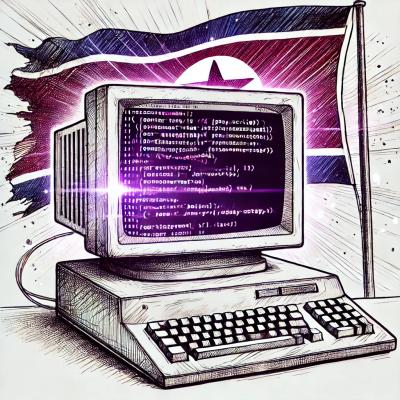
Research
Security News
Lazarus Strikes npm Again with New Wave of Malicious Packages
The Socket Research Team has discovered six new malicious npm packages linked to North Koreaβs Lazarus Group, designed to steal credentials and deploy backdoors.
Find the best probability distribution for your dataset and simulate processes and queues
Phitter analyzes datasets and determines the best analytical probability distributions that represent them. Phitter studies over 80 probability distributions, both continuous and discrete, 3 goodness-of-fit tests, and interactive visualizations. For each selected probability distribution, a standard modeling guide is provided along with spreadsheets that detail the methodology for using the chosen distribution in data science, operations research, and artificial intelligence.
In addition, Phitter offers the capability to perform process simulations, allowing users to graph and observe minimum times for specific observations. It also supports queue simulations with flexibility to configure various parameters, such as the number of servers, maximum population size, system capacity, and different queue disciplines, including First-In-First-Out (FIFO), Last-In-First-Out (LIFO), and priority-based service (PBS).
This repository contains the implementation of the python library and the kernel of Phitter Web
python: >=3.9
pip install phitter
Tutorial | Notebooks |
---|---|
Fit Continuous | |
Fit Discrete | |
Fit Accelerate [Sample>100K] | |
Fit Specific Disribution | |
Working Distribution |
Pending
import phitter
## Define your dataset
data: list[int | float] = [...]
## Make a continuous fit using Phitter
phi = phitter.PHITTER(data)
phi.fit()
import phitter
## Define your dataset
data: list[int | float] = [...]
## Make a continuous fit using Phitter
phi = phitter.PHITTER(
data=data,
fit_type="continuous",
num_bins=15,
confidence_level=0.95,
minimum_sse=1e-2,
distributions_to_fit=["beta", "normal", "fatigue_life", "triangular"],
)
phi.fit(n_workers=6)
import phitter
## Define your dataset
data: list[int | float] = [...]
## Make a discrete fit using Phitter
phi = phitter.PHITTER(
data=data,
fit_type="discrete",
confidence_level=0.95,
minimum_sse=1e-2,
distributions_to_fit=["binomial", "geometric"],
)
phi.fit(n_workers=2)
import phitter
## Define your dataset
data: list[int | float] = [...]
## Make a fit using Phitter
phi = phitter.PHITTER(data)
phi.fit(n_workers=2)
## Global methods and properties
phi.summarize(k: int) -> pandas.DataFrame
phi.summarize_info(k: int) -> pandas.DataFrame
phi.best_distribution -> dict
phi.sorted_distributions_sse -> dict
phi.not_rejected_distributions -> dict
phi.df_sorted_distributions_sse -> pandas.DataFrame
phi.df_not_rejected_distributions -> pandas.DataFrame
## Specific distribution methods and properties
phi.get_parameters(id_distribution: str) -> dict
phi.get_test_chi_square(id_distribution: str) -> dict
phi.get_test_kolmmogorov_smirnov(id_distribution: str) -> dict
phi.get_test_anderson_darling(id_distribution: str) -> dict
phi.get_sse(id_distribution: str) -> float
phi.get_n_test_passed(id_distribution: str) -> int
phi.get_n_test_null(id_distribution: str) -> int
import phitter
data: list[int | float] = [...]
phi = phitter.PHITTER(data)
phi.fit()
phi.plot_histogram()
import phitter
data: list[int | float] = [...]
phi = phitter.PHITTER(data)
phi.fit()
phi.plot_histogram_distributions()
import phitter
data: list[int | float] = [...]
phi = phitter.PHITTER(data)
phi.fit()
phi.plot_distribution("beta")
import phitter
data: list[int | float] = [...]
phi = phitter.PHITTER(data)
phi.fit()
phi.plot_ecdf()
import phitter
data: list[int | float] = [...]
phi = phitter.PHITTER(data)
phi.fit()
phi.plot_ecdf_distribution("beta")
import phitter
data: list[int | float] = [...]
