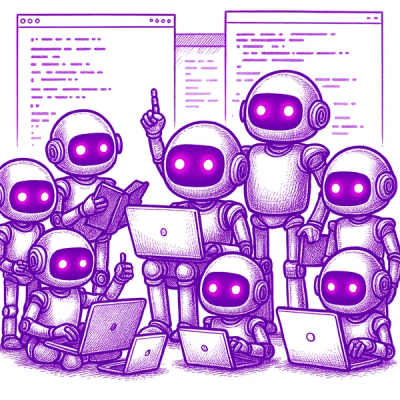
Security News
Open Source CAI Framework Handles Pen Testing Tasks up to 3,600× Faster Than Humans
CAI is a new open source AI framework that automates penetration testing tasks like scanning and exploitation up to 3,600× faster than humans.
SONAR provides a set of speech and text encoders for multilingual, multimodal semantic embedding.
We introduce SONAR, a new multilingual and multimodal fixed-size sentence embedding space, with a full suite of speech and text encoders and decoders. It substantially outperforms existing sentence embeddings such as LASER3 and LabSE on the xsim and xsim++ multilingual similarity search tasks.
Speech segments can be embedded in the same SONAR embedding space using language-specific speech encoders trained in a teacher-student setting on speech transcription data. We also provide a single text decoder, which allows us to perform text-to-text and speech-to-text machine translation, including for zero-shot language and modality combinations.
SONAR stands for Sentence-level multimOdal and laNguage-Agnostic Representations
The full list of supported languages (along with download links) can be found here below.
You can install SONAR with pip install sonar-space
. Note that there is another sonar
package on pip that IS NOT this project, make sure to use sonar-space
in your dependencies.
Note that SONAR depends on Fairseq2, which should precisely match the versions of pytorch
and CUDA
(here are the possible variants). You can check with pip show torch
which version of pytorch you gave. For example, if it equals 2.6.0+cu124
, you should install fairseq2
with from the following source:
pip install fairseq2 --extra-index-url https://fair.pkg.atmeta.com/fairseq2/whl/pt2.6.0/cu124
If fairseq2 does not provide a build for your machine, check the readme of that project to build it locally.
We recommend installing SONAR only after you have a correct version of fairseq2
installed. Note that SONAR currently relies on the stable version of fairseq2 0.4.5 (with minor variations possible).
If you want to install SONAR manually, you can install it localy:
pip install --upgrade pip
pip install -e .
fairseq2 will automatically download models into your $TORCH_HOME/hub
directory upon using the commands below.
from sonar.inference_pipelines.text import TextToEmbeddingModelPipeline
t2vec_model = TextToEmbeddingModelPipeline(encoder="text_sonar_basic_encoder",
tokenizer="text_sonar_basic_encoder")
sentences = ['My name is SONAR.', 'I can embed the sentences into vectorial space.']
embeddings = t2vec_model.predict(sentences, source_lang="eng_Latn")
print(embeddings.shape)
# torch.Size([2, 1024])
Note that by default, all SONAR models are loaded to a CPU device, which is relatively slow. If you want to use a GPU instead, you should provide the device
argument when initializing the model (this applies to every model). Similarly, you can pass a dtype
argument. For example:
import torch
from sonar.inference_pipelines.text import TextToEmbeddingModelPipeline
embedder = TextToEmbeddingModelPipeline(
encoder="text_sonar_basic_encoder",
tokenizer="text_sonar_basic_encoder",
device=torch.device("cuda"),
dtype=torch.float16,
)
from sonar.inference_pipelines.text import EmbeddingToTextModelPipeline
vec2text_model = EmbeddingToTextModelPipeline(decoder="text_sonar_basic_decoder",
tokenizer="text_sonar_basic_encoder")
reconstructed = vec2text_model.predict(embeddings, target_lang="eng_Latn", max_seq_len=512)
# max_seq_len is a keyword argument passed to the fairseq2 BeamSearchSeq2SeqGenerator.
print(reconstructed)
