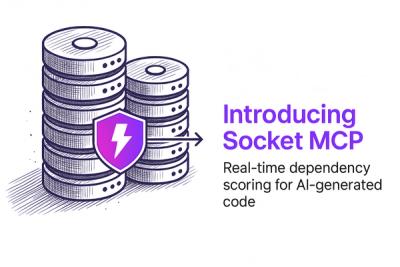
Product
Secure Your AI-Generated Code with Socket MCP
Socket MCP brings real-time security checks to AI-generated code, helping developers catch risky dependencies before they enter the codebase.
Gone are the days of black-box dataframes in otherwise type-safe code! Pandantic builds off the Pydantic API to enable validation and filtering of the usual dataframe types (i.e., pandas, etc.)
pandantic
introduces the ability to validate (pandas
) DataFrames using the pydantic.BaseModel
. The package is still in development and wants to focus on more dataframe types in the future (like polars
and spark
) besides pandas
. Currently, only the pandas
type is supported together with a pandas
plugin.
First, install pandantic
by using pip (or any other package managing tool).
pip install pandantic
Documentation can be found here
from pydantic import BaseModel
from pydantic.types import StrictInt
from pandantic import Pandantic
# Define your schema using Pydantic BaseModel
class DataFrameSchema(BaseModel):
"""Example schema for testing."""
example_str: str
example_int: StrictInt
# Create a validator instance
validator = Pandantic(schema=DataFrameSchema)
# Example DataFrame with some invalid data
df_invalid = pd.DataFrame(
data={
"example_str": ["foo", "bar", 1], # Last value is invalid (int instead of str)
"example_int": ["1", 2, 3.0], # First and last values are invalid (str and float)
}
)
# Validate with error raising
try:
validator.validate(dataframe=df_invalid, errors="raise")
except ValueError:
print("Validation failed!")
# Or filter out invalid rows
df_valid = validator.validate(dataframe=df_invalid, errors="skip")
# Only the second row remains as it's the only valid one
The validator supports two modes:
errors="raise"
: Raises a ValueError if any row fails validationerrors="skip"
: Returns a new DataFrame with only the valid rowsAnother way to use pandantic
is via our pandas.DataFrame
extension plugin. This adds the following methods to pandas
(once "registered" by import pandantic.plugins.pandas
):
DataFrame.pandantic.validate(schema:PandanticBaseModel)
, which returns a boolean for all valid inputs.DataFrame.pandantic.filter(schema:PandanticBaseModel)
, which wraps PandanticBaseModel.parse_obj(errors="filter")
and returns as dataframe.Example:
import pandas as pd
from pydantic import BaseModel
import pandantic.plugins.pandas
df1: pd.DataFrame = pd.DataFrame({"a": [1, 2, 3], "b": ["a", "b", "c"]})
class MyModel(BaseModel):
a: int
b: str
df1.pandantic.validate(MyModel) # returns True
df1.pandantic.filter(MyModel) # returns the same dataframe
# but if we have a mixed DataFrame
df2: pd.DataFrame = pd.DataFrame({"a": [1, 2, "3"], "b": ["a", 3, "c"]})
df2.pandantic.validate(MyModel) # returns False
df2.pandantic.filter(MyModel) # returns the filtered DataFrame with only the first row
The validator supports Pydantic's strict types for more rigorous validation:
from pydantic import BaseModel
from pydantic.types import StrictInt
from pandantic import Pandantic
class StrictSchema(BaseModel):
example_str: str
example_int: StrictInt # Will only accept actual integers
validator = Pandantic(schema=StrictSchema)
df = pd.DataFrame({
"example_str": ["foo", "bar"],
"example_int": [1, "2"] # Second value will fail as it's a string
})
# This will only keep the first row
df_valid = validator.validate(dataframe=df, errors="skip")
You can still use all of Pydantic's validation features in your schema:
from pydantic import BaseModel, field_validator
from pandantic import Pandantic
class CustomSchema(BaseModel):
example_str: str
example_int: int
@field_validator("example_int")
def must_be_even(cls, v: int) -> int:
if v % 2 != 0:
raise ValueError("Number must be even")
return v
validator = Pandantic(schema=CustomSchema)
As the DataFrame is being parsed into a dict, a None
value is considered as a nan
value in cases there are different values in the dict. Therefore, specifying Optional
columns (where the value can be empty) can be speciyfied by using the custom pandantic.Optional
type. This type is a replacement for typing.Optional
.
from pydantic import BaseModel
from pandantic import Optional # pylint: disable=import-outside-toplevel
# GIVEN
class Model(BaseModel):
a: Optional[int] = None
b: int
df_example = pd.DataFrame({"a": [1, None, 2], "b": ["str", 2, 3]})
validator = Pandantic(schema=Model)
FAQs
Gone are the days of black-box dataframes in otherwise type-safe code! Pandantic builds off the Pydantic API to enable validation and filtering of the usual dataframe types (i.e., pandas, etc.)
We found that pandantic demonstrated a healthy version release cadence and project activity because the last version was released less than a year ago. It has 1 open source maintainer collaborating on the project.
Did you know?
Socket for GitHub automatically highlights issues in each pull request and monitors the health of all your open source dependencies. Discover the contents of your packages and block harmful activity before you install or update your dependencies.
Product
Socket MCP brings real-time security checks to AI-generated code, helping developers catch risky dependencies before they enter the codebase.
Security News
As vulnerability data bottlenecks grow, the federal government is formally investigating NIST’s handling of the National Vulnerability Database.
Research
Security News
Socket’s Threat Research Team has uncovered 60 npm packages using post-install scripts to silently exfiltrate hostnames, IP addresses, DNS servers, and user directories to a Discord-controlled endpoint.