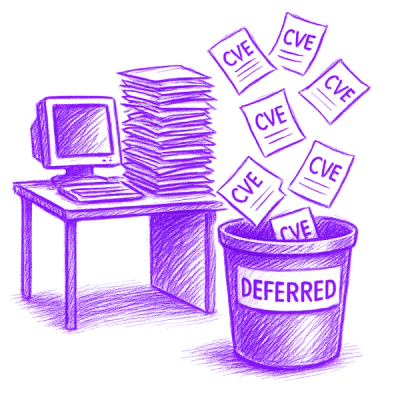
Security News
NVD Quietly Sweeps 100K+ CVEs Into a “Deferred” Black Hole
NVD now marks all pre-2018 CVEs as "Deferred," signaling it will no longer enrich older vulnerabilities, further eroding trust in its data.
Library for managing hyperparameters and mutable state of machine learning training systems.
Opinionated library for managing hyperparameter configs and mutable program state of machine learning training systems.
Key Features:
Install with pip:
pip install hyperstate
All you need to use HyperState is a (nested) dataclass for your hyperparameters:
from dataclasses import dataclass
@dataclass
class OptimizerConfig:
lr: float = 0.003
batch_size: int = 512
@dataclass
class NetConfig:
hidden_size: int = 128
num_layers: int = 2
@dataclass
class Config:
optimizer: OptimizerConfig
net: NetConfig
steps: int = 100
The hyperstate.load
function can load values from a config file and/or apply specific overrides from the command line.
import argparse
import hyperstate
if __name__ == "__main__":
parser = argparse.ArgumentParser()
parser.add_argument("--config", type=str, default=None, help="Path to config file")
parser.add_argument("--hps", nargs="+", help="Override hyperparameter value")
args = parser.parse_args()
config = hyperstate.load(Config, file=args.config, overrides=args.hps)
print(config)
$ python main.py --hps net.num_layers=96 steps=50
Config(optimizer=OptimizerConfig(lr=0.003, batch_size=512), net=NetConfig(hidden_size=128, num_layers=96), steps=50)
$ cat config.ron
Config(
optimizer: (
lr: 0.05,
batch_size: 4096,
),
)
$ python main.py --config=config.ron
Config(optimizer=OptimizerConfig(lr=0.05, batch_size=4096), net=NetConfig(hidden_size=128, num_layers=2), steps=100)
The full code for this example can be found in examples/basic-config.
Learn more about:
HyperState supports a strictly typed subset of Python objects:
Dict
, List
, Tuple
, Optional
int
, float
, str
, Enum
hyperstate.Serializable
Use hyperstate.dump
to serialize configs.
The second argument to dump
is a path to a file, and can be omitted to return the serialized config as a string instead of saving it to a file:
>>> print(hyperstate.dump(Config(lr=0.1, batch_size=256))
Config(
lr: 0.1,
batch_size: 256,
)
Use hyperstate.load
to deserialize configs.
The load
method takes the type of the config as the first argugment, and allows you to optionally specify the path to a config file and/or a List[str]
of overrides:
@dataclass
class OptimizerConfig:
lr: float
batch_size: int
@dataclass
class Config:
optimzer: OptimizerConfig
steps: int
config = hyperstate.load(Config, file="config.ron", overrides=["optimizer.lr=0.1", "steps=100"])
Versioning allows you to modify your Config
class while still remaining compatible with checkpoints recorded at previous version.
To benefit from versionining, your config must inherit hyperstate.Versioned
and implement its version
function:
@dataclass
class Config(hyperstate.Versioned):
lr: float
batch_size: int
@classmethod
def version(clz) -> int:
return 0
When serializing the config, hyperstate will now record an additional version
field with the value of the current version.
Any snapshots that contain configs without a version field are assumed to have a version of 0
.
RewriteRule
Now suppose you modify your Config
class, e.g. by renaming the lr
field to learning_rate
.
