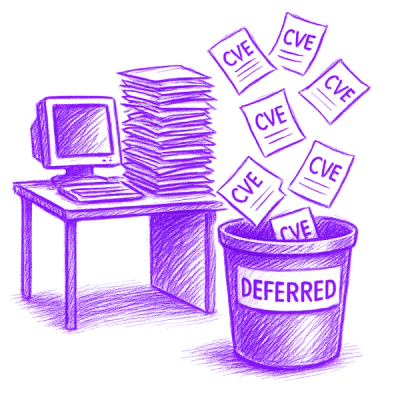
Security News
NVD Quietly Sweeps 100K+ CVEs Into a “Deferred” Black Hole
NVD now marks all pre-2018 CVEs as "Deferred," signaling it will no longer enrich older vulnerabilities, further eroding trust in its data.
PyDeequ is a Python API for Deequ, a library built on top of Apache Spark for defining "unit tests for data", which measure data quality in large datasets. PyDeequ is written to support usage of Deequ in Python.
There are 4 main components of Deequ, and they are:
Profiles
leverages Analyzers to analyze each column of a dataset.Analyzers
serve here as a foundational module that computes metrics for data profiling and validation at scale.SPARK_VERSION
to specify your Spark version!The following will quickstart you with some basic usage. For more in-depth examples, take a look in the tutorials/
directory for executable Jupyter notebooks of each module. For documentation on supported interfaces, view the documentation
.
You can install PyDeequ via pip.
pip install pydeequ
from pyspark.sql import SparkSession, Row
import pydeequ
spark = (SparkSession
.builder
.config("spark.jars.packages", pydeequ.deequ_maven_coord)
.config("spark.jars.excludes", pydeequ.f2j_maven_coord)
.getOrCreate())
df = spark.sparkContext.parallelize([
Row(a="foo", b=1, c=5),
Row(a="bar", b=2, c=6),
Row(a="baz", b=3, c=None)]).toDF()
from pydeequ.analyzers import *
analysisResult = AnalysisRunner(spark) \
.onData(df) \
.addAnalyzer(Size()) \
.addAnalyzer(Completeness("b")) \
.run()
analysisResult_df = AnalyzerContext.successMetricsAsDataFrame(spark, analysisResult)
analysisResult_df.show()
from pydeequ.profiles import *
result = ColumnProfilerRunner(spark) \
.onData(df) \
.run()
for col, profile in result.profiles.items():
print(profile)
from pydeequ.suggestions import *
suggestionResult = ConstraintSuggestionRunner(spark) \
.onData(df) \
.addConstraintRule(DEFAULT()) \
.run()
# Constraint Suggestions in JSON format
print(suggestionResult)
from pydeequ.checks import *
from pydeequ.verification import *
check = Check(spark, CheckLevel.Warning, "Review Check")
checkResult = VerificationSuite(spark) \
.onData(df) \
.addCheck(
check.hasSize(lambda x: x >= 3) \
.hasMin("b", lambda x: x == 0) \
.isComplete("c") \
.isUnique("a") \
.isContainedIn("a", ["foo", "bar", "baz"]) \
.isNonNegative("b")) \
.run()
checkResult_df = VerificationResult.checkResultsAsDataFrame(spark, checkResult)
checkResult_df.show()
Save to a Metrics Repository by adding the useRepository()
and saveOrAppendResult()
calls to your Analysis Runner.
from pydeequ.repository import *
from pydeequ.analyzers import *
metrics_file = FileSystemMetricsRepository.helper_metrics_file(spark, 'metrics.json')
repository = FileSystemMetricsRepository(spark, metrics_file)
key_tags = {'tag': 'pydeequ hello world'}
resultKey = ResultKey(spark, ResultKey.current_milli_time(), key_tags)
analysisResult = AnalysisRunner(spark) \
.onData(df) \
.addAnalyzer(ApproxCountDistinct('b')) \
.useRepository(repository) \
.saveOrAppendResult(resultKey) \
.run()
To load previous runs, use the repository
object to load previous results back in.
result_metrep_df = repository.load() \
.before(ResultKey.current_milli_time()) \
.forAnalyzers([ApproxCountDistinct('b')]) \
.getSuccessMetricsAsDataFrame()
After you've ran your jobs with PyDeequ, be sure to shut down your Spark session to prevent any hanging processes.
spark.sparkContext._gateway.shutdown_callback_server()
spark.stop()
Please refer to the contributing doc for how to contribute to PyDeequ.
This library is licensed under the Apache 2.0 License.
SDKMAN is a tool for managing parallel Versions of multiple Software Development Kits on any Unix based system. It provides a convenient command line interface for installing, switching, removing and listing Candidates. SDKMAN! installs smoothly on Mac OSX, Linux, WSL, Cygwin, etc... Support Bash and ZSH shells. See documentation on the SDKMAN! website.
Open your favourite terminal and enter the following:
$ curl -s https://get.sdkman.io | bash
If the environment needs tweaking for SDKMAN to be installed,
the installer will prompt you accordingly and ask you to restart.
Next, open a new terminal or enter:
$ source "$HOME/.sdkman/bin/sdkman-init.sh"
Lastly, run the following code snippet to ensure that installation succeeded:
$ sdk version
Install Java Now open favourite terminal and enter the following:
List the AdoptOpenJDK OpenJDK versions
$ sdk list java
To install For Java 11
$ sdk install java 11.0.10.hs-adpt
To install For Java 11
$ sdk install java 8.0.292.hs-adpt
Install Java Now open favourite terminal and enter the following:
List the Apache Spark versions:
$ sdk list spark
To install For Spark 3
$ sdk install spark 3.0.2
Poetry Commands
poetry install
poetry update
# --tree: List the dependencies as a tree.
# --latest (-l): Show the latest version.
# --outdated (-o): Show the latest version but only for packages that are outdated.
poetry show -o
Take a look at tests in tests/dataquality
and tests/jobs
$ poetry run pytest
If you have issues installing the dependencies listed above, another way to run the tests and verify your changes is through Docker. There is a Dockerfile that will install the required dependencies and run the tests in a container.
docker build . -t spark-3.3-docker-test
docker run spark-3.3-docker-test
FAQs
PyDeequ - Unit Tests for Data
We found that pydeequ demonstrated a healthy version release cadence and project activity because the last version was released less than a year ago. It has 2 open source maintainers collaborating on the project.
Did you know?
Socket for GitHub automatically highlights issues in each pull request and monitors the health of all your open source dependencies. Discover the contents of your packages and block harmful activity before you install or update your dependencies.
Security News
NVD now marks all pre-2018 CVEs as "Deferred," signaling it will no longer enrich older vulnerabilities, further eroding trust in its data.
Research
Security News
Lazarus-linked threat actors expand their npm malware campaign with new RAT loaders, hex obfuscation, and over 5,600 downloads across 11 packages.
Security News
Safari 18.4 adds support for Iterator Helpers and two other TC39 JavaScript features, bringing full cross-browser coverage to key parts of the ECMAScript spec.