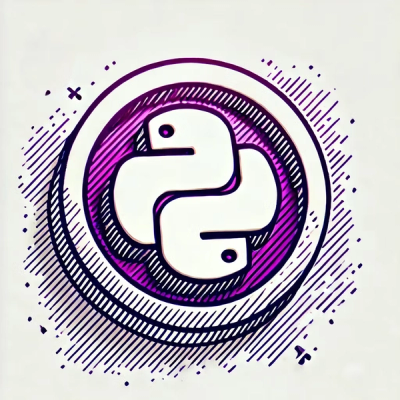
Security News
Python Tools Are Quickly Adopting the New pylock.toml Standard
pip, PDM, pip-audit, and the packaging library are already adding support for Python’s new lock file format.
pgvector support for Python
Supports Django, SQLAlchemy, SQLModel, Psycopg 3, Psycopg 2, asyncpg, pg8000, and Peewee
Run:
pip install pgvector
And follow the instructions for your database library:
Or check out some examples:
COPY
Create a migration to enable the extension
from pgvector.django import VectorExtension
class Migration(migrations.Migration):
operations = [
VectorExtension()
]
Add a vector field to your model
from pgvector.django import VectorField
class Item(models.Model):
embedding = VectorField(dimensions=3)
Also supports HalfVectorField
, BitField
, and SparseVectorField
Insert a vector
item = Item(embedding=[1, 2, 3])
item.save()
Get the nearest neighbors to a vector
from pgvector.django import L2Distance
Item.objects.order_by(L2Distance('embedding', [3, 1, 2]))[:5]
Also supports MaxInnerProduct
, CosineDistance
, L1Distance
, HammingDistance
, and JaccardDistance
Get the distance
Item.objects.annotate(distance=L2Distance('embedding', [3, 1, 2]))
Get items within a certain distance
Item.objects.alias(distance=L2Distance('embedding', [3, 1, 2])).filter(distance__lt=5)
Average vectors
from django.db.models import Avg
Item.objects.aggregate(Avg('embedding'))
Also supports Sum
Add an approximate index
from pgvector.django import HnswIndex, IvfflatIndex
class Item(models.Model):
class Meta:
indexes = [
HnswIndex(
name='my_index',
fields=['embedding'],
m=16,
ef_construction=64,
opclasses=['vector_l2_ops']
),
# or
IvfflatIndex(
name='my_index',
fields=['embedding'],
lists=100,
opclasses=['vector_l2_ops']
)
]
Use vector_ip_ops
for inner product and vector_cosine_ops
for cosine distance
Index vectors at half-precision
from django.contrib.postgres.indexes import OpClass
from django.db.models.functions import Cast
from pgvector.django import HnswIndex, HalfVectorField
class Item(models.Model):
class Meta:
indexes = [
HnswIndex(
OpClass(Cast('embedding', HalfVectorField(dimensions=3)), name='halfvec_l2_ops'),
name='my_index',
m=16,
ef_construction=64
)
]
Note: Add 'django.contrib.postgres'
to INSTALLED_APPS
to use OpClass
Get the nearest neighbors
distance = L2Distance(Cast('embedding', HalfVectorField(dimensions=3)), [3, 1, 2])
Item.objects.order_by(distance)[:5]
Enable the extension
session.execute(text('CREATE EXTENSION IF NOT EXISTS vector'))
Add a vector column
from pgvector.sqlalchemy import Vector
class Item(Base):
embedding = mapped_column(Vector(3))
Also supports HALFVEC
, BIT
, and SPARSEVEC
Insert a vector
item = Item(embedding=[1, 2, 3])
session.add(item)
session.commit()
Get the nearest neighbors to a vector
session.scalars(select(Item).order_by(Item.embedding.l2_distance([3, 1, 2])).limit(5))
Also supports max_inner_product
, cosine_distance
, l1_distance
, hamming_distance
, and jaccard_distance
Get the distance
session.scalars(select(Item.embedding.l2_distance([3, 1, 2])))
Get items within a certain distance
session.scalars(select(Item).filter(Item.embedding.l2_distance([3, 1, 2]) < 5))
Average vectors
from pgvector.sqlalchemy import avg
session.scalars(select(avg(Item.embedding))).first()
Also supports sum
Add an approximate index
index = Index(
'my_index',
Item.embedding,
postgresql_using='hnsw',
postgresql_with={'m': 16, 'ef_construction': 64},
postgresql_ops={'embedding': 'vector_l2_ops'}
)
# or
index = Index(
'my_index',
Item.embedding,
postgresql_using='ivfflat',
postgresql_with={'lists': 100},
postgresql_ops={'embedding': 'vector_l2_ops'}
)
index.create(engine)
Use vector_ip_ops
for inner product and vector_cosine_ops
for cosine distance
Index vectors at half-precision
from pgvector.sqlalchemy import HALFVEC
from sqlalchemy.sql import func
index = Index(
'my_index',
func.cast(Item.embedding, HALFVEC(3)).label('embedding'),
postgresql_using='hnsw',
postgresql_with={'m': 16, 'ef_construction': 64},
postgresql_ops={'embedding': 'halfvec_l2_ops'}
)
Get the nearest neighbors
order = func.cast(Item.embedding, HALFVEC(3)).l2_distance([3, 1, 2])
session.scalars(select(Item).order_by(order).limit(5))
Add an array column
from pgvector.sqlalchemy import Vector
from sqlalchemy import ARRAY
class Item(Base):
embeddings = mapped_column(ARRAY(Vector(3)))
And register the types with the underlying driver
