


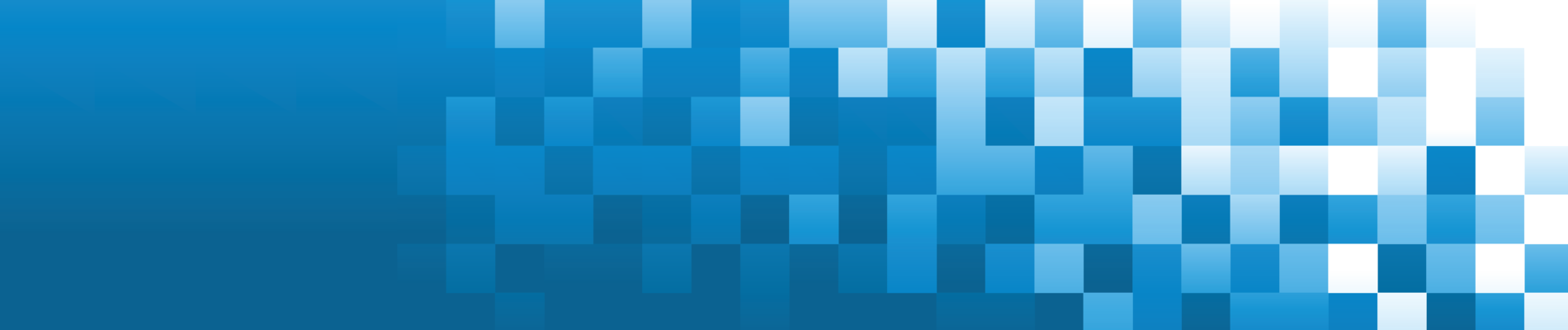
Silero Models
Silero Models: pre-trained enterprise-grade STT / TTS models and benchmarks.
Enterprise-grade STT made refreshingly simple (seriously, see benchmarks).
We provide quality comparable to Google's STT (and sometimes even better) and we are not Google.
As a bonus:
- No Kaldi;
- No compilation;
- No 20-step instructions;
Also we have published TTS models that satisfy the following criteria:
- One-line usage;
- A large library of voices;
- A fully end-to-end pipeline;
- Naturally sounding speech;
- No GPU or training required;
- Minimalism and lack of dependencies;
- Faster than real-time on one CPU thread (!!!);
- Support for 16kHz and 8kHz out of the box;
Also we have published a model for text repunctuation and recapitalization that:
- Inserts capital letters and basic punctuation marks (dot, comma, hyphen, question mark, exclamation mark, dash for Russian);
- Works for 4 languages (Russian, English, German, Spanish) and can be extended;
- By design is domain agnostic and is not based on any hard-coded rules;
- Has non-trivial metrics and succeeds in the task of improving text readability;
Installation and Basics
You can basically use our models in 3 flavours:
- Via PyTorch Hub:
torch.hub.load()
; - Via pip:
pip install silero
and then import silero
; - Via caching the required models and utils manually and modifying if necessary;
Models are downloaded on demand both by pip and PyTorch Hub. If you need caching, do it manually or via invoking a necessary model once (it will be downloaded to a cache folder). Please see these docs for more information.
PyTorch Hub and pip package are based on the same code. Hence all examples, historically based on torch.hub.load
can be used with a pip-package via this basic change:
# before
torch.hub.load(repo_or_dir='snakers4/silero-models',
model='silero_stt', # or silero_tts or silero_te
**kwargs)
# after
from silero import silero_stt, silero_tts, silero_te
silero_stt(**kwargs)
Speech-To-Text
All of the provided models are listed in the models.yml file.
Any meta-data and newer versions will be added there.
