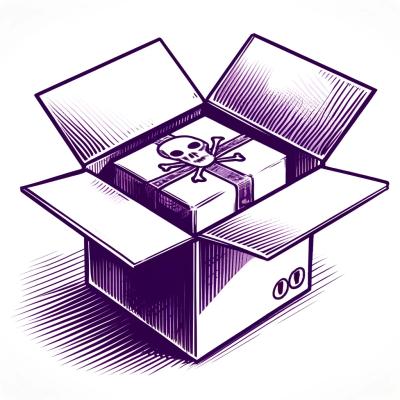
Security News
Research
Data Theft Repackaged: A Case Study in Malicious Wrapper Packages on npm
The Socket Research Team breaks down a malicious wrapper package that uses obfuscation to harvest credentials and exfiltrate sensitive data.
Implementation of visualisation and reporting analytics for Quantitative Investment Strategies
qis package implements analytics for visualisation of financial data, performance reporting, analysis of quantitative strategies.
qis package is split into 5 main modules with the dependecy path increasing sequentially as follows.
qis.utils
is module containing low level utilities for operations with pandas, numpy, and datetimes.
qis.perfstats
is module for computing performance statistics and performance attribution including returns, volatilities, etc.
qis.plots
is module for plotting and visualization apis.
qis.models
is module containing statistical models including filtering and regressions.
qis.portfolio
is high level module for analysis, simulation, backtesting, and reporting of quant strategies.
Function backtest_model_portfolio()
in qis.portfolio.backtester.py
takes instrument prices
and simulated weights from a generic strategy and compute the total return, performance attribution, and risk analysis
qis.examples
contains scripts with illustrations of QIS analytics.
qis.examples.factheets
contains scripts with examples of factsheets for simulated and actual strategies,
and cross-sectional analysis of backtests.
Install using
pip install qis
Upgrade using
pip install --upgrade qis
Close using
git clone https://github.com/ArturSepp/QuantInvestStrats.git
Core dependencies: python = ">=3.8", numba = ">=0.56.4", numpy = ">=1.22.4", scipy = ">=1.10", statsmodels = ">=0.13.5", pandas = ">=1.5.2", matplotlib = ">=3.2.2", seaborn = ">=0.12.2"
Optional dependencies: yfinance ">=0.1.38" (for getting test price data), pybloqs ">=1.2.13" (for producing html and pdf factsheets)
To use pybloqs for pandas > 2.x, locate file "...\Lib\site-packages\pybloqs\jinja\table.html" and change line 44 from {% for col_name, cell in row.iteritems() %} to {% for col_name, cell in row.items() %}
The script is located in qis.examples.performances
(https://github.com/ArturSepp/QuantInvestStrats/blob/master/qis/examples/performances.py)
import matplotlib.pyplot as plt
import seaborn as sns
import yfinance as yf
import qis
# define tickers and fetch price data
tickers = ['SPY', 'QQQ', 'EEM', 'TLT', 'IEF', 'SHY', 'LQD', 'HYG', 'GLD']
prices = yf.download(tickers, start=None, end=None)['Adj Close'][tickers].dropna()
# plotting price data with minimum usage
with sns.axes_style("darkgrid"):
fig, ax = plt.subplots(1, 1, figsize=(10, 7))
qis.plot_prices(prices=prices, x_date_freq='YE', ax=ax)
# 2-axis plot with drawdowns using sns styles
with sns.axes_style("darkgrid"):
fig, axs = plt.subplots(2, 1, figsize=(10, 7), tight_layout=True)
qis.plot_prices_with_dd(prices=prices, x_date_freq='YE', axs=axs)
# plot risk-adjusted performance table with excess Sharpe ratio
ust_3m_rate = yf.download('^IRX', start=None, end=None)['Adj Close'].dropna() / 100.0
# set parameters for computing performance stats including returns vols and regressions
perf_params = qis.PerfParams(freq='ME', freq_reg='QE', alpha_an_factor=4.0, rates_data=ust_3m_rate)
# perf_columns is list to display different perfomance metrics from enumeration PerfStat
fig = qis.plot_ra_perf_table(prices=prices,
perf_columns=[PerfStat.TOTAL_RETURN, PerfStat.PA_RETURN, PerfStat.PA_EXCESS_RETURN,
PerfStat.VOL, PerfStat.SHARPE_RF0,
PerfStat.SHARPE_EXCESS, PerfStat.SORTINO_RATIO, PerfStat.CALMAR_RATIO,
PerfStat.MAX_DD, PerfStat.MAX_DD_VOL,
PerfStat.SKEWNESS, PerfStat.KURTOSIS],
title=f"Risk-adjusted performance: {qis.get_time_period_label(prices, date_separator='-')}",
perf_params=perf_params)
# add benchmark regression using excess returns for linear beta
# regression frequency is specified using perf_params.freq_reg
# regression alpha is multiplied using perf_params.alpha_an_factor
fig = qis.plot_ra_perf_table_benchmark(prices=prices,
benchmark='SPY',
perf_columns=[PerfStat.TOTAL_RETURN, PerfStat.PA_RETURN, PerfStat.PA_EXCESS_RETURN,
