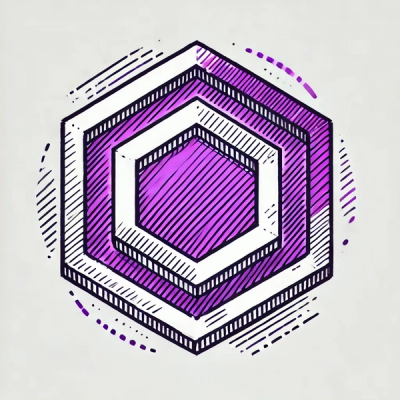
Security News
ESLint Adds Official Support for Linting HTML
ESLint now supports HTML linting with 48 new rules, expanding its language plugin system to cover more of the modern web development stack.
Selective is a white-box feature selection library that supports supervised and unsupervised selection methods for classification and regression tasks.
Selective also provides optimized item selection based on diversity of text embeddings (via TextWiser) and the coverage of binary labels by solving a multi-objective optimization problem (CPAIOR'21, DSO@IJCAI'22). The approach showed to speed-up online experimentation significantly and boost recommender systems NVIDIA GTC'22.
The library provides:
Selective is developed by the Artificial Intelligence Center of Excellence at Fidelity Investments.
# Import Selective and SelectionMethod
from sklearn.datasets import fetch_california_housing
from feature.utils import get_data_label
from feature.selector import Selective, SelectionMethod
# Data
data, label = get_data_label(fetch_california_housing())
# Feature selectors from simple to more complex
selector = Selective(SelectionMethod.Variance(threshold=0.0))
selector = Selective(SelectionMethod.Correlation(threshold=0.5, method="pearson"))
selector = Selective(SelectionMethod.Statistical(num_features=3, method="anova"))
selector = Selective(SelectionMethod.Linear(num_features=3, regularization="none"))
selector = Selective(SelectionMethod.TreeBased(num_features=3))
# Feature reduction
subset = selector.fit_transform(data, label)
print("Reduction:", list(subset.columns))
print("Scores:", list(selector.get_absolute_scores()))
Method | Options |
---|---|
Variance per Feature | threshold |
Correlation pairwise Features | Pearson Correlation Coefficient Kendall Rank Correlation Coefficient Spearman's Rank Correlation Coefficient |
Statistical Analysis | ANOVA F-test Classification F-value Regression Chi-Square Mutual Information Classification Variance Inflation Factor |
Linear Methods | Linear Regression Logistic Regression Lasso Regularization Ridge Regularization |
Tree-based Methods | Decision Tree Random Forest Extra Trees Classifier XGBoost LightGBM AdaBoost CatBoost Gradient Boosting Tree |
Text-based Methods | featurization_method = TextWiser optimization_method = ["exact", "greedy", "kmeans", "random"] cost_metric = ["unicost", "diverse"] |
# Imports
from sklearn.datasets import fetch_california_housing
from feature.utils import get_data_label
from xgboost import XGBClassifier, XGBRegressor
from feature.selector import SelectionMethod, benchmark, calculate_statistics
# Data
data, label = get_data_label(fetch_california_housing())
# Selectors
corr_threshold = 0.5
num_features = 3
tree_params = {"n_estimators": 50, "max_depth": 5, "random_state": 111, "n_jobs": 4}
selectors = {
# Correlation methods
"corr_pearson": SelectionMethod.Correlation(corr_threshold, method="pearson"),
"corr_kendall": SelectionMethod.Correlation(corr_threshold, method="kendall"),
"corr_spearman": SelectionMethod.Correlation(corr_threshold, method="spearman"),
# Statistical methods
"stat_anova": SelectionMethod.Statistical(num_features, method="anova"),
"stat_chi_square": SelectionMethod.Statistical(num_features, method="chi_square"),
"stat_mutual_info": SelectionMethod.Statistical(num_features, method="mutual_info"),
# Linear methods
"linear": SelectionMethod.Linear(num_features, regularization="none"),
"lasso": SelectionMethod.Linear(num_features, regularization="lasso", alpha=1000),
"ridge": SelectionMethod.Linear(num_features, regularization="ridge", alpha=1000),
# Non-linear tree-based methods
"random_forest": SelectionMethod.TreeBased(num_features),
"xgboost_classif": SelectionMethod.TreeBased(num_features, estimator=XGBClassifier(**tree_params)),
"xgboost_regress": SelectionMethod.TreeBased(num_features, estimator=XGBRegressor(**tree_params))
}
# Benchmark (sequential)
score_df, selected_df, runtime_df = benchmark(selectors, data, label, cv=5)
print(score_df, "\n\n", selected_df, "\n\n", runtime_df)
# Benchmark (in parallel)
score_df, selected_df, runtime_df = benchmark(selectors, data, label, cv=5, n_jobs=4)
print(score_df, "\n\n", selected_df, "\n\n", runtime_df)
# Get benchmark statistics by feature
stats_df = calculate_statistics(score_df, selected_df)
print(stats_df)
This example shows how to use text-based selection. In this scenario, we would like to select a subset of articles that is most diverse in the text embedding space and covers a range of topics.
