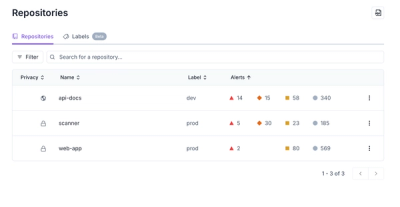
Product
Redesigned Repositories Page: A Faster Way to Prioritize Security Risk
Our redesigned Repositories page adds alert severity, filtering, and tabs for faster triage and clearer insights across all your projects.
A Python library for estimating kidney failure risk using the KFRE model developed by Tangri et al.
kfre
is a Python library designed to estimate the risk of chronic kidney disease (CKD) progression using the Kidney Failure Risk Equation (KFRE) developed by Tangri et al. It provides risk assessments over two distinct timelines: 2 years and 5 years. The library is tailored for healthcare professionals and researchers, enabling precise CKD risk predictions based on patient data. It supports predictions for both males and females and includes adjustments for individuals from North American and non-North American regions.
Before you install kfre
, ensure you have the following:
kfre
.Additionally, kfre has the following package dependencies:
You can install kfre
directly from PyPI:
pip install kfre
https://lshpaner.github.io/kfre
kfre
is distributed under the MIT License. See LICENSE for more information.
kfre
If you use kfre
in your research or projects, please consider citing it.
@software{shpaner_2024_11100222,
author = {Shpaner, Leonid},
title = {{kfre: A Python Library for Reproducing Kidney
Failure Risk Equations (KFRE)}},
month = may,
year = 2024,
publisher = {Zenodo},
version = {0.1.12},
doi = {10.5281/zenodo.11100222},
url = {https://doi.org/10.5281/zenodo.11100222}
}
If you have any questions or issues with kfre
, please open an issue on this GitHub repository.
The KFRE model developed by Tangri et al. has made significant contributions to kidney disease research.
The kfre
library is based on the risk prediction models developed in the studies referenced below. Please refer to these studies for an in-depth understanding of the kidney failure risk prediction models used within this library.
Special thanks to Panayiotis Petousis, PhD, Obidiugwu Duru, MD, MS, Kenn B. Daratha, PhD, Keith C. Norris, MD, PhD, Katherine R. Tuttle MD, FASN, FACP, FNKF, Susanne B. Nicholas, MD, MPH, PhD, and Alex Bui, PhD. Their exceptional work on end-stage kidney disease has greatly inspired the creation of this library.
Sumida, K., Nadkarni, G. N., Grams, M. E., Sang, Y., Ballew, S. H., Coresh, J., Matsushita, K., Surapaneni, A., Brunskill, N., Chadban, S. J., Chang, A. R., Cirillo, M., Daratha, K. B., Gansevoort, R. T., Garg, A. X., Iacoviello, L., Kayama, T., Konta, T., Kovesdy, C. P., Lash, J., Lee, B. J., Major, R. W., Metzger, M., Miura, K., Naimark, D. M. J., Nelson, R. G., Sawhney, S., Stempniewicz, N., Tang, M., Townsend, R. R., Traynor, J. P., Valdivielso, J. M., Wetzels, J., Polkinghorne, K. R., & Heerspink, H. J. L. (2020). Conversion of urine protein-creatinine ratio or urine dipstick protein to urine albumin-creatinine ratio for use in chronic kidney disease screening and prognosis. Annals of Internal Medicine, 173(6), 426-435. https://doi.org/10.7326/M20-0529
Tangri, N., Grams, M. E., Levey, A. S., Coresh, J., Appel, L. J., Astor, B. C., Chodick, G., Collins, A. J., Djurdjev, O., Elley, C. R., Evans, M., Garg, A. X., Hallan, S. I., Inker, L. A., Ito, S., Jee, S. H., Kovesdy, C. P., Kronenberg, F., Heerspink, H. J. L., Marks, A., Nadkarni, G. N., Navaneethan, S. D., Nelson, R. G., Titze, S., Sarnak, M. J., Stengel, B., Woodward, M., Iseki, K., & for the CKD Prognosis Consortium. (2016). Multinational assessment of accuracy of equations for predicting risk of kidney failure: A meta-analysis. JAMA, 315(2), 164–174. https://doi.org/10.1001/jama.2015.18202
Tangri, N., Stevens, L. A., Griffith, J., Tighiouart, H., Djurdjev, O., Naimark, D., Levin, A., & Levey, A. S. (2011). A predictive model for progression of chronic kidney disease to kidney failure. JAMA, 305(15), 1553-1559. https://doi.org/10.1001/jama.2011.451
FAQs
A Python library for estimating kidney failure risk using the KFRE model developed by Tangri et al.
We found that kfre demonstrated a healthy version release cadence and project activity because the last version was released less than a year ago. It has 1 open source maintainer collaborating on the project.
Did you know?
Socket for GitHub automatically highlights issues in each pull request and monitors the health of all your open source dependencies. Discover the contents of your packages and block harmful activity before you install or update your dependencies.
Product
Our redesigned Repositories page adds alert severity, filtering, and tabs for faster triage and clearer insights across all your projects.
Security News
Slopsquatting is a new supply chain threat where AI-assisted code generators recommend hallucinated packages that attackers register and weaponize.
Security News
Multiple deserialization flaws in PyTorch Lightning could allow remote code execution when loading untrusted model files, affecting versions up to 2.4.0.