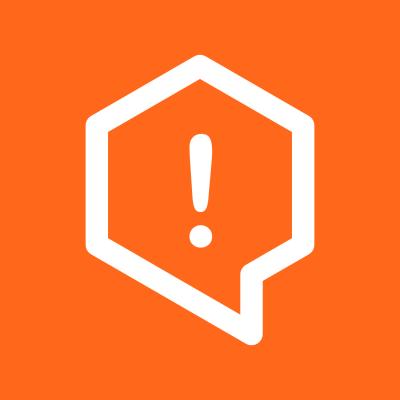
Security News
Fluent Assertions Faces Backlash After Abandoning Open Source Licensing
Fluent Assertions is facing backlash after dropping the Apache license for a commercial model, leaving users blindsided and questioning contributor rights.
mlflow-export-import
Advanced tools
Copy MLflow objects (experiments, runs or registered models) to another tracking server
The MLflow Export Import package provides tools to copy MLflow objects (runs, experiments or registered models) from one MLflow tracking server (Databricks workspace) to another. Using the MLflow REST API, the tools export MLflow objects to an intermediate directory and then import them into the target tracking server.
For more details:
Source tracking server | Destination tracking server | Note |
---|---|---|
Open source | Open source | common |
Open source | Databricks | less common |
Databricks | Databricks | common |
Databricks | Open source | rare |
These are the MLflow objects and their attributes that can be exported.
Object | REST | Python | SQL |
---|---|---|---|
Run | link | link | link |
Experiment | link | link | link |
Registered Model | link | link | link |
Registered Model Version | link | link | link |
MLflow provides rudimentary capabilities for tracking lineage regarding the original source objects. See README_governance.
There are two dimensions to the MLflow Export Import tools:
Single and Bulk Tools
The two export modes are:
Single tools. Copy a single MLflow object between tracking servers.
These tools allow you to specify a different destination object name.
For example, if you want to clone the experiment /Mary/Experiments/Iris
under a new name, you can specify the target experiment name as /John/Experiments/Iris
.
Bulk tools. High-level tools to copy an entire tracking server or a collection of MLflow objects. There is no option to change destination object names. Full object referential integrity is maintained (e.g. an imported registered model version will point to the imported run that it refers to.
Databricks notebooks simply invoke the corresponding Python classes.
Setup
pip install mlflow-export-import
Export experiment
export MLFLOW_TRACKING_URI=http://localhost:5000
export-experiment \
--experiment sklearn-wine \
--output-dir /tmp/export
Import experiment
export MLFLOW_TRACKING_URI=http://localhost:5001
import-experiment \
--experiment-name sklearn-wine \
--input-dir /tmp/export
Supports python 3.8.
First create a virtual environment.
python -m venv mlflow-export-import
source mlflow-export-import/bin/activate
There are several different ways to install the package.
pip install mlflow-export-import
pip install git+https:///github.com/mlflow/mlflow-export-import/#egg=mlflow-export-import
git clone https://github.com/mlflow/mlflow-export-import
cd mlflow-export-import
pip install -e .
There are two different ways to install the package in a Databricks notebook.
See documentation: Install notebook-scoped libraries with %pip.
%pip install mlflow-export-import
Build the wheel artifact, upload it to DBFS and then install it on your cluster.
git clone https://github.com/mlflow/mlflow-export-import
cd mlflow-export-import
python setup.py bdist_wheel
databricks fs cp dist/mlflow_export_import-1.0.0-py3-none-any.whl {MY_DBFS_PATH}
To run the tools externally (from your laptop) against a Databricks tracking server (workspace) set the following environment variables:
export MLFLOW_TRACKING_URI=databricks
export DATABRICKS_HOST=https://mycompany.cloud.databricks.com
export DATABRICKS_TOKEN=MY_TOKEN
For full details see Access the MLflow tracking server from outside Databricks.
The main tool scripts can be executed either as a standard Python script or console script.
Python console scripts (such as export-run, import-run, etc.) are provided as a convenience. For a list of scripts see setup.py.
This allows you to use:
export-experiment --help
instead of:
python -u -m mlflow_export_import.experiment.export_experiment --help
There are two types of tests : open source and Databricks tests. See tests/README for details.
FAQs
Copy MLflow objects (experiments, runs or registered models) to another tracking server
We found that mlflow-export-import demonstrated a healthy version release cadence and project activity because the last version was released less than a year ago. It has 1 open source maintainer collaborating on the project.
Did you know?
Socket for GitHub automatically highlights issues in each pull request and monitors the health of all your open source dependencies. Discover the contents of your packages and block harmful activity before you install or update your dependencies.
Security News
Fluent Assertions is facing backlash after dropping the Apache license for a commercial model, leaving users blindsided and questioning contributor rights.
Research
Security News
Socket researchers uncover the risks of a malicious Python package targeting Discord developers.
Security News
The UK is proposing a bold ban on ransomware payments by public entities to disrupt cybercrime, protect critical services, and lead global cybersecurity efforts.