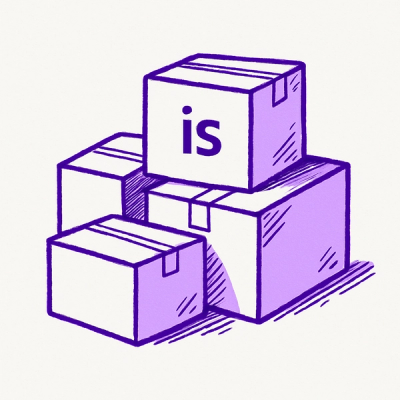
Security News
npm ‘is’ Package Hijacked in Expanding Supply Chain Attack
The ongoing npm phishing campaign escalates as attackers hijack the popular 'is' package, embedding malware in multiple versions.
Efficient RaggedBuffer datatype that implements 3D arrays with variable-length 2nd dimension.
This Python package implements an efficient RaggedBuffer
datatype that is similar to
a 3D numpy array, but which allows for variable sequence length in the second
dimension. It was created primarily for use in enn-trainer
and currently only supports a small selection of the numpy array methods.
Install the package with pip install ragged-buffer
.
The package currently supports three RaggedBuffer
variants, RaggedBufferF32
, RaggedBufferI64
, and RaggedBufferBool
.
There are three ways to create a RaggedBuffer
:
RaggedBufferF32(features: int)
creates an empty RaggedBuffer
with the specified number of features.RaggedBufferF32.from_flattened(flattened: np.ndarray, lenghts: np.ndarray)
creates a RaggedBuffer
from a flattened 2D numpy array and a 1D numpy array of lengths.RaggedBufferF32.from_array
creates a RaggedBuffer
(with equal sequence lenghts) from a 3D numpy array.Creating an empty buffer and pushing each row:
import numpy as np
from ragged_buffer import RaggedBufferF32
# Create an empty RaggedBuffer with a feature size of 3
buffer = RaggedBufferF32(3)
# Push sequences with 3, 5, 0, and 1 elements
buffer.push(np.array([[1, 2, 3], [4, 5, 6], [7, 8, 9]], dtype=np.float32))
buffer.push(np.array([[10, 11, 12], [13, 14, 15], [16, 17, 18], [19, 20, 21], [22, 23, 24]], dtype=np.float32))
buffer.push(np.array([], dtype=np.float32)) # Alternative: `buffer.push_empty()`
buffer.push(np.array([[25, 25, 27]], dtype=np.float32))
Creating a RaggedBuffer from a flat 2D numpy array which combines the first and second dimension, and an array of sequence lengths:
import numpy as np
from ragged_buffer import RaggedBufferF32
buffer = RaggedBufferF32.from_flattened(
np.array([[1, 2, 3], [4, 5, 6], [7, 8, 9], [10, 11, 12], [13, 14, 15], [16, 17, 18], [19, 20, 21], [22, 23, 24], [25, 25, 27]], dtype=np.float32),
np.array([3, 5, 0, 1], dtype=np.int64))
)
Creating a RaggedBuffer from a 3D numpy array (all sequences have the same length):
import numpy as np
from ragged_buffer import RaggedBufferF32
buffer = RaggedBufferF32.from_array(np.zeros((4, 5, 3), dtype=np.float32))
The size0
, size1
, and size2
methods return the number of sequences, the number of elements in a sequence, and the number of features respectively.
import numpy as np
from ragged_buffer import RaggedBufferF32
buffer = RaggedBufferF32.from_flattened(
np.zeros((9, 64), dtype=np.float32),
np.array([3, 5, 0, 1], dtype=np.int64))
)
# Get size of the first/batch dimension.
assert buffer.size0() == 10
# Get size of individual sequences.
assert buffer.size1(1) == 5
assert buffer.size1(2) == 0
# Get size of the last/feature dimension.
assert buffer.size2() == 64
as_aray
converts a RaggedBuffer
to a flat 2D numpy array that combines the first and second dimension.