PerfStat.VOL, PerfStat.SHARPE_RF0,
PerfStat.SHARPE_EXCESS, PerfStat.SORTINO_RATIO, PerfStat.CALMAR_RATIO,
PerfStat.MAX_DD, PerfStat.MAX_DD_VOL,
PerfStat.SKEWNESS, PerfStat.KURTOSIS,
PerfStat.ALPHA_AN, PerfStat.BETA, PerfStat.R2],
title=f"Risk-adjusted performance: {qis.get_time_period_label(prices, date_separator='-')} benchmarked with SPY",
perf_params=perf_params)
This report is adopted for reporting the risk-adjusted performance of several assets with the goal of cross-sectional comparision
Run example in qis.examples.factsheets.multi_assets.py
https://github.com/ArturSepp/QuantInvestStrats/blob/master/qis/examples/factsheets/multi_assets.py
This report is adopted for report performance, risk, and trading statistics for either backtested or actual strategy with strategy data passed as PortfolioData object
Run example in qis.examples.factsheets.strategy.py
https://github.com/ArturSepp/QuantInvestStrats/blob/master/qis/examples/factsheets/strategy.py
This report is adopted for report performance and marginal comparison of strategy vs a benchmark strategy (data for both are passed using individual PortfolioData object)
Run example in qis.examples.factsheets.strategy_benchmark.py
https://github.com/ArturSepp/QuantInvestStrats/blob/master/qis/examples/factsheets/strategy_benchmark.py
Brinson-Fachler performance attribution (https://en.wikipedia.org/wiki/Performance_attribution)
This report is adopted to examine the sensitivity of backtested strategy to a parameter or set of parameters:
Run example in qis.examples.factsheets.multi_strategy.py
https://github.com/ArturSepp/QuantInvestStrats/blob/master/qis/examples/factsheets/multi_strategy.py
Recommended package to work with notebooks:
pip install notebook
Starting local server
jupyter notebook
Examples of using qis analytics jupyter notebooks are located here https://github.com/ArturSepp/QuantInvestStrats/blob/master/qis/examples/notebooks
If you are interested in extending and improving QIS analytics, please consider contributing to the library.
I have found it is a good practice to isolate general purpose and low level analytics and visualizations, which can be outsourced and shared, while keeping the focus on developing high level commercial applications.
There are a number of requirements:
The code is Pep 8 compliant
Reliance on common Python data types including numpy arrays, pandas, and dataclasses.
Transparent naming of functions and data types with enough comments. Type annotations of functions and arguments is a must.
Each submodule has a unit test for core functions and a localised entry point to core functions.
Avoid "super" pythonic constructions. Readability is the priority.
Core Changes
Enhanced documentation and readme examples.
Docstrings for key functions.
Reporting analytics and factsheets generation enhancing to matplotlib.
QIS package is distributed FREE & WITHOUT ANY WARRANTY under the GNU GENERAL PUBLIC LICENSE.
See the LICENSE.txt in the release for details.
Please report any bugs or suggestions by opening an issue.
FAQs
Implementation of visualisation and reporting analytics for Quantitative Investment Strategies
We found that qis demonstrated a healthy version release cadence and project activity because the last version was released less than a year ago. It has 1 open source maintainer collaborating on the project.
Did you know?
Socket for GitHub automatically highlights issues in each pull request and monitors the health of all your open source dependencies. Discover the contents of your packages and block harmful activity before you install or update your dependencies.
Security News
Research
The Socket Research Team breaks down a malicious wrapper package that uses obfuscation to harvest credentials and exfiltrate sensitive data.
Research
Security News
Attackers used a malicious npm package typosquatting a popular ESLint plugin to steal sensitive data, execute commands, and exploit developer systems.
Security News
The Ultralytics' PyPI Package was compromised four times in one weekend through GitHub Actions cache poisoning and failure to rotate previously compromised API tokens.