For Psycopg 3, use
from pgvector.psycopg import register_vector
from sqlalchemy import event
@event.listens_for(engine, "connect")
def connect(dbapi_connection, connection_record):
register_vector(dbapi_connection)
For async connections with Psycopg 3, use
from pgvector.psycopg import register_vector_async
from sqlalchemy import event
@event.listens_for(engine.sync_engine, "connect")
def connect(dbapi_connection, connection_record):
dbapi_connection.run_async(register_vector_async)
For Psycopg 2, use
from pgvector.psycopg2 import register_vector
from sqlalchemy import event
@event.listens_for(engine, "connect")
def connect(dbapi_connection, connection_record):
register_vector(dbapi_connection, arrays=True)
Enable the extension
session.exec(text('CREATE EXTENSION IF NOT EXISTS vector'))
Add a vector column
from pgvector.sqlalchemy import Vector
class Item(SQLModel, table=True):
embedding: Any = Field(sa_type=Vector(3))
Also supports HALFVEC
, BIT
, and SPARSEVEC
Insert a vector
item = Item(embedding=[1, 2, 3])
session.add(item)
session.commit()
Get the nearest neighbors to a vector
session.exec(select(Item).order_by(Item.embedding.l2_distance([3, 1, 2])).limit(5))
Also supports max_inner_product
, cosine_distance
, l1_distance
, hamming_distance
, and jaccard_distance
Get the distance
session.exec(select(Item.embedding.l2_distance([3, 1, 2])))
Get items within a certain distance
session.exec(select(Item).filter(Item.embedding.l2_distance([3, 1, 2]) < 5))
Average vectors
from pgvector.sqlalchemy import avg
session.exec(select(avg(Item.embedding))).first()
Also supports sum
Add an approximate index
from sqlmodel import Index
index = Index(
'my_index',
Item.embedding,
postgresql_using='hnsw',
postgresql_with={'m': 16, 'ef_construction': 64},
postgresql_ops={'embedding': 'vector_l2_ops'}
)
# or
index = Index(
'my_index',
Item.embedding,
postgresql_using='ivfflat',
postgresql_with={'lists': 100},
postgresql_ops={'embedding': 'vector_l2_ops'}
)
index.create(engine)
Use vector_ip_ops
for inner product and vector_cosine_ops
for cosine distance
Enable the extension
conn.execute('CREATE EXTENSION IF NOT EXISTS vector')
Register the vector type with your connection
from pgvector.psycopg import register_vector
register_vector(conn)
For connection pools, use
def configure(conn):
register_vector(conn)
pool = ConnectionPool(..., configure=configure)
For async connections, use
from pgvector.psycopg import register_vector_async
await register_vector_async(conn)
Create a table
conn.execute('CREATE TABLE items (id bigserial PRIMARY KEY, embedding vector(3))')
Insert a vector
embedding = np.array([1, 2, 3])
conn.execute('INSERT INTO items (embedding) VALUES (%s)', (embedding,))
Get the nearest neighbors to a vector
conn.execute('SELECT * FROM items ORDER BY embedding <-> %s LIMIT 5', (embedding,)).fetchall()
Add an approximate index
conn.execute('CREATE INDEX ON items USING hnsw (embedding vector_l2_ops)')
# or
conn.execute('CREATE INDEX ON items USING ivfflat (embedding vector_l2_ops) WITH (lists = 100)')
Use vector_ip_ops
for inner product and vector_cosine_ops
for cosine distance
Enable the extension
cur = conn.cursor()
cur.execute('CREATE EXTENSION IF NOT EXISTS vector')
Register the vector type with your connection or cursor
from pgvector.psycopg2 import register_vector
register_vector(conn)
Create a table
cur.execute('CREATE TABLE items (id bigserial PRIMARY KEY, embedding vector(3))')
Insert a vector
embedding = np.array([1, 2, 3])
cur.execute('INSERT INTO items (embedding) VALUES (%s)', (embedding,))
Get the nearest neighbors to a vector
cur.execute('SELECT * FROM items ORDER BY embedding <-> %s LIMIT 5', (embedding,))
cur.fetchall()
Add an approximate index
cur.execute('CREATE INDEX ON items USING hnsw (embedding vector_l2_ops)')
# or
cur.execute('CREATE INDEX ON items USING ivfflat (embedding vector_l2_ops) WITH (lists = 100)')
Use vector_ip_ops
for inner product and vector_cosine_ops
for cosine distance
Enable the extension
await conn.execute('CREATE EXTENSION IF NOT EXISTS vector')
Register the vector type with your connection
from pgvector.asyncpg import register_vector
await register_vector(conn)
or your pool
async def init(conn):
await register_vector(conn)
pool = await asyncpg.create_pool(..., init=init)
Create a table
await conn.execute('CREATE TABLE items (id bigserial PRIMARY KEY, embedding vector(3))')
Insert a vector