# ['My name is SONAR.', 'I can embed the sentences into vector space.']
By default, text generation in SONAR is based on beam search (BeamSearchSeq2SeqGenerator from fairseq2) with the default setting of beam_size=5
. If one passes a sampler
argument, we will use a SamplingSeq2SeqGenerator instead. All additional arguments are passed to the generator constructor. For example:
from fairseq2.generation import TopPSampler, TopKSampler
embeddings = t2vec_model.predict(["Bonjour le monde!"] * 10, source_lang="fra_Latn")
vec2text_model.predict(embeddings, target_lang="eng_Latn", sampler=TopPSampler(0.99), max_seq_len=128)
# ['Hello, the world!',
# 'Hey, everybody!',
# 'Good day to you, world!',
# 'Hello, the world!',
# 'Hello, people.',
# 'Hello, everybody, around the world.',
# 'Hello, world. How are you?',
# "Hey, what's up?",
# 'Good afternoon, everyone.',
# 'Hello to the world!']
# the outputs are now random, so they will be different every time
Note that the sampler
argument was a singal to use a SamplingSeq2SeqGenerator
instead of a BeamSearchSeq2SeqGenerator
, and the max_seq_len
argument was passed to the SamplingSeq2SeqGenerator
constructor.
from sonar.inference_pipelines.text import TextToTextModelPipeline
t2t_model = TextToTextModelPipeline(encoder="text_sonar_basic_encoder",
decoder="text_sonar_basic_decoder",
tokenizer="text_sonar_basic_encoder") # tokenizer is attached to both encoder and decoder cards
sentences = ['My name is SONAR.', 'I can embed the sentences into vectorial space.']
t2t_model.predict(sentences, source_lang="eng_Latn", target_lang="fra_Latn")
# ['Mon nom est SONAR.', "Je peux intégrer les phrases dans l'espace vectoriel."]
from sonar.inference_pipelines.speech import SpeechToEmbeddingModelPipeline
s2vec_model = SpeechToEmbeddingModelPipeline(encoder="sonar_speech_encoder_eng")
s2vec_model.predict(["./tests/integration_tests/data/audio_files/audio_1.wav",
"./tests/integration_tests/data/audio_files/audio_2.wav"]).shape
# torch.Size([2, 1024])
import torchaudio
inp, sr = torchaudio.load("./tests/integration_tests/data/audio_files/audio_1.wav")
assert sr == 16000, "Sample rate should be 16kHz"
s2vec_model.predict([inp]).shape
# torch.Size([1, 1024])
from sonar.inference_pipelines.speech import SpeechToTextModelPipeline
s2t_model = SpeechToTextModelPipeline(encoder="sonar_speech_encoder_eng",
decoder="text_sonar_basic_decoder",
tokenizer="text_sonar_basic_decoder")
import torchaudio
inp, sr = torchaudio.load("./tests/integration_tests/data/audio_files/audio_1.wav")
assert sr == 16000, "Sample rate should be 16kHz"
# passing loaded audio files
s2t_model.predict([inp], target_lang="eng_Latn")
# ['Television reports show white smoke coming from the plant.']
# passing multiple wav files
s2t_model.predict(["./tests/integration_tests/data/audio_files/audio_1.wav",
"./tests/integration_tests/data/audio_files/audio_2.wav"], target_lang="eng_Latn")
# ['Television reports show white smoke coming from the plant.',
# 'These couples may choose to make an adoption plan for their baby.']
BLASER 2.0 is a family of models for automatic evaluation of machine translation quality based on SONAR embeddings. They predict cross-lingual semantic similarity between the translation and the source (optionally, also using a reference translation).
from sonar.inference_pipelines.text import TextToEmbeddingModelPipeline
from sonar.models.blaser.loader import load_blaser_model
blaser_ref = load_blaser_model("blaser_2_0_ref").eval()
blaser_qe = load_blaser_model("blaser_2_0_qe").eval()
text_embedder = TextToEmbeddingModelPipeline(encoder="text_sonar_basic_encoder", tokenizer="text_sonar_basic_encoder")
src_embs = text_embedder.predict(["Le chat s'assit sur le tapis."], source_lang="fra_Latn")
ref_embs = text_embedder.predict(["The cat sat on the mat."], source_lang="eng_Latn")
mt_embs = text_embedder.predict(["The cat sat down on the carpet."], source_lang="eng_Latn")
with torch.inference_mode():
print(blaser_ref(src=src_embs, ref=ref_embs, mt=mt_embs).item()) # 4.688
print(blaser_qe(src=src_embs, mt=mt_embs).item()) # 4.708
Detailed model cards with more examples: facebook/blaser-2.0-ref, facebook/blaser-2.0-qe.
