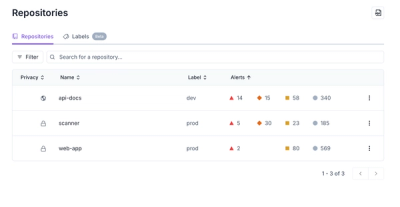
Product
Redesigned Repositories Page: A Faster Way to Prioritize Security Risk
Our redesigned Repositories page adds alert severity, filtering, and tabs for faster triage and clearer insights across all your projects.
Pytorch implementation of echo state networks for static graphs and discrete-time dynamic graphs.
Easiest way to get our library is via python package:
pip install graphesn
The library is quite straightforward to use:
from graphesn import StaticGraphReservoir, Readout, initializer
from torch_geometric.data import Data
data = Data(...)
reservoir = StaticGraphReservoir(num_layers=3, in_features=8, hidden_features=16)
reservoir.initialize_parameters(recurrent=initializer('uniform', rho=.9), input=initializer('uniform', scale=1))
embeddings = reservoir(data.edge_index, data.x)
readout = Readout(num_features=reservoir.out_features, num_targets=3)
readout.fit(data=(embeddings, data.y), regularization=1e-3)
predictions = readout(embeddings)
The library is contained in folder src/graphesn
:
reservoir.py
implementation of reservoirs for static and discrete-time dynamic graphs;matrix.py
random matrices generating functions;readout.py
implementation of a linear readout for large-scale ridge regression;data.py
classes to represent temporal and dynamic graphs;dataset.py
some dynamic graph datasets;util.py
general utilities.The examples
folder contains demos for our library on some common graph datasets.
This research software is provided as-is. We are working on this library in our spare time.
Code is released under the MIT license, see LICENSE
for details.
If you find a bug, please open an issue to report it, and we will do our best to solve it. For general or technical questions, please email us rather than opening an issue.
FAQs
Python implementation of Deep Graph Echo State Networks
We found that graphesn demonstrated a healthy version release cadence and project activity because the last version was released less than a year ago. It has 3 open source maintainers collaborating on the project.
Did you know?
Socket for GitHub automatically highlights issues in each pull request and monitors the health of all your open source dependencies. Discover the contents of your packages and block harmful activity before you install or update your dependencies.
Product
Our redesigned Repositories page adds alert severity, filtering, and tabs for faster triage and clearer insights across all your projects.
Security News
Multiple deserialization flaws in PyTorch Lightning could allow remote code execution when loading untrusted model files, affecting versions up to 2.4.0.
Security News
NVD now marks all pre-2018 CVEs as "Deferred," signaling it will no longer enrich older vulnerabilities, further eroding trust in its data.