phi = phitter.PHITTER(data)
phi.fit()
phi.qq_plot("beta")
import phitter
data: list[int | float] = [...]
phi = phitter.PHITTER(data)
phi.fit()
phi.qq_plot_regression("beta")
import phitter
distribution = phitter.continuous.BETA({"alpha": 5, "beta": 3, "A": 200, "B": 1000})
## CDF, PDF, PPF, PMF receive float or numpy.ndarray. For discrete distributions PMF instead of PDF. Parameters notation are in description of ditribution
distribution.cdf(752) # -> 0.6242831129533498
distribution.pdf(388) # -> 0.0002342575686629883
distribution.ppf(0.623) # -> 751.5512889417921
distribution.sample(2) # -> [550.800114 514.85410326]
## STATS
distribution.mean # -> 700.0
distribution.variance # -> 16666.666666666668
distribution.standard_deviation # -> 129.09944487358058
distribution.skewness # -> -0.3098386676965934
distribution.kurtosis # -> 2.5854545454545454
distribution.median # -> 708.707130841534
distribution.mode # -> 733.3333333333333
Distribution | Phitter Playground | Excel File | Google Sheets Files |
---|---|---|---|
bernoulli | βΆοΈphitter:bernoulli | πbernoulli.xlsx | πgs:bernoulli |
binomial | βΆοΈphitter:binomial | πbinomial.xlsx | πgs:binomial |
geometric | βΆοΈphitter:geometric | πgeometric.xlsx | πgs:geometric |
hypergeometric | βΆοΈphitter:hypergeometric | πhypergeometric.xlsx | πgs:hypergeometric |
logarithmic | βΆοΈphitter:logarithmic | πlogarithmic.xlsx | πgs:logarithmic |
negative_binomial | βΆοΈphitter:negative_binomial | πnegative_binomial.xlsx | πgs:negative_binomial |
poisson | βΆοΈphitter:poisson | πpoisson.xlsx | πgs:poisson |
uniform | βΆοΈphitter:uniform | πuniform.xlsx | πgs:uniform |
Sample Size / Workers | 1 | 2 | 6 | 10 | 20 |
---|---|---|---|---|---|
1K | 8.2981 | 7.1242 | 8.9667 | 9.9287 | 16.2246 |
10K | 20.8711 | 14.2647 | 10.5612 | 11.6004 | 17.8562 |
100K | 152.6296 | 97.2359 | 57.7310 | 51.6182 | 53.2313 |
500K | 914.9291 | 640.8153 | 370.0323 | 267.4597 | 257.7534 |
1M | 1580.8501 | 972.3985 | 573.5429 | 496.5569 | 425.7809 |
Sample Size / Workers | 1 | 2 | 4 |
---|---|---|---|
1K | 0.1688 | 2.6402 | 2.8719 |
10K | 0.4462 | 2.4452 | 3.0471 |
100K | 4.5598 | 6.3246 | 7.5869 |
500K | 19.0172 | 21.8047 | 19.8420 |
1M | 39.8065 | 29.8360 | 30.2334 |
Distribution / Sample Size | 1K | 10K | 100K | 500K | 1M | 10M |
---|---|---|---|---|---|---|
alpha | 0.3345 | 0.4625 | 2.5933 | 18.3856 | 39.6533 | 362.2951 |
arcsine | 0.0000 | 0.0000 | 0.0000 | 0.0000 | 0.0000 | 0.0000 |
argus | 0.0559 | 0.2050 | 2.2472 | 13.3928 | 41.5198 | 362.2472 |
beta | 0.1880 | 0.1790 | 0.1940 | 0.2110 | 0.1800 | 0.3134 |
beta_prime | 0.1766 | 0.7506 | 7.6039 | 40.4264 | 85.0677 | 812.1323 |
beta_prime_4p | 0.0720 | 0.3630 | 3.9478 | 20.2703 | 40.2709 | 413.5239 |
bradford | 0.0110 | 0.0000 | 0.0000 | 0.0000 | 0.0000 | 0.0010 |
burr | 0.0733 | 0.6931 | 5.5425 | 36.7684 | 79.8269 | 668.2016 |
burr_4p | 0.1552 | 0.7981 | 8.4716 | 44.4549 | 87.7292 | 858.0035 |
