algorithms
is a collection of GraphBLAS algorithms written using
python-graphblas
.
It may be used directly or as an experimental
backend to NetworkX.
Why use GraphBLAS Algorithms? Because it is fast, flexible, and familiar by using the NetworkX API.
Are we missing any algorithms that you want?
Please let us know!
conda install -c conda-forge graphblas-algorithms
pip install graphblas-algorithms
First, create a GraphBLAS Matrix.
import graphblas as gb
M = gb.Matrix.from_coo(
[0, 0, 1, 2, 2, 3],
[1, 3, 0, 0, 1, 2],
[1., 2., 3., 4., 5., 6.],
nrows=4, ncols=4, dtype='float32'
)
Next wrap the Matrix as ga.Graph
.
import graphblas_algorithms as ga
G = ga.Graph(M)
Finally call an algorithm.
hubs, authorities = ga.hits(G)
When the result is a value per node, a gb.Vector
will be returned.
In the case of HITS,
two Vectors are returned representing the hubs and authorities values.
Algorithms whose result is a subgraph will return ga.Graph
.
Dispatching to plugins is a new feature in Networkx 3.0.
When both networkx
and graphblas-algorithms
are installed in an
environment, calls to NetworkX algorithms can be dispatched to the
equivalent version in graphblas-algorithms
.
import networkx as nx
import graphblas_algorithms as ga
# Generate a random graph (5000 nodes, 1_000_000 edges)
G = nx.erdos_renyi_graph(5000, 0.08)
# Explicitly convert to ga.Graph
G2 = ga.Graph.from_networkx(G)
# Pass G2 to NetworkX's k_truss
T5 = nx.k_truss(G2, 5)
G2
is not a nx.Graph
, but it does have an attribute
__networkx_plugin__ = "graphblas"
. This tells NetworkX to
dispatch the k_truss call to graphblas-algorithms. This link
connection exists because graphblas-algorithms registers
itself as a "networkx.plugin" entry point.
The result T5
is a ga.Graph
representing the 5-truss structure of the
original graph. To convert to a NetworkX Graph, use:
T5.to_networkx()
Note that even with the conversions to and from ga.Graph
, this example still runs 10x
faster than using the native NetworkX k-truss implementation. Speed improvements scale
with graph size, so larger graphs will see an even larger speed-up relative to NetworkX.
The following NetworkX algorithms have been implemented by graphblas-algorithms and can be used following the dispatch pattern shown above.
graphblas_algorithms.nxapi
āāā boundary
ā āāā edge_boundary
ā āāā node_boundary
āāā centrality
ā āāā degree_alg
ā ā āāā degree_centrality
ā ā āāā in_degree_centrality
ā ā āāā out_degree_centrality
ā āāā eigenvector
ā ā āāā eigenvector_centrality
ā āāā katz
ā āāā katz_centrality
āāā cluster
ā āāā average_clustering
ā āāā clustering
ā āāā generalized_degree
ā āāā square_clustering
ā āāā transitivity
ā āāā triangles
āāā community
ā āāā quality
ā āāā inter_community_edges
ā āāā intra_community_edges
āāā components
ā āāā connected
ā ā āāā is_connected
ā ā āāā node_connected_component
ā āāā weakly_connected
ā āāā is_weakly_connected
āāā core
ā āāā k_truss
āāā cuts
ā āāā boundary_expansion
ā āāā conductance
ā āāā cut_size
ā āāā edge_expansion
ā āāā mixing_expansion
ā āāā node_expansion
ā āāā normalized_cut_size
ā āāā volume
āāā dag
ā āāā ancestors
ā āāā descendants
āāā dominating
ā āāā is_dominating_set
āāā efficiency_measures
ā āāā efficiency
āāā generators
ā āāā ego
ā āāā ego_graph
āāā isolate
ā āāā is_isolate
ā āāā isolates
ā āāā number_of_isolates
āāā isomorphism
ā āāā isomorph
ā āāā fast_could_be_isomorphic
ā āāā faster_could_be_isomorphic
āāā linalg
ā āāā bethehessianmatrix
ā ā āāā bethe_hessian_matrix
ā āāā graphmatrix
ā ā āāā adjacency_matrix
ā āāā laplacianmatrix
ā ā āāā laplacian_matrix
ā ā āāā normalized_laplacian_matrix
ā āāā modularitymatrix
ā āāā directed_modularity_matrix
ā āāā modularity_matrix
āāā link_analysis
ā āāā hits_alg
ā ā āāā hits
ā āāā pagerank_alg
ā āāā google_matrix
ā āāā pagerank
āāā lowest_common_ancestors
ā āāā lowest_common_ancestor
āāā operators
ā āāā binary
ā ā āāā compose
ā ā āāā difference
ā ā āāā disjoint_union
ā ā āāā full_join
ā ā āāā intersection
ā ā āāā symmetric_difference
ā ā āāā union
ā āāā unary
ā āāā complement
ā āāā reverse
āāā reciprocity
ā āāā overall_reciprocity
ā āāā reciprocity
āāā regular
ā āāā is_k_regular
ā āāā is_regular
āāā shortest_paths
ā āāā dense
ā ā āāā floyd_warshall
ā ā āāā floyd_warshall_numpy
ā ā āāā floyd_warshall_predecessor_and_distance
ā āāā generic
ā ā āāā has_path
ā āāā unweighted
ā ā āāā all_pairs_shortest_path_length
ā ā āāā single_source_shortest_path_length
ā ā āāā single_target_shortest_path_length
ā āāā weighted
ā āāā all_pairs_bellman_ford_path_length
ā āāā bellman_ford_path
ā āāā bellman_ford_path_length
ā āāā negative_edge_cycle
ā āāā single_source_bellman_ford_path_length
āāā simple_paths
ā āāā is_simple_path
āāā smetric
ā āāā s_metric
āāā structuralholes
ā āāā mutual_weight
āāā tournament
ā āāā is_tournament
ā āāā score_sequence
ā āāā tournament_matrix
āāā traversal
ā āāā breadth_first_search
ā āāā bfs_layers
ā āāā descendants_at_distance
āāā triads
āāā is_triad
FAQs
Graph algorithms written in GraphBLAS and backend for NetworkX
We found that graphblas-algorithms demonstrated a healthy version release cadence and project activity because the last version was released less than a year ago.Ā It has 2 open source maintainers collaborating on the project.
Did you know?
Socket for GitHub automatically highlights issues in each pull request and monitors the health of all your open source dependencies. Discover the contents of your packages and block harmful activity before you install or update your dependencies.
Security News
CAI is a new open source AI framework that automates penetration testing tasks like scanning and exploitation up to 3,600Ć faster than humans.
Security News
Deno 2.4 brings back bundling, improves dependency updates and telemetry, and makes the runtime more practical for real-world JavaScript projects.
Security News
CVEForecast.org uses machine learning to project a record-breaking surge in vulnerability disclosures in 2025.