embedding = np.array([1, 2, 3])
await conn.execute('INSERT INTO items (embedding) VALUES ($1)', embedding)
Get the nearest neighbors to a vector
await conn.fetch('SELECT * FROM items ORDER BY embedding <-> $1 LIMIT 5', embedding)
Add an approximate index
await conn.execute('CREATE INDEX ON items USING hnsw (embedding vector_l2_ops)')
# or
await conn.execute('CREATE INDEX ON items USING ivfflat (embedding vector_l2_ops) WITH (lists = 100)')
Use vector_ip_ops
for inner product and vector_cosine_ops
for cosine distance
Enable the extension
conn.run('CREATE EXTENSION IF NOT EXISTS vector')
Register the vector type with your connection
from pgvector.pg8000 import register_vector
register_vector(conn)
Create a table
conn.run('CREATE TABLE items (id bigserial PRIMARY KEY, embedding vector(3))')
Insert a vector
embedding = np.array([1, 2, 3])
conn.run('INSERT INTO items (embedding) VALUES (:embedding)', embedding=embedding)
Get the nearest neighbors to a vector
conn.run('SELECT * FROM items ORDER BY embedding <-> :embedding LIMIT 5', embedding=embedding)
Add an approximate index
conn.run('CREATE INDEX ON items USING hnsw (embedding vector_l2_ops)')
# or
conn.run('CREATE INDEX ON items USING ivfflat (embedding vector_l2_ops) WITH (lists = 100)')
Use vector_ip_ops
for inner product and vector_cosine_ops
for cosine distance
Add a vector column
from pgvector.peewee import VectorField
class Item(BaseModel):
embedding = VectorField(dimensions=3)
Also supports HalfVectorField
, FixedBitField
, and SparseVectorField
Insert a vector
item = Item.create(embedding=[1, 2, 3])
Get the nearest neighbors to a vector
Item.select().order_by(Item.embedding.l2_distance([3, 1, 2])).limit(5)
Also supports max_inner_product
, cosine_distance
, l1_distance
, hamming_distance
, and jaccard_distance
Get the distance
Item.select(Item.embedding.l2_distance([3, 1, 2]).alias('distance'))
Get items within a certain distance
Item.select().where(Item.embedding.l2_distance([3, 1, 2]) < 5)
Average vectors
from peewee import fn
Item.select(fn.avg(Item.embedding).coerce(True)).scalar()
Also supports sum
Add an approximate index
Item.add_index('embedding vector_l2_ops', using='hnsw')
Use vector_ip_ops
for inner product and vector_cosine_ops
for cosine distance
Create a half vector from a list
vec = HalfVector([1, 2, 3])
Or a NumPy array
vec = HalfVector(np.array([1, 2, 3]))
Get a list
lst = vec.to_list()
Get a NumPy array
arr = vec.to_numpy()
Create a sparse vector from a list
vec = SparseVector([1, 0, 2, 0, 3, 0])
Or a NumPy array
vec = SparseVector(np.array([1, 0, 2, 0, 3, 0]))
Or a SciPy sparse array
arr = coo_array(([1, 2, 3], ([0, 2, 4],)), shape=(6,))
vec = SparseVector(arr)
Or a dictionary of non-zero elements
vec = SparseVector({0: 1, 2: 2, 4: 3}, 6)
Note: Indices start at 0
Get the number of dimensions
dim = vec.dimensions()
Get the indices of non-zero elements
indices = vec.indices()
Get the values of non-zero elements
values = vec.values()
Get a list
lst = vec.to_list()
Get a NumPy array
arr = vec.to_numpy()
Get a SciPy sparse array
arr = vec.to_coo()
View the changelog
Everyone is encouraged to help improve this project. Here are a few ways you can help:
To get started with development:
git clone https://github.com/pgvector/pgvector-python.git
cd pgvector-python
pip install -r requirements.txt
createdb pgvector_python_test
pytest
To run an example:
cd examples/loading
pip install -r requirements.txt
createdb pgvector_example
python3 example.py
FAQs
pgvector support for Python
We found that pgvector demonstrated a healthy version release cadence and project activity because the last version was released less than a year ago. It has 1 open source maintainer collaborating on the project.
Did you know?
Socket for GitHub automatically highlights issues in each pull request and monitors the health of all your open source dependencies. Discover the contents of your packages and block harmful activity before you install or update your dependencies.
Security News
pip, PDM, pip-audit, and the packaging library are already adding support for Python’s new lock file format.
Product
Socket's Go support is now generally available, bringing automatic scanning and deep code analysis to all users with Go projects.
Security News
vlt adds real-time security selectors powered by Socket, enabling developers to query and analyze package risks directly in their dependency graph.