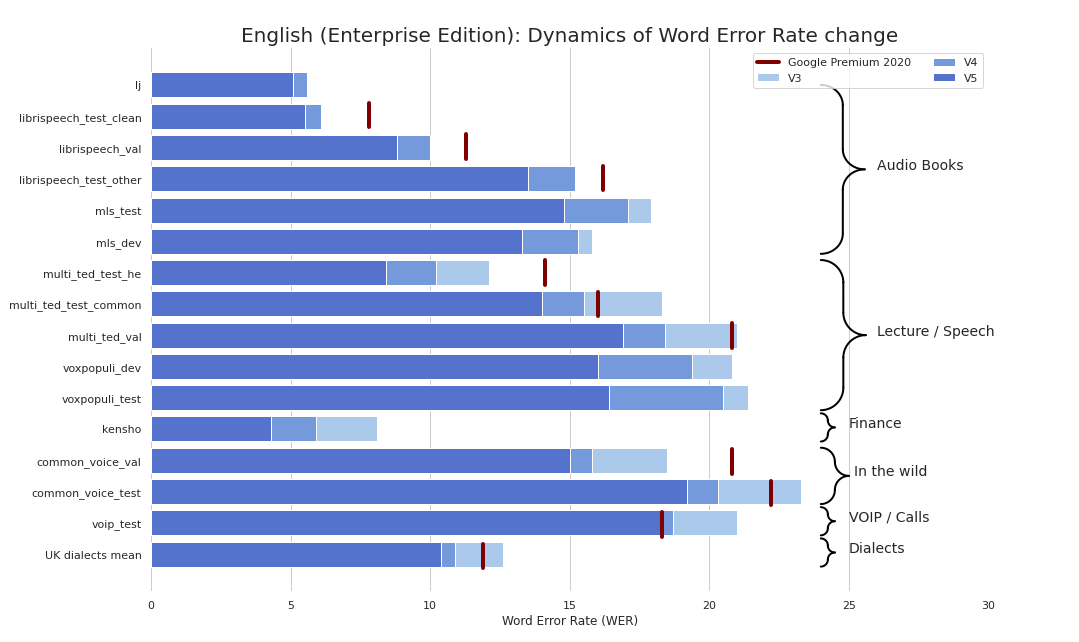
Currently we provide the following checkpoints:
| PyTorch | ONNX | Quantization | Quality | Colab |
---|
English (en_v6 ) | :heavy_check_mark: | :heavy_check_mark: | :heavy_check_mark: | link |  |
English (en_v5 ) | :heavy_check_mark: | :heavy_check_mark: | :heavy_check_mark: | link |  |
German (de_v4 ) | :heavy_check_mark: | :heavy_check_mark: | :hourglass: | link |  |
English (en_v3 ) | :heavy_check_mark: | :heavy_check_mark: | :heavy_check_mark: | link |  |
German (de_v3 ) | :heavy_check_mark: | :hourglass: | :hourglass: | link |  |
German (de_v1 ) | :heavy_check_mark: | :heavy_check_mark: | :hourglass: | link |  |
Spanish (es_v1 ) | :heavy_check_mark: | :heavy_check_mark: | :hourglass: | link |  |
Ukrainian (ua_v3 ) | :heavy_check_mark: | :heavy_check_mark: | :heavy_check_mark: | N/A |  |
Model flavours:
| jit | jit | jit | jit | jit_q | jit_q | onnx | onnx | onnx | onnx |
---|
| xsmall | small | large | xlarge | xsmall | small | xsmall | small | large | xlarge |
English en_v6 | | :heavy_check_mark: | | :heavy_check_mark: | | :heavy_check_mark: | | :heavy_check_mark: | | :heavy_check_mark: |
English en_v5 | | :heavy_check_mark: | | :heavy_check_mark: | | :heavy_check_mark: | | :heavy_check_mark: | | :heavy_check_mark: |
English en_v4_0 | | | :heavy_check_mark: | | | | | | :heavy_check_mark: | |
English en_v3 | :heavy_check_mark: | :heavy_check_mark: | :heavy_check_mark: | | :heavy_check_mark: | :heavy_check_mark: | :heavy_check_mark: | :heavy_check_mark: | :heavy_check_mark: | |
German de_v4 | | | :heavy_check_mark: | | | | | | :heavy_check_mark: | |
German de_v3 | | | :heavy_check_mark: | | | | | | | |
German de_v1 | | :heavy_check_mark: | | | | | :heavy_check_mark: | | | |
Spanish es_v1 | | :heavy_check_mark: | | | | | :heavy_check_mark: | | | |
Ukrainian ua_v3 | | :heavy_check_mark: | | | :heavy_check_mark: | | :heavy_check_mark: | | | |
Dependencies
- All examples:
torch
, 1.8+ (used to clone the repo in tf and onnx examples), breaking changes for version older than 1.6torchaudio
, latest version bound to PyTorch should workomegaconf
, latest just should work
- Additional for ONNX examples:
onnx
, latest just should workonnxruntime
, latest just should work
- Additional for TensorFlow examples:
tensorflow
, latest just should worktensorflow_hub
, latest just should work
Please see the provided Colab for details for each example below. All examples are maintained to work with the latest major packaged versions of the installed libraries.
