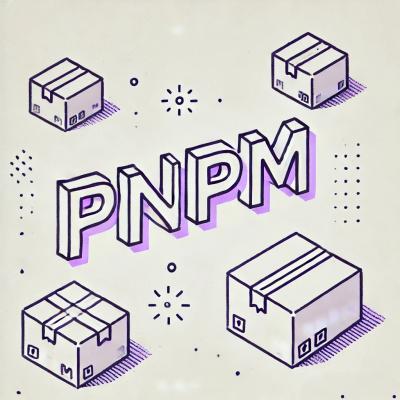
Security News
pnpm 10.12 Introduces Global Virtual Store and Expanded Version Catalogs
pnpm 10.12.1 introduces a global virtual store for faster installs and new options for managing dependencies with version catalogs.
Paper | Introduction | Implemented Solvers | Features | Installation | Contributing
JaxSGMC brings Stochastic Gradient Markov chain Monte Carlo (SGMCMC) samplers to JAX. Inspired by optax, JaxSGMC is built on a modular concept to increase reusability and accelerate research of new SGMCMC solvers. Additionally, JaxSGMC aims to promote probabilistic machine learning by removing obstacles in switching from stochastic optimizers to SGMCMC samplers.
alias.py
To get started quickly using SGMCMC samplers, JaxSGMC provides some popular pre-built samplers in alias.py:
JaxSGMC aims to increase reusability of SGMCMC components via a toolbox of helper functions and a modular concept:
In the simplest case of employing a pre-built sampler from alias.py, the user only needs to provide the computational model, consisting of functions for Prior and Likelihood. Schedulers allow to change sampler properies over the course of the training. Advanced users may build custom samplers from given components.
jit
JaxSGMC provides a toolbox to pass reference data to the computation and save collected samples from the Markov chain.
By combining different data loader / collector classes and general wrappers it is possible to read data from and save samples to different data types via the mechanisms of JAX's Host-Callback module. It is therefore also possible to access datasets bigger than the device memory.
Saving Data:
.npz
Loading Data:
Stochastic Gradient MCMC requires the evaluation of a potential function for a batch of data. JaxSGMC allows to compute this potential from likelihoods accepting only single observations and batches them automatically with sequential, parallel or vectorized execution. Moreover, JaxSGMC supports passing a model state between the evaluations of the likelihood function, which is saved corresponding to the samples, speeding up postprocessing.
JaxSGMC can be installed via pip:
pip install jax-sgmc --upgrade
The above command installs Jax for CPU. To run JaxSGMC on the GPU, the GPU version of JAX has to be installed. Further information can be found here: Jax Installation Instructions
Some parts of JaxSGMC require additional packages:
pip install jax-sgmc[tensorflow] --upgrade
pip install jax-sgmc[hdf5] --upgrade
For development purposes, JaxSGMC can be installed from source in editable mode:
git clone git@github.com:tummfm/jax-sgmc.git
pip install -e .[test,docs]
This command additionally installs the requirements to run the tests:
pytest tests
And to build the documentation (e.g. in html):
make -C docs html
Contributions are always welcome! Please open a pull request to discuss the code additions.
If you use JaxSGMC in your own work, please consider citing
@article{jaxsgmc2024,
title = {JaxSGMC: Modular stochastic gradient MCMC in JAX},
journal = {SoftwareX},
volume = {26},
pages = {101722},
year = {2024},
issn = {2352-7110},
doi = {https://doi.org/10.1016/j.softx.2024.101722},
url = {https://www.sciencedirect.com/science/article/pii/S2352711024000931},
author = {Stephan Thaler and Paul Fuchs and Ana Cukarska and Julija Zavadlav},
}
FAQs
Stochastic Gradient Monte Carlo in Jax
We found that jax-sgmc demonstrated a healthy version release cadence and project activity because the last version was released less than a year ago. It has 1 open source maintainer collaborating on the project.
Did you know?
Socket for GitHub automatically highlights issues in each pull request and monitors the health of all your open source dependencies. Discover the contents of your packages and block harmful activity before you install or update your dependencies.
Security News
pnpm 10.12.1 introduces a global virtual store for faster installs and new options for managing dependencies with version catalogs.
Security News
Amaro 1.0 lays the groundwork for stable TypeScript support in Node.js, bringing official .ts loading closer to reality.
Research
A deceptive PyPI package posing as an Instagram growth tool collects user credentials and sends them to third-party bot services.