To still be able to load old configs that are using lr
instead of learning_rate
, you increase the version
to 1
and add an entry to the dictionary returned by upgrade_rules
that tells HyperState to change lr
to learning_rate
when upgrading configs from version 0
.
from dataclasses import dataclass
from typing import Dict, List
from hyperstate import Versioned
from hyperstate.schema.rewrite_rule import RenameField, RewriteRule
@dataclass
class Config(Versioned):
learning_rate: float
batch_size: int
@classmethod
def version(clz) -> int:
return 1
@classmethod
def upgrade_rules(clz) -> Dict[int, List[RewriteRule]]:
"""
Returns a list of rewrite rules that can be applied to the given version
to make it compatible with the next version.
"""
return {
0: [RenameField(old_field=("lr",), new_field=("learning_rate",))],
}
In the majority of cases, you don't actually have to manually write out RewriteRule
s.
Instead, they are generated for you automatically by the Schema Evolution CLI.
HyperState comes with a command line tool for managing changes to your config schema. To access the CLI, simply add the following code to the Python file defining your config:
# config.py
from hyperstate import schema_evolution_cli
if __name__ == "__main__":
schema_evolution_cli(Config)
Run python config.py
to see a list of available commands, described in more detail below.
dump-schema
The dump-schema
command creates a file describing the schema of your config.
This file should commited to version control, and is used to detect changes to the config schema and perform automatic upgrades.
check-schema
The check-schema
command compares your config class to a schema file and detects any backwards incompatible changes.
It also emits a suggested list of RewriteRule
s that can upgrade old configs to the new schema.
HyperState does not always guess the correct RewriteRule
s so you still need to check that they are correct.
$ python config.py check-schema
WARN field renamed to learning_rate: lr
WARN schema changed but version identical
Schema incompatible
Proposed mitigations
- add upgrade rules:
0: [
RenameField(old_field=('lr',), new_field=('learning_rate',)),
],
- bump version to 1
upgrade-schema
The upgrade-schema
command functions much the same as check-schema
, but also updates your schema config files once all backwards-incompatability issues have been address.
upgrade-config
The upgrade-config
command takes a list of paths to config files, and upgrades them to the latest version.
To prevent accidental backwards-incompatible modifications of your Config
class, you can use the following code as an automated test that checks your config Class
against a schema file created with dump-schema
:
from hyperstate.schema.schema_change import Severity
from hyperstate.schema.schema_checker import SchemaChecker
from hyperstate.schema.types import load_schema
from config import Config
def test_schema():
old = load_schema("config-schema.ron")
checker = SchemaChecker(old, Config)
if checker.severity() >= Severity.WARN:
checker.print_report()
assert checker.severity() == Severity.INFO
Serializable
You can define custom serialization logic for a class by inheriting from hyperstate.Serializable
and implementing the serialize
and deserialize
methods.
from dataclasses import dataclass
import torch
import torch.nn as nn
import torch.nn.functional as F
import hyperstate
@dataclass
class Config:
inputs: int
class LinearRegression(nn.Module, hyperstate.Serializable):
def __init__(self, inputs):
super(Net, self).__init__()
self.fc1 = nn.Linear(inputs, 1)
def forward(self, x):
return self.fc1(x)
# `serialize` should return a representation of the object consisting only of
# primitives, containers, numpy arrays, and torch tensors.
def serialize(self) -> Any:
return self.state_dict()
# `deserialize` should take a serialized representation of the object and
# return an instance of the class. The `ctx` argument allows you to pass
# additional information to the deserialization function.
@classmethod
def deserialize(clz, state_dict, ctx):
net = clz(ctx["config"].inputs)
return net.load_state_dict(state_dict)
@dataclass
class State:
net: LinearRegression
config = hyperstate.load("config.ron")
state = hyperstate.load("state.ron", ctx={"config": config})
Objects that implement Serializable
are stored in separate files using a binary encoding.