PyTorch


import torch
import zipfile
import torchaudio
from glob import glob
device = torch.device('cpu')
model, decoder, utils = torch.hub.load(repo_or_dir='snakers4/silero-models',
model='silero_stt',
language='en',
device=device)
(read_batch, split_into_batches,
read_audio, prepare_model_input) = utils
torch.hub.download_url_to_file('https://opus-codec.org/static/examples/samples/speech_orig.wav',
dst ='speech_orig.wav', progress=True)
test_files = glob('speech_orig.wav')
batches = split_into_batches(test_files, batch_size=10)
input = prepare_model_input(read_batch(batches[0]),
device=device)
output = model(input)
for example in output:
print(decoder(example.cpu()))
ONNX

You can run our model everywhere, where you can import the ONNX model or run ONNX runtime.
import onnx
import torch
import onnxruntime
from omegaconf import OmegaConf
language = 'en'
_, decoder, utils = torch.hub.load(repo_or_dir='snakers4/silero-models', model='silero_stt', language=language)
(read_batch, split_into_batches,
read_audio, prepare_model_input) = utils
torch.hub.download_url_to_file('https://raw.githubusercontent.com/snakers4/silero-models/master/models.yml', 'models.yml')
models = OmegaConf.load('models.yml')
available_languages = list(models.stt_models.keys())
assert language in available_languages
torch.hub.download_url_to_file(models.stt_models.en.latest.onnx, 'model.onnx', progress=True)
onnx_model = onnx.load('model.onnx')
onnx.checker.check_model(onnx_model)
ort_session = onnxruntime.InferenceSession('model.onnx')
torch.hub.download_url_to_file('https://opus-codec.org/static/examples/samples/speech_orig.wav', dst ='speech_orig.wav', progress=True)
test_files = ['speech_orig.wav']
batches = split_into_batches(test_files, batch_size=10)
input = prepare_model_input(read_batch(batches[0]))
onnx_input = input.detach().cpu().numpy()
ort_inputs = {'input': onnx_input}
ort_outs = ort_session.run(None, ort_inputs)
decoded = decoder(torch.Tensor(ort_outs[0])[0])
print(decoded)
TensorFlow

SavedModel example
import os
import torch
import subprocess
import tensorflow as tf
import tensorflow_hub as tf_hub
from omegaconf import OmegaConf
language = 'en'
_, decoder, utils = torch.hub.load(repo_or_dir='snakers4/silero-models', model='silero_stt', language=language)
(read_batch, split_into_batches,
read_audio, prepare_model_input) = utils
torch.hub.download_url_to_file('https://raw.githubusercontent.com/snakers4/silero-models/master/models.yml', 'models.yml')
models = OmegaConf.load('models.yml')
available_languages = list(models.stt_models.keys())
assert language in available_languages
torch.hub.download_url_to_file(models.stt_models.en.latest.tf, 'tf_model.tar.gz')
subprocess.run('rm -rf tf_model && mkdir tf_model && tar xzfv tf_model.tar.gz -C tf_model', shell=True, check=True)
tf_model = tf.saved_model.load('tf_model')
torch.hub.download_url_to_file('https://opus-codec.org/static/examples/samples/speech_orig.wav', dst ='speech_orig.wav', progress=True)
test_files = ['speech_orig.wav']
batches = split_into_batches(test_files, batch_size=10)
input = prepare_model_input(read_batch(batches[0]))
res = tf_model.signatures["serving_default"](tf.constant(input.numpy()))['output_0']
print(decoder(torch.Tensor(res.numpy())[0]))
Text-To-Speech
Models and Speakers
All of the provided models are listed in the models.yml file. Any meta-data and newer versions will be added there.
V3
V3 models support SSML. Also see Colab examples for main SSML tag usage.