cauchy | 0.0090 | 0.0160 | 0.1581 | 1.1052 | 2.1090 | 21.5244 |
chi_square | 0.0000 | 0.0000 | 0.0000 | 0.0000 | 0.0000 | 0.0000 |
chi_square_3p | 0.0000 | 0.0000 | 0.0000 | 0.0000 | 0.0000 | 0.0000 |
dagum | 0.3381 | 0.8278 | 9.6907 | 45.5855 | 98.6691 | 917.6713 |
dagum_4p | 0.3646 | 1.3307 | 13.3437 | 70.9462 | 140.9371 | 1396.3368 |
erlang | 0.0010 | 0.0000 | 0.0000 | 0.0000 | 0.0000 | 0.0000 |
erlang_3p | 0.0000 | 0.0000 | 0.0000 | 0.0000 | 0.0000 | 0.0000 |
error_function | 0.0000 | 0.0000 | 0.0000 | 0.0000 | 0.0000 | 0.0000 |
exponential | 0.0000 | 0.0000 | 0.0000 | 0.0000 | 0.0000 | 0.0000 |
exponential_2p | 0.0000 | 0.0000 | 0.0000 | 0.0000 | 0.0000 | 0.0000 |
f | 0.0592 | 0.2948 | 2.6920 | 18.9458 | 29.9547 | 402.2248 |
fatigue_life | 0.0352 | 0.1101 | 1.7085 | 9.0090 | 20.4702 | 186.9631 |
folded_normal | 0.0020 | 0.0020 | 0.0020 | 0.0022 | 0.0033 | 0.0040 |
frechet | 0.1313 | 0.4359 | 5.7031 | 39.4202 | 43.2469 | 671.3343 |
f_4p | 0.3269 | 0.7517 | 0.6183 | 0.6037 | 0.5809 | 0.2073 |
gamma | 0.0000 | 0.0000 | 0.0000 | 0.0000 | 0.0000 | 0.0000 |
gamma_3p | 0.0000 | 0.0000 | 0.0000 | 0.0000 | 0.0000 | 0.0000 |
generalized_extreme_value | 0.0833 | 0.2054 | 2.0337 | 10.3301 | 22.1340 | 243.3120 |
generalized_gamma | 0.0298 | 0.0178 | 0.0227 | 0.0236 | 0.0170 | 0.0241 |
generalized_gamma_4p | 0.0371 | 0.0116 | 0.0732 | 0.0725 | 0.0707 | 0.0730 |
generalized_logistic | 0.1040 | 0.1073 | 0.1037 | 0.0819 | 0.0989 | 0.0836 |
generalized_normal | 0.0154 | 0.0736 | 0.7367 | 2.4831 | 5.9752 | 55.2417 |
generalized_pareto | 0.3189 | 0.8978 | 8.9370 | 51.3813 | 101.6832 | 1015.2933 |
gibrat | 0.0328 | 0.0432 | 0.4287 | 2.7159 | 5.5721 | 54.1702 |
gumbel_left | 0.0000 | 0.0000 | 0.0000 | 0.0000 | 0.0010 | 0.0010 |
gumbel_right | 0.0000 | 0.0000 | 0.0000 | 0.0000 | 0.0000 | 0.0000 |
half_normal | 0.0010 | 0.0000 | 0.0000 | 0.0010 | 0.0000 | 0.0000 |
hyperbolic_secant | 0.0000 | 0.0000 | 0.0000 | 0.0000 | 0.0000 | 0.0000 |
inverse_gamma | 0.0308 | 0.0632 | 0.7233 | 5.0127 | 10.7885 | 99.1316 |
inverse_gamma_3p | 0.0787 | 0.1472 | 1.6513 | 11.1161 | 23.4587 | 227.6125 |
inverse_gaussian | 0.0000 | 0.0000 | 0.0000 | 0.0000 | 0.0000 | 0.0000 |
inverse_gaussian_3p | 0.0000 | 0.0000 | 0.0000 | 0.0000 | 0.0000 | 0.0000 |
johnson_sb | 0.2966 | 0.7466 | 4.0707 | 40.2028 | 56.2130 | 728.2447 |
johnson_su | 0.0070 | 0.0010 | 0.0010 | 0.0143 | 0.0010 | 0.0010 |
kumaraswamy | 0.0164 | 0.0120 | 0.0130 | 0.0123 | 0.0125 | 0.0150 |
laplace | 0.0000 | 0.0000 | 0.0000 | 0.0000 | 0.0000 | 0.0000 |
levy | 0.0100 | 0.0314 | 0.2296 | 1.1365 | 2.7211 | 26.4966 |
loggamma | 0.0085 | 0.0050 | 0.0050 | 0.0070 | 0.0062 | 0.0080 |
logistic | 0.0000 | 0.0000 | 0.0000 | 0.0000 | 0.0000 | 0.0000 |
loglogistic | 0.0000 | 0.0000 | 0.0000 | 0.0000 | 0.0000 | 0.0000 |
loglogistic_3p | 0.0000 | 0.0000 | 0.0000 | 0.0000 | 0.0000 | 0.0000 |
lognormal | 0.0000 | 0.0000 | 0.0000 | 0.0000 | 0.0010 | 0.0000 |