In the above example, calling hyperstate.dump(state, "checkpoint/state.ron")
will result in the following file structure:
checkpoint
├── state.net.blob
└── state.ron
Lazy
If you inherit from hyperstate.Lazy
, any fields with Serializable
types will only be loaded/deserialized when accessed. If the .blob
file for a field is missing, HyperState will not raise an error unless the corresponding field is accessed.
blob
To include objects in your state that do not directly implement hyperstate.Serializable
, you can seperately implement hyperstate.Serializable
and use the blob
function to mix in the Serializable
implementation:
import torch.optim as optim
import torch.nn as nn
import hyperstate
class SerializableOptimizer(hyperstate.Serializable):
def serialize(self):
return self.state_dict()
@classmethod
def deserialize(clz, state_dict: Any, config: Config, state: "State") -> optim.Optimizer:
optimizer = blob(optim.SerializableAdam, mixin=SerializableOptimizer)(state.net.parameters())
optimizer.load_state_dict(state_dict)
return optimizer
@dataclass
class State(hyperstate.Lazy):
net: nn.Module
optimizer: blob(Adam, mixin=SerializableOptimizer)
HyperState
To unlock the full power of HyperState, you must inherit from the HyperState
class.
This class combines an immutable config and mutable state, and provides automatic checkpointing, hyperparameter schedules, and the on-the-fly changes to the config and state (not implemented yet).
from dataclasses import dataclass
import torch
import torch.nn as nn
import torch.nn.functional as F
import hyperstate
@dataclass
class Config:
inputs: int
steps: int
class LinearRegression(nn.Module, hyperstate.Serializable):
def __init__(self, inputs):
super(Net, self).__init__()
self.fc1 = nn.Linear(inputs, 1)
def forward(self, x):
return self.fc1(x)
def serialize(self) -> Any:
return self.state_dict()
@classmethod
def deserialize(clz, state_dict, ctx):
net = clz(ctx["config"].inputs)
return net.load_state_dict(state_dict)
@dataclass
class State:
net: LinearRegression
step: int
class Trainer(HyperState[Config, State]):
def __init__(
self,
# Path to the config file
initial_config: str,
# Optional path to the checkpoint directory, which enables automatic checkpointing.
# If any checkpoint files are present, they will be used to initialize the state.
checkpoint_dir: Optional[str] = None,
# List of manually specified config overrides.
config_overrides: Optional[List[str]] = None,
):
super().__init__(Config, State, initial_config, checkpoint_dir, overrides=config_overrides)
def initial_state(self) -> State:
"""
This function is called to initialize the state if no checkpoint files are found.
"""
return State(net=LinearRegression(self.config.inputs))
def train(self) -> None:
for step in range(self.state.step, self.config.steps):
# training code...
self.state.step = step
# At the end of each iteration, call `self.step()` to checkpoint the state and apply hyperparameter schedules.
self.step()
When using the HyperState
object, the config and state are automatically checkpointed to the configured directory when calling the step
method.
Any int
/float
fields in the config can also be set to a schedule that will be updated at each step.
For example, the following config defines a schedule that linearly decays the learning rate from 1.0 to 0.1 over 1000 steps:
Config(
lr: Schedule(
key: "state.step",
schedule: [
(0, 1.0),
"lin",
(1000, 0.1),
],
),
batch_size: 256,
)
When you call step()
, all config values that are schedules will be updated.
HyperState is dual-licensed under the MIT license and Apache License (Version 2.0).
See LICENSE-MIT and LICENSE-APACHE for more information.
FAQs
Library for managing hyperparameters and mutable state of machine learning training systems.
We found that hyperstate demonstrated a healthy version release cadence and project activity because the last version was released less than a year ago. It has 1 open source maintainer collaborating on the project.
Did you know?
Socket for GitHub automatically highlights issues in each pull request and monitors the health of all your open source dependencies. Discover the contents of your packages and block harmful activity before you install or update your dependencies.
Security News
NVD now marks all pre-2018 CVEs as "Deferred," signaling it will no longer enrich older vulnerabilities, further eroding trust in its data.
Research
Security News
Lazarus-linked threat actors expand their npm malware campaign with new RAT loaders, hex obfuscation, and over 5,600 downloads across 11 packages.
Security News
Safari 18.4 adds support for Iterator Helpers and two other TC39 JavaScript features, bringing full cross-browser coverage to key parts of the ECMAScript spec.