import numpy as np
from ragged_buffer import RaggedBufferI64
buffer = RaggedBufferI64(1)
buffer.push(np.array([[1], [1], [1]], dtype=np.int64))
buffer.push(np.array([[2], [2]], dtype=np.int64))
assert np.all(buffer.as_array(), np.array([[1], [1], [1], [2], [2]], dtype=np.int64))
You can index a RaggedBuffer
with a single integer (returning a RaggedBuffer
with a single sequence), or with a numpy array of integers selecting/permuting multiple sequences.
import numpy as np
from ragged_buffer import RaggedBufferF32
# Create a new `RaggedBufferF32`
buffer = RaggedBufferF32.from_flattened(
np.arange(0, 40, dtype=np.float32).reshape(10, 4),
np.array([3, 5, 0, 1], dtype=np.int64)
)
# Retrieve the first sequence.
assert np.all(
buffer[0].as_array() ==
np.array([[0, 1, 2, 3], [4, 5, 6, 7], [8, 9, 10, 11]], dtype=np.float32)
)
# Get a RaggedBatch with 2 randomly selected sequences.
buffer[np.random.permutation(4)[:2]]
You can add two RaggedBuffer
s with the +
operator if they have the same number of sequences, sequence lengths, and features. You can also add a RaggedBuffer
where all sequences have a length of 1 to a RaggedBuffer
with variable length sequences, broadcasting along each sequence.
import numpy as np
from ragged_buffer import RaggedBufferF32
# Create ragged buffer with dimensions (3, [1, 3, 2], 1)
rb3 = RaggedBufferI64(1)
rb3.push(np.array([[0]], dtype=np.int64))
rb3.push(np.array([[0], [1], [2]], dtype=np.int64))
rb3.push(np.array([[0], [5]], dtype=np.int64))
# Create ragged buffer with dimensions (3, [1, 1, 1], 1)
rb4 = RaggedBufferI64.from_array(np.array([0, 3, 10], dtype=np.int64).reshape(3, 1, 1))
# Add rb3 and rb4, broadcasting along the sequence dimension.
rb5 = rb3 + rb4
assert np.all(
rb5.as_array() == np.array([[0], [3], [4], [5], [10], [15]], dtype=np.int64)
)
The extend
method can be used to mutate a RaggedBuffer
by appending another RaggedBuffer
to it.
import numpy as np
from ragged_buffer import RaggedBufferF32
rb1 = RaggedBufferF32.from_array(np.zeros((4, 5, 3), dtype=np.float32))
rb2 = RaggedBufferF32.from_array(np.zeros((2, 5, 3), dtype=np.float32))
rb1.extend(r2)
assert rb1.size0() == 6
The clear
method removes all elements from a RaggedBuffer
without deallocating the underlying memory.
import numpy as np
from ragged_buffer import RaggedBufferF32
rb = RaggedBufferF32.from_array(np.zeros((4, 5, 3), dtype=np.float32))
rb.clear()
assert rb.size0() == 0
ENN Ragged Buffer dual-licensed under Apache-2.0 and MIT.
FAQs
Efficient RaggedBuffer datatype that implements 3D arrays with variable-length 2nd dimension.
We found that ragged-buffer demonstrated a healthy version release cadence and project activity because the last version was released less than a year ago. It has 1 open source maintainer collaborating on the project.
Did you know?
Socket for GitHub automatically highlights issues in each pull request and monitors the health of all your open source dependencies. Discover the contents of your packages and block harmful activity before you install or update your dependencies.
Security News
The ongoing npm phishing campaign escalates as attackers hijack the popular 'is' package, embedding malware in multiple versions.
Security News
A critical flaw in the popular npm form-data package could allow HTTP parameter pollution, affecting millions of projects until patched versions are adopted.
Security News
Bun 1.2.19 introduces isolated installs for smoother monorepo workflows, along with performance boosts, new tooling, and key compatibility fixes.