maxwell | 0.0000 | 0.0000 | 0.0000 | 0.0000 | 0.0000 | 0.0010 |
moyal | 0.0000 | 0.0000 | 0.0000 | 0.0000 | 0.0000 | 0.0000 |
nakagami | 0.0000 | 0.0030 | 0.0213 | 0.1215 | 0.2649 | 2.2457 |
non_central_chi_square | 0.0000 | 0.0000 | 0.0000 | 0.0000 | 0.0000 | 0.0000 |
non_central_f | 0.0190 | 0.0182 | 0.0210 | 0.0192 | 0.0190 | 0.0200 |
non_central_t_student | 0.0874 | 0.0822 | 0.0862 | 0.1314 | 0.2516 | 0.1781 |
normal | 0.0000 | 0.0000 | 0.0000 | 0.0000 | 0.0000 | 0.0000 |
pareto_first_kind | 0.0010 | 0.0030 | 0.0390 | 0.2494 | 0.5226 | 5.5246 |
pareto_second_kind | 0.0643 | 0.1522 | 1.1722 | 10.9871 | 23.6534 | 201.1626 |
pert | 0.0052 | 0.0030 | 0.0030 | 0.0040 | 0.0040 | 0.0092 |
power_function | 0.0075 | 0.0040 | 0.0040 | 0.0030 | 0.0040 | 0.0040 |
rayleigh | 0.0000 | 0.0000 | 0.0000 | 0.0000 | 0.0000 | 0.0000 |
reciprocal | 0.0000 | 0.0000 | 0.0000 | 0.0000 | 0.0000 | 0.0000 |
rice | 0.0182 | 0.0030 | 0.0040 | 0.0060 | 0.0030 | 0.0050 |
semicircular | 0.0000 | 0.0000 | 0.0000 | 0.0000 | 0.0000 | 0.0000 |
trapezoidal | 0.0083 | 0.0072 | 0.0073 | 0.0060 | 0.0070 | 0.0060 |
triangular | 0.0000 | 0.0000 | 0.0000 | 0.0000 | 0.0000 | 0.0000 |
t_student | 0.0000 | 0.0000 | 0.0000 | 0.0000 | 0.0000 | 0.0000 |
t_student_3p | 0.3892 | 1.1860 | 11.2759 | 71.1156 | 143.1939 | 1409.8578 |
uniform | 0.0000 | 0.0000 | 0.0000 | 0.0000 | 0.0000 | 0.0000 |
weibull | 0.0010 | 0.0000 | 0.0000 | 0.0000 | 0.0010 | 0.0010 |
weibull_3p | 0.0061 | 0.0040 | 0.0030 | 0.0040 | 0.0050 | 0.0050 |
Distribution / Sample Size | 1K | 10K | 100K | 500K | 1M | 10M |
---|---|---|---|---|---|---|
bernoulli | 0.0000 | 0.0000 | 0.0000 | 0.0000 | 0.0000 | 0.0000 |
binomial | 0.0000 | 0.0000 | 0.0000 | 0.0000 | 0.0000 | 0.0000 |
geometric | 0.0000 | 0.0000 | 0.0000 | 0.0000 | 0.0000 | 0.0000 |
hypergeometric | 0.0773 | 0.0061 | 0.0030 | 0.0020 | 0.0030 | 0.0051 |
logarithmic | 0.0210 | 0.0035 | 0.0171 | 0.0050 | 0.0030 | 0.0756 |
negative_binomial | 0.0293 | 0.0000 | 0.0000 | 0.0000 | 0.0000 | 0.0000 |
poisson | 0.0000 | 0.0000 | 0.0000 | 0.0000 | 0.0000 | 0.0000 |
uniform | 0.0000 | 0.0000 | 0.0000 | 0.0000 | 0.0000 | 0.0000 |
This will help you to understand your processes. To use it, run the following line
from phitter import simulation
# Create a simulation process instance
simulation = simulation.ProcessSimulation()
There are two ways to add processes to your simulation instance:
# Add a new process without preceding process
simulation.add_process(
prob_distribution="normal",
parameters={"mu": 5, "sigma": 2},
process_id="first_process",
number_of_products=10,
number_of_servers=3,
new_branch=True,
)
# Add a new process with preceding process
simulation.add_process(
prob_distribution="exponential",
parameters={"lambda": 4},
process_id="second_process",
previous_ids=["first_process"],
)
The order in which you add each process matters. You can add as many processes as you need.
