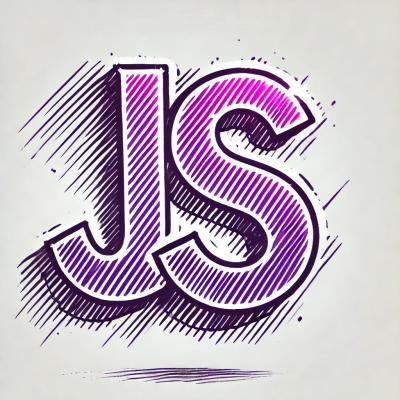
Security News
Oracle Drags Its Feet in the JavaScript Trademark Dispute
Oracle seeks to dismiss fraud claims in the JavaScript trademark dispute, delaying the case and avoiding questions about its right to the name.
Face related toolkit. This repo is still under construction to include more models.
The easiest way to install it is using pip:
pip install git+https://github.com/FacePerceiver/facer.git@main
No extra setup needs, pretrained weights will be downloaded automatically.
If you have trouble install from source, you can try install from PyPI:
pip install pyfacer
the PyPI version is not guaranteed to be the latest version, but we will try to keep it up to date.
We simply wrap a retinaface detector for easy usage.
import facer
image = facer.hwc2bchw(facer.read_hwc('data/twogirls.jpg')).to(device=device) # image: 1 x 3 x h x w
face_detector = facer.face_detector('retinaface/mobilenet', device=device)
with torch.inference_mode():
faces = face_detector(image)
facer.show_bchw(facer.draw_bchw(image, faces))
Check this notebook for full example.
Please consider citing
@inproceedings{deng2020retinaface,
title={Retinaface: Single-shot multi-level face localisation in the wild},
author={Deng, Jiankang and Guo, Jia and Ververas, Evangelos and Kotsia, Irene and Zafeiriou, Stefanos},
booktitle={Proceedings of the IEEE/CVF Conference on Computer Vision and Pattern Recognition},
pages={5203--5212},
year={2020}
}
We wrap the FaRL models for face parsing.
import torch
import facer
device = 'cuda' if torch.cuda.is_available() else 'cpu'
image = facer.hwc2bchw(facer.read_hwc('data/twogirls.jpg')).to(device=device) # image: 1 x 3 x h x w
face_detector = facer.face_detector('retinaface/mobilenet', device=device)
with torch.inference_mode():
faces = face_detector(image)
face_parser = facer.face_parser('farl/lapa/448', device=device) # optional "farl/celebm/448"
with torch.inference_mode():
faces = face_parser(image, faces)
seg_logits = faces['seg']['logits']
seg_probs = seg_logits.softmax(dim=1) # nfaces x nclasses x h x w
n_classes = seg_probs.size(1)
vis_seg_probs = seg_probs.argmax(dim=1).float()/n_classes*255
vis_img = vis_seg_probs.sum(0, keepdim=True)
facer.show_bhw(vis_img)
facer.show_bchw(facer.draw_bchw(image, faces))
Check this notebook for full example.
Please consider citing
@inproceedings{zheng2022farl,
title={General facial representation learning in a visual-linguistic manner},
author={Zheng, Yinglin and Yang, Hao and Zhang, Ting and Bao, Jianmin and Chen, Dongdong and Huang, Yangyu and Yuan, Lu and Chen, Dong and Zeng, Ming and Wen, Fang},
booktitle={Proceedings of the IEEE/CVF Conference on Computer Vision and Pattern Recognition},
pages={18697--18709},
year={2022}
}
We wrap the FaRL models for face alignment.
import torch
import cv2
from matplotlib import pyplot as plt
device = 'cuda' if torch.cuda.is_available() else 'cpu'
import facer
img_file = 'data/twogirls.jpg'
# image: 1 x 3 x h x w
image = facer.hwc2bchw(facer.read_hwc(img_file)).to(device=device)
face_detector = facer.face_detector('retinaface/mobilenet', device=device)
with torch.inference_mode():
faces = face_detector(image)
face_aligner = facer.face_aligner('farl/ibug300w/448', device=device) # optional: "farl/wflw/448", "farl/aflw19/448"
with torch.inference_mode():
faces = face_aligner(image, faces)
img = cv2.imread(img_file)[..., ::-1]
vis_img = img.copy()
for pts in faces['alignment']:
vis_img = facer.draw_landmarks(vis_img, None, pts.cpu().numpy())
plt.imshow(vis_img)
Check this notebook for full example.
Please consider citing
@inproceedings{zheng2022farl,
title={General facial representation learning in a visual-linguistic manner},
author={Zheng, Yinglin and Yang, Hao and Zhang, Ting and Bao, Jianmin and Chen, Dongdong and Huang, Yangyu and Yuan, Lu and Chen, Dong and Zeng, Ming and Wen, Fang},
booktitle={Proceedings of the IEEE/CVF Conference on Computer Vision and Pattern Recognition},
pages={18697--18709},
year={2022}
}
We wrap the FaRL models for face attribute recognition, the model achieves 92.06% accuracy on CelebA dataset.
import sys
import torch
import facer
device = "cuda" if torch.cuda.is_available() else "cpu"
# image: 1 x 3 x h x w
image = facer.hwc2bchw(facer.read_hwc("data/girl.jpg")).to(device=device)
face_detector = facer.face_detector("retinaface/mobilenet", device=device)
with torch.inference_mode():
faces = face_detector(image)
face_attr = facer.face_attr("farl/celeba/224", device=device)
with torch.inference_mode():
faces = face_attr(image, faces)
labels = face_attr.labels
face1_attrs = faces["attrs"][0] # get the first face's attributes
print(labels)
for prob, label in zip(face1_attrs, labels):
if prob > 0.5:
print(label, prob.item())
Check this notebook for full example.
Please consider citing
@inproceedings{zheng2022farl,
title={General facial representation learning in a visual-linguistic manner},
author={Zheng, Yinglin and Yang, Hao and Zhang, Ting and Bao, Jianmin and Chen, Dongdong and Huang, Yangyu and Yuan, Lu and Chen, Dong and Zeng, Ming and Wen, Fang},
booktitle={Proceedings of the IEEE/CVF Conference on Computer Vision and Pattern Recognition},
pages={18697--18709},
year={2022}
}
FAQs
Face related toolkit
We found that pyfacer demonstrated a healthy version release cadence and project activity because the last version was released less than a year ago. It has 1 open source maintainer collaborating on the project.
Did you know?
Socket for GitHub automatically highlights issues in each pull request and monitors the health of all your open source dependencies. Discover the contents of your packages and block harmful activity before you install or update your dependencies.
Security News
Oracle seeks to dismiss fraud claims in the JavaScript trademark dispute, delaying the case and avoiding questions about its right to the name.
Security News
The Linux Foundation is warning open source developers that compliance with global sanctions is mandatory, highlighting legal risks and restrictions on contributions.
Security News
Maven Central now validates Sigstore signatures, making it easier for developers to verify the provenance of Java packages.