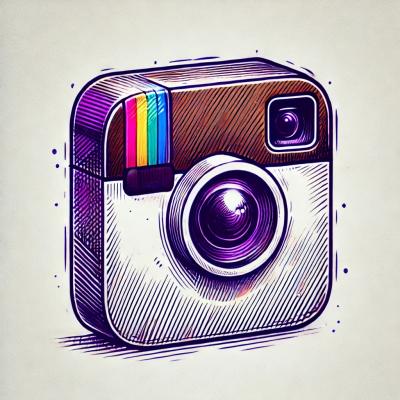
Research
PyPI Package Disguised as Instagram Growth Tool Harvests User Credentials
A deceptive PyPI package posing as an Instagram growth tool collects user credentials and sends them to third-party bot services.
Library that contains implementations of machine learning components in the hyperbolic space
This library implements common Neural Network components in the hyperbolic space (using the Poincare model). The implementation of this library uses Tensorflow as a backend and can easily be used with Keras and is meant to help Data Scientists, Machine Learning Engineers, Researchers and others to implement hyperbolic neural networks.
You can also use this library for uses other than neural networks by using the mathematical functions available in the Poincare class. In the future we may implement components that can be used in models other than neural networks. You can learn more about Hyperbolic networks here, and in the references1 2 3 4.
The recommended way to install is with pip
pip install hyperlib
To build from source, you need to compile the pybind11 extensions.
For example to build on linux:
conda -n hyperlib python=3.8 gxx_linux-64 pybind11
python setup.py install
Hyperlib works with python>=3.8 and tensorflow>=2.0.
Creating a hyperbolic neural network using Keras:
import tensorflow as tf
from tensorflow import keras
from hyperlib.nn.layers.lin_hyp import LinearHyperbolic
from hyperlib.nn.optimizers.rsgd import RSGD
from hyperlib.manifold.poincare import Poincare
# Create layers
hyperbolic_layer_1 = LinearHyperbolic(32, Poincare(), 1)
hyperbolic_layer_2 = LinearHyperbolic(32, Poincare(), 1)
output_layer = LinearHyperbolic(10, Poincare(), 1)
# Create optimizer
optimizer = RSGD(learning_rate=0.1)
# Create model architecture
model = tf.keras.models.Sequential([
hyperbolic_layer_1,
hyperbolic_layer_2,
output_layer
])
# Compile the model with the Riemannian optimizer
model.compile(
optimizer=optimizer,
loss=tf.keras.losses.SparseCategoricalCrossentropy(from_logits=True),
metrics=[tf.keras.metrics.SparseCategoricalAccuracy()],
)
Using math functions on the Poincare ball:
import tensorflow as tf
from hyperlib.manifold.poincare import Poincare
p = Poincare()
# Create two matrices
a = tf.constant([[5.0,9.4,3.0],[2.0,5.2,8.9],[4.0,7.2,8.9]])
b = tf.constant([[4.8,1.0,2.3]])
# Matrix multiplication on the Poincare ball
curvature = 1
p.mobius_matvec(a, b, curvature)
A big advantage of hyperbolic space is its ability to represent hierarchical data. There are several techniques for embedding data in hyperbolic space; the most common is gradient methods 5.
If your data has a natural metric you can also use TreeRep6. Input a symmetric distance matrix, or a compressed distance matrix
import numpy as np
from hyperlib.embedding.treerep import treerep
from hyperlib.embedding.sarkar import sarkar_embedding
# Example: immunological distances between 8 mammals by Sarich
compressed_metric = np.array([
32., 48., 51., 50., 48., 98., 148.,
26., 34., 29., 33., 84., 136.,
42., 44., 44., 92., 152.,
44., 38., 86., 142.,
42., 89., 142.,
90., 142.,
148.
])
# outputs a weighted networkx Graph
tree = treerep(compressed_metric, return_networkx=True)
# embed the tree in 2D hyperbolic space
root = 0
embedding = sarkar_embedding(tree, root, tau=0.5)
Please see the examples directory for complete examples.
Chami, I., Ying, R., Ré, C. and Leskovec, J. Hyperbolic Graph Convolutional Neural Networks. NIPS 2019. ↩
Nickel, M. and Kiela, D. Poincaré embeddings for learning hierarchical representations. NIPS 2017. ↩
Khrulkov, Mirvakhabova, Ustinova, Oseledets, Lempitsky. Hyperbolic Image Embeddings. ↩
Wei Peng, Varanka, Mostafa, Shi, Zhao. Hyperbolic Deep Neural Networks: A Survey. ↩
De Sa et. al. Representation Tradeoffs for Hyperbolic Embeddings ↩
Rishi Sonthalia and Anna Gilbert. Tree! I am no Tree! I am a Low Dimensional Hyperbolic Embedding ↩
FAQs
Library that contains implementations of machine learning components in the hyperbolic space
We found that hyperlib demonstrated a healthy version release cadence and project activity because the last version was released less than a year ago. It has 1 open source maintainer collaborating on the project.
Did you know?
Socket for GitHub automatically highlights issues in each pull request and monitors the health of all your open source dependencies. Discover the contents of your packages and block harmful activity before you install or update your dependencies.
Research
A deceptive PyPI package posing as an Instagram growth tool collects user credentials and sends them to third-party bot services.
Product
Socket now supports pylock.toml, enabling secure, reproducible Python builds with advanced scanning and full alignment with PEP 751's new standard.
Security News
Research
Socket uncovered two npm packages that register hidden HTTP endpoints to delete all files on command.