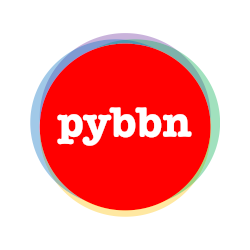
PyBBN
PyBBN is Python library for Bayesian Belief Networks (BBNs) exact inference using the junction tree algorithm or Probability Propagation in Trees of Clusters (PPTC). The implementation is taken directly from C. Huang and A. Darwiche, "Inference in
Belief Networks: A Procedural Guide," in International Journal of Approximate Reasoning, vol. 15,
pp. 225--263, 1999. In this API, PPTC is applied to BBNs with all discrete variables. When dealing with a BBN with all Gaussian variables (or a Gaussian Belief Network, GBN), exact inference is conducted through an incremental algorithm manipulating the means and covariance matrix. Additionally, there is the ability to generate singly- and multi-connected graphs, which is taken from JS Ide and FG Cozman,
"Random Generation of Bayesian Network," in Advances in Artificial Intelligence, Lecture Notes in Computer Science, vol 2507. There is also the option to generate sample data from your BBN. This synthetic data may be summarized to generate your posterior marginal probabilities and work as a form of approximate inference. Lastly, we have added Pearl's do-operator
for causal inference.
Power Up, Next Level
turing_bbn | pyspark-bbn |
---|
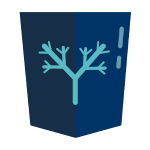 |  |
If you like py-bbn, please inquire about our next-generation products below! info@oneoffcoder.com
- turing_bbn is a C++17 implementation of py-bbn; take your causal and probabilistic inferences to the next computing level!
- pyspark-bbn is a is a scalable, massively parallel processing MPP framework for learning structures and parameters of Bayesian Belief Networks BBNs using Apache Spark.
Exact Inference, Discrete Variables
Below is an example code to create a Bayesian Belief Network, transform it into a join tree, and then set observation evidence. The last line prints the marginal probabilities for each node.
from pybbn.graph.dag import Bbn
from pybbn.graph.edge import Edge, EdgeType
from pybbn.graph.jointree import EvidenceBuilder
from pybbn.graph.node import BbnNode
from pybbn.graph.variable import Variable
from pybbn.pptc.inferencecontroller import InferenceController
a = BbnNode(Variable(0, 'a', ['on', 'off']), [0.5, 0.5])
b = BbnNode(Variable(1, 'b', ['on', 'off']), [0.5, 0.5, 0.4, 0.6])
c = BbnNode(Variable(2, 'c', ['on', 'off']), [0.7, 0.3, 0.2, 0.8])
d = BbnNode(Variable(3, 'd', ['on', 'off']), [0.9, 0.1, 0.5, 0.5])
e = BbnNode(Variable(4, 'e', ['on', 'off']), [0.3, 0.7, 0.6, 0.4])
f = BbnNode(Variable(5, 'f', ['on', 'off']), [0.01, 0.99, 0.01, 0.99, 0.01, 0.99, 0.99, 0.01])
g = BbnNode(Variable(6, 'g', ['on', 'off']), [0.8, 0.2, 0.1, 0.9])
h = BbnNode(Variable(7, 'h', ['on', 'off']), [0.05, 0.95, 0.95, 0.05, 0.95, 0.05, 0.95, 0.05])
bbn = Bbn() \
.add_node(a) \
.add_node(b) \
.add_node(c) \
.add_node(d) \
.add_node(e) \
.add_node(f) \
.add_node(g) \
.add_node(h) \
.add_edge(Edge(a, b, EdgeType.DIRECTED)) \
.add_edge(Edge(a, c, EdgeType.DIRECTED)) \
.add_edge(Edge(b, d, EdgeType.DIRECTED)) \
.add_edge(Edge(c, e, EdgeType.DIRECTED)) \
.add_edge(Edge(d, f, EdgeType.DIRECTED)) \
.add_edge(Edge(e, f, EdgeType.DIRECTED)) \
.add_edge(Edge(c, g, EdgeType.DIRECTED)) \
.add_edge(Edge(e, h, EdgeType.DIRECTED)) \
.add_edge(Edge(g, h, EdgeType.DIRECTED))
join_tree = InferenceController.apply(bbn)
ev = EvidenceBuilder() \
.with_node(join_tree.get_bbn_node_by_name('a')) \
.with_evidence('on', 1.0) \
.build()
join_tree.set_observation(ev)
for node in join_tree.get_bbn_nodes():
potential = join_tree.get_bbn_potential(node)
print(node)
print(potential)
Exact Inference, Gaussian Variables
The example belows shows how to perform inference on multivariate Gaussian variables.
import numpy as np
from pybbn.gaussian.inference import GaussianInference
def get_cowell_data():
"""
Gets Cowell data.