# Add a new process without preceding process
simulation.add_process(
prob_distribution="normal",
parameters={"mu": 5, "sigma": 2},
process_id="first_process",
number_of_products=10,
number_of_servers=3,
new_branch=True,
)
# Add a new process with preceding process
simulation.add_process(
prob_distribution="exponential",
parameters={"lambda": 4},
process_id="second_process",
previous_ids=["first_process"],
)
# Add a new process with preceding process
simulation.add_process(
prob_distribution="gamma",
parameters={"alpha": 15, "beta": 3},
process_id="third_process",
previous_ids=["first_process"],
)
# Add a new process without preceding process
simulation.add_process(
prob_distribution="exponential",
parameters={"lambda": 4.3},
process_id="fourth_process",
new_branch=True,
)
# Add a new process with preceding process
simulation.add_process(
prob_distribution="beta",
parameters={"alpha": 1, "beta": 1, "A": 2, "B": 3},
process_id="fifth_process",
previous_ids=["second_process", "fourth_process"],
)
# Add a new process with preceding process
simulation.add_process(
prob_distribution="normal",
parameters={"mu": 15, "sigma": 2},
process_id="sixth_process",
previous_ids=["third_process", "fifth_process"],
)
You can visualize your processes to see if what you're trying to simulate is your actual process.
# Graph your process
simulation.process_graph()
You can simulate and have different simulation time values or you can create a confidence interval for your process
Simulate several scenarios of your complete process
# Run Simulation
simulation.run(number_of_simulations=100)
# After run
simulation: pandas.Dataframe
If you want to review average time and standard deviation by stage run this line of code
# Review simulation metrics
simulation.simulation_metrics() -> pandas.Dataframe
If you want to have a confidence interval for the simulation metrics, run the following line of code
# Confidence interval for Simulation metrics
simulation.run_confidence_interval(
confidence_level=0.99,
number_of_simulations=100,
replications=10,
) -> pandas.Dataframe
If you need to simulate queues run the following code:
from phitter import simulation
# Create a simulation process instance
simulation = simulation.QueueingSimulation(
a="exponential",
a_paramters={"lambda": 5},
s="exponential",
s_parameters={"lambda": 20},
c=3,
)
In this case we are going to simulate a (arrivals) with exponential distribution and s (service) as exponential distribution with c equals to 3 different servers.
By default Maximum Capacity k is infinity, total population n is infinity and the queue discipline d is FIFO. As we are not selecting d equals to "PBS" we don't have any information to add for pbs_distribution nor pbs_parameters
If you want to have the simulation results
# Run simulation
simulation = simulation.run(simulation_time = 2000)
simulation: pandas.Dataframe
If you want to see some metrics and probabilities from this simulation you should use::
# Calculate metrics
simulation.metrics_summary() -> pandas.Dataframe
# Calculate probabilities
number_probability_summary() -> pandas.Dataframe
If you want to have a confidence interval for your metrics and probabilities you should run the following line
# Calculate confidence interval for metrics and probabilities
probabilities, metrics = simulation.confidence_interval_metrics(
simulation_time=2000,
confidence_level=0.99,
replications=10,
)
probabilities -> pandas.Dataframe
metrics -> pandas.Dataframe
If you would like to contribute to the Phitter project, please create a pull request with your proposed changes or enhancements. All contributions are welcome!
FAQs
Find the best probability distribution for your dataset and simulate processes and queues
We found that phitter demonstrated a healthy version release cadence and project activity because the last version was released less than a year ago.Β It has 1 open source maintainer collaborating on the project.
Did you know?
Socket for GitHub automatically highlights issues in each pull request and monitors the health of all your open source dependencies. Discover the contents of your packages and block harmful activity before you install or update your dependencies.
Research
Security News
The Socket Research Team has discovered six new malicious npm packages linked to North Koreaβs Lazarus Group, designed to steal credentials and deploy backdoors.
Security News
Socket CEO Feross Aboukhadijeh discusses the open web, open source security, and how Socket tackles software supply chain attacks on The Pair Program podcast.
Security News
Opengrep continues building momentum with the alpha release of its Playground tool, demonstrating the project's rapid evolution just two months after its initial launch.