ID | Speakers | Auto-stress | Language | SR | Colab |
---|
v3_1_ru | aidar , baya , kseniya , xenia , eugene , random | yes | ru (Russian) | 8000 , 24000 , 48000 |  |
v3_en | en_0 , en_1 , ..., en_117 , random | no | en (English) | 8000 , 24000 , 48000 |  |
v3_en_indic | tamil_female , ..., assamese_male , random | no | en (English) | 8000 , 24000 , 48000 |  |
v3_de | eva_k , ..., karlsson , random | no | de (German) | 8000 , 24000 , 48000 |  |
v3_es | es_0 , es_1 , es_2 , random | no | es (Spanish) | 8000 , 24000 , 48000 |  |
v3_fr | fr_0 , ..., fr_5 , random | no | fr (French) | 8000 , 24000 , 48000 |  |
v3_tt | dilyara | no | tt (Tatar) | 8000 , 24000 , 48000 |  |
v3_ua | mykyta , random | no | ua (Ukrainian) | 8000 , 24000 , 48000 |  |
v3_uz | dilnavoz | no | uz (Uzbek) | 8000 , 24000 , 48000 |  |
v3_xal | erdni , delghir , random | no | xal (Kalmyk) | 8000 , 24000 , 48000 |  |
v3_indic | hindi_male , hindi_female , ..., random | no | indic (Hindi, Telugu, ...) | 8000 , 24000 , 48000 |  |
ru_v3 | aidar , baya , kseniya , xenia , random | yes | ru (Russian) | 8000 , 24000 , 48000 |  |
Dependencies
Basic dependencies for colab examples:
torch
, 1.10+;torchaudio
, latest version bound to PyTorch should work (required only because models are hosted together with STT, not required for work);omegaconf
, latest (can be removed as well, if you do not load all of the configs);
PyTorch


import torch
language = 'ru'
model_id = 'v3_1_ru'
sample_rate = 48000
speaker = 'xenia'
device = torch.device('cpu')
model, example_text = torch.hub.load(repo_or_dir='snakers4/silero-models',
model='silero_tts',
language=language,
speaker=model_id)
model.to(device)
audio = model.apply_tts(text=example_text,
speaker=speaker,
sample_rate=sample_rate)
Standalone Use
- Standalone usage just requires PyTorch 1.10+ and python standard library;
- Please see the detailed examples in Colab;
import os
import torch
device = torch.device('cpu')
torch.set_num_threads(4)
local_file = 'model.pt'
if not os.path.isfile(local_file):
torch.hub.download_url_to_file('https://models.silero.ai/models/tts/ru/v3_1_ru.pt',
local_file)
model = torch.package.PackageImporter(local_file).load_pickle("tts_models", "model")
model.to(device)
example_text = 'В недрах тундры выдры в г+етрах т+ырят в вёдра ядра кедров.'
sample_rate = 48000
speaker='baya'
audio_paths = model.save_wav(text=example_text,
speaker=speaker,
sample_rate=sample_rate)
SSML
Check out our TTS Wiki page.
Indic languages
Example
(!!!) All input sentences should be romanized to ISO format using aksharamukha
tool. An example for hindi
:
import torch
from aksharamukha import transliterate
model, example_text = torch.hub.load(repo_or_dir='snakers4/silero-models',
model='silero_tts',
language='indic',
speaker='v3_indic')
orig_text = "प्रसिद्द कबीर अध्येता, पुरुषोत्तम अग्रवाल का यह शोध आलेख, उस रामानंद की खोज करता है"
roman_text = transliterate.process('Devanagari', 'ISO', orig_text)
print(roman_text)
audio = model.apply_tts(roman_text,
speaker='hindi_male')
Supported languages
Language | Speakers | Romanization function |
---|
hindi | hindi_female , hindi_male | transliterate.process('Devanagari', 'ISO', orig_text) |
malayalam | malayalam_female , malayalam_male | transliterate.process('Malayalam', 'ISO', orig_text) |
manipuri | manipuri_female | transliterate.process('Bengali', 'ISO', orig_text) |
bengali | bengali_female , bengali_male | transliterate.process('Bengali', 'ISO', orig_text) |
rajasthani | rajasthani_female , rajasthani_female | transliterate.process('Devanagari', 'ISO', orig_text) |
tamil | tamil_female , tamil_male | transliterate.process('Tamil', 'ISO', orig_text, pre_options=['TamilTranscribe']) |
telugu | telugu_female , telugu_male | transliterate.process('Telugu', 'ISO', orig_text) |
gujarati | gujarati_female , gujarati_male | transliterate.process('Gujarati', 'ISO', orig_text) |
kannada | kannada_female , kannada_male | transliterate.process('Kannada', 'ISO', orig_text) |
Text-Enhancement
Languages | Quantization | Quality | Colab |
---|
'en', 'de', 'ru', 'es' | :heavy_check_mark: | link |  |
Dependencies
Basic dependencies for colab examples:
torch
, 1.9+;pyyaml
, but it's installed with torch itself
Standalone Use
- Standalone usage just requires PyTorch 1.9+ and python standard library;
- Please see the detailed examples in Colab;
import torch
model, example_texts, languages, punct, apply_te = torch.hub.load(repo_or_dir='snakers4/silero-models',
model='silero_te')
input_text = input('Enter input text\n')
apply_te(input_text, lan='en')
FAQ
Wiki
Also check out our wiki.