# Import Selective and TextWiser
import pandas as pd
from feature.selector import Selective, SelectionMethod
from textwiser import TextWiser, Embedding, Transformation
# Data with the text content of each article
data = pd.DataFrame({"article_1": ["article text here"],
"article_2": ["article text here"],
"article_3": ["article text here"],
"article_4": ["article text here"],
"article_5": ["article text here"]})
# Labels to denote 0/1 coverage metadata for each article
# across four labels, e.g., sports, international, entertainment, science
labels = pd.DataFrame({"article_1": [1, 1, 0, 1],
"article_2": [0, 1, 0, 0],
"article_3": [0, 0, 1, 0],
"article_4": [0, 0, 1, 1],
"article_5": [1, 1, 1, 0]},
index=["label_1", "label_2", "label_3", "label_4"])
# TextWiser featurization method to create text embeddings
textwiser = TextWiser(Embedding.TfIdf(), Transformation.NMF(n_components=20))
# Text-based selection
# The goal is to select a subset of articles
# that is most diverse in the text embedding space of articles
# and covers the most labels in each topic
selector = Selective(SelectionMethod.TextBased(num_features=2, featurization_method=textwiser))
# Feature reduction
subset = selector.fit_transform(data, labels)
print("Reduction:", list(subset.columns))
import pandas as pd
from sklearn.datasets import fetch_california_housing
from feature.utils import get_data_label
from feature.selector import SelectionMethod, Selective, plot_importance
# Data
data, label = get_data_label(fetch_california_housing())
# Feature Selector
selector = Selective(SelectionMethod.Linear(num_features=8, regularization="none"))
subset = selector.fit_transform(data, label)
# Plot Feature Importance
df = pd.DataFrame(selector.get_absolute_scores(), index=data.columns)
plot_importance(df)
Selective requires Python 3.7+ and can be installed from PyPI using pip install selective
.
Alternatively, you can build a wheel package on your platform from scratch using the source code:
git clone https://github.com/fidelity/selective.git
cd selective
pip install setuptools wheel # if wheel is not installed
python setup.py sdist bdist_wheel
pip install dist/selective-X.X.X-py3-none-any.whl
cd selective
python -m unittest discover tests
Please submit bug reports and feature requests as Issues.
Selective is licensed under the GNU GPL 3.0.
FAQs
feature selection library
We found that selective demonstrated a healthy version release cadence and project activity because the last version was released less than a year ago. It has 4 open source maintainers collaborating on the project.
Did you know?
Socket for GitHub automatically highlights issues in each pull request and monitors the health of all your open source dependencies. Discover the contents of your packages and block harmful activity before you install or update your dependencies.
Security News
ESLint now supports HTML linting with 48 new rules, expanding its language plugin system to cover more of the modern web development stack.
Security News
CISA is discontinuing official RSS support for KEV and cybersecurity alerts, shifting updates to email and social media, disrupting automation workflows.
Security News
The MCP community is launching an official registry to standardize AI tool discovery and let agents dynamically find and install MCP servers.