:return: Data and headers.
"""
n = 10000
Y = np.random.normal(0, 1, n)
X = np.random.normal(Y, 1, n)
Z = np.random.normal(X, 1, n)
D = np.vstack([Y, X, Z]).T
return D, ['Y', 'X', 'Z']
X, H = get_cowell_data()
M = X.mean(axis=0)
E = np.cov(X.T)
g = GaussianInference(H, M, E)
print(g.P)
g1 = g.do_inference('X', 1.5)
print(g1.P)
g2 = g.do_inferences([('Z', 1.5), ('X', 2.0)])
print(g2.P)
Building
To build, you will need 3.7. Managing environments through Anaconda is highly recommended to be able to build this project (though not absolutely required if you know what you are doing). Assuming you have installed Anaconda, you may create an environment as follows (make sure you cd
into the root of this project's location).
To create the environment, use the following commands.
conda env create -f environment.yml
If you want to use the environments with Jupyter, install the kernel.
conda activate pybbn37
python -m ipykernel install --user --name pybbn37 --display-name "pybbn37"
Then you may build the project as follows. (Note that in Python 3.6 you will get some warnings).
make build
To build the documents, go into the docs sub-directory and type in the following.
make html
Testing
You can do a fresh test with Docker as follows.
docker build -t pybbn-test:local -f Dockerfile.test .
Installing
From PyPi
Use pip to install the package as it has been published to PyPi.
pip install pybbn
From Source
If you check out the source do the following.
pip list | grep pybbn
pip uninstall pybbn
python setup.py install
pip list | grep pybbn
GraphViz issue
Make sure you install GraphViz on your system.
- CentOS:
yum install graphviz*
- Ubuntu:
sudo apt-get install graphviz libgraphviz-dev
- Mac OSX:
brew install graphviz
and when you install pygraphviz pip install pygraphviz --install-option="--include-path=/usr/local/lib/graphviz/" --install-option="--library-path=/usr/local/lib/graphviz/"
- Windows: use the msi installer
- For Anaconda + Windows, install pygraphviz from this channel
conda install -c alubbock pygraphviz
testpypi issue
You should NOT be doing this operation, but if you do want to install from testpypi
, then add the --extra-index-url
as follows.
pip install -i https://test.pypi.org/simple/ --extra-index-url https://pypi.org/simple/ pybbn
Other Python Bayesian Belief Network Inference Libraries
Here is a list of other Python libraries for inference in Bayesian Belief Networks.
Library | Algorithm | Algorithm Type | License |
---|
BayesPy | variational message passing | approximate | MIT |
pomegranate | loopy belief | approximate | MIT |
pgmpy | multiple | approximate/exact | MIT |
libpgm | likelihood sampling | approximate | Proprietary |
bayesnetinference | variable elimination | exact | None |
I found other packages in PyPI too.
Java
But I am coming from the Java mothership and I want to use Bayesian Belief Networks in Java. How do I perform probabilistic inference in Java?
This Python code base is a port of the original Java code.
Help
Citation
@misc{vang_2017,
title={PyBBN},
url={https://github.com/vangj/py-bbn/},
journal={GitHub},
author={Vang, Jee},
year={2017},
month={Jan}}
Online Articles
I found these online articles using PyBBN.
Copyright Stuff
Software
Copyright 2017 -- 2023 Jee Vang
Licensed under the Apache License, Version 2.0 (the "License");
you may not use this file except in compliance with the License.
You may obtain a copy of the License at
http://www.apache.org/licenses/LICENSE-2.0
Unless required by applicable law or agreed to in writing, software
distributed under the License is distributed on an "AS IS" BASIS,
WITHOUT WARRANTIES OR CONDITIONS OF ANY KIND, either express or implied.
See the License for the specific language governing permissions and
limitations under the License.
Art Copyright
Copyright 2020 Daytchia Vang