Performance and Quality
Please refer to this wiki sections:
Adding new Languages
Please refer here.
Contact
Get in Touch
Try our models, create an issue, join our chat, email us, read our news.
Commercial Inquiries
Please see our wiki and tiers for relevant information and email us.
Citations
@misc{Silero Models,
author = {Silero Team},
title = {Silero Models: pre-trained enterprise-grade STT / TTS models and benchmarks},
year = {2021},
publisher = {GitHub},
journal = {GitHub repository},
howpublished = {\url{https://github.com/snakers4/silero-models}},
commit = {insert_some_commit_here},
email = {hello@silero.ai}
}
Further reading
English
-
STT:
- Towards an Imagenet Moment For Speech-To-Text - link
- A Speech-To-Text Practitioners Criticisms of Industry and Academia - link
- Modern Google-level STT Models Released - link
-
TTS:
- Our new public speech synthesis in super-high quality, 10x faster and more stable - link
- High-Quality Text-to-Speech Made Accessible, Simple and Fast - link
-
VAD:
- One Voice Detector to Rule Them All - link
- Modern Portable Voice Activity Detector Released - link
-
Text Enhancement:
- We have published a model for text repunctuation and recapitalization for four languages - link
Chinese
- STT:
- 迈向语音识别领域的 ImageNet 时刻 - link
- 语音领域学术界和工业界的七宗罪 - link
Russian
-
STT
- Наши сервисы для бесплатного распознавания речи стали лучше и удобнее - link
- Telegram-бот Silero бесплатно переводит речь в текст - link
- Бесплатное распознавание речи для всех желающих - link
- Последние обновления моделей распознавания речи из Silero Models - link
- Сжимаем трансформеры: простые, универсальные и прикладные способы cделать их компактными и быстрыми - link
- Ультимативное сравнение систем распознавания речи: Ashmanov, Google, Sber, Silero, Tinkoff, Yandex - link
- Мы опубликовали современные STT модели сравнимые по качеству с Google - link
- Понижаем барьеры на вход в распознавание речи - link
- Огромный открытый датасет русской речи версия 1.0 - link
- Насколько Быстрой Можно Сделать Систему STT? - link
- Наша система Speech-To-Text - link
- Speech To Text - link
-
TTS:
- Теперь наш синтез на 20 языках - link
- Теперь наш публичный синтез в супер-высоком качестве, в 10 раз быстрее и без детских болячек - link
- Синтезируем голос бабушки, дедушки и Ленина + новости нашего публичного синтеза - link
- Мы сделали наш публичный синтез речи еще лучше - link
- Мы Опубликовали Качественный, Простой, Доступный и Быстрый Синтез Речи - link
-
VAD:
- А ты используешь VAD? Что это такое и зачем он нужен - link
- Модели для Детекции Речи, Чисел и Распознавания Языков - link
- Мы опубликовали современный Voice Activity Detector и не только -link
-
Text Enhancement:
- Восстановление знаков пунктуации и заглавных букв — теперь и на длинных текстах - link
- Мы опубликовали модель, расставляющую знаки препинания и заглавные буквы в тексте на четырех языках - link
Donations
Please use the "sponsor" button.