MuTox, the first highly multilingual audio-based classifier (binary) and dataset with toxicity labels. The dataset consists of 20k audio utterances for English and Spanish, and 4k for the other 19 languages, and uses the multi-model and multilingual encoders from SONAR. The output of the MuTox classifier is a logit of the evaluated being "toxic", according to the definition adopted in the corresponding dataset.
from sonar.models.mutox.loader import load_mutox_model
from sonar.inference_pipelines.text import TextToEmbeddingModelPipeline
import torch
if torch.cuda.is_available():
device = torch.device("cuda:0")
dtype = torch.float16
else:
device = torch.device("cpu")
dtype = torch.float32
t2vec_model = TextToEmbeddingModelPipeline(
encoder="text_sonar_basic_encoder",
tokenizer="text_sonar_basic_encoder",
device=device,
)
text_column='lang_txt'
classifier = load_mutox_model(
"sonar_mutox",
device=device,
dtype=dtype,
).eval()
with torch.inference_mode():
emb = t2vec_model.predict(["De peur que le pays ne se prostitue et ne se remplisse de crimes."], source_lang='fra_Latn')
x = classifier(emb.to(device).to(dtype))
print(x) # tensor([[-19.7812]], device='cuda:0', dtype=torch.float16)
with torch.inference_mode():
emb = t2vec_model.predict(["She worked hard and made a significant contribution to the team."], source_lang='eng_Latn')
x = classifier(emb.to(device).to(dtype))
print(x) # tensor([[-53.5938]], device='cuda:0', dtype=torch.float16)
with torch.inference_mode():
emb = t2vec_model.predict(["El no tiene ni el más mínimo talento, todo lo que ha logrado ha sido gracias a sobornos y manipulaciones."], source_lang='spa_Latn')
x = classifier(emb.to(device).to(dtype))
print(x) # tensor([[-21.4062]], device='cuda:0', dtype=torch.float16)
For a CLI way of running the MuTox pipeline, go to Seamless Communication/.../MuTox.
See more complete demo notebooks :
The SONAR text encoder & decoder supports 200 languages. SONAR speech encoders support 37 languages.
model | link |
---|---|
encoder | download |
decoder | download |
finetuned decoder | download |
tokenizer | download |
All 200 languages from the No Language Left Behind project are supported.
lang_code | language | link |
---|---|---|
arb | ms arabic | download |
asm | assamese | download |
bel | belarussian | download |
ben | bengali | download |
bos | bosnian | download |
bul | bulgarian | download |
cat | catalan | download |
ces | czech | download |
cmn | mandarin chinese | download |
cym | welsh | download |
dan | danish | download |
deu | german | download |
est | estonian | download |
fin | finnish | download |
fra | french | download |
guj | gujurati | download |
heb | hebrew | download |
hin | hindi | download |
hrv | croatian | download |
ind | indonesian | download |
ita | italian | download |
jpn | japanse | download |
kan | kannada | download |
kor | korean | download |
lao | lao | download |
lit | lithaian | download |
lvs | standard latvian | download |
mal | malayalam | download |
mar | marathi | download |
mkd | macedonian | download |
mlt | maltese | download |
npi | nepali | download |
nld | dutch | download |
ory | odia | download |
pan | punjabi | download |
pes | western persian | download |
pol | polish | download |
por | portuguese | download |
ron | romanian | download |
rus | russian | download |
slk | slovak | download |
slv | slovenian | download |
snd | sindhi | download |
srp | serbian | download |
spa | spanish | download |
swe | swedish | download |
swh | swahili | download |
tam | tamil | download |
tel | telugu | download |
tgl | tagalog | download |
tha | thai | download |
tur | turkish | download |
ukr | ukrainian | download |
urd | urdu | download |
uzn | northern uzbek | download |
vie | vietnamese | download |
yue | yue | download |
Please cite the paper when referencing the SONAR embedding space, encoders and decoders as:
@misc{Duquenne:2023:sonar_arxiv,
author = {Paul-Ambroise Duquenne and Holger Schwenk and Benoit Sagot},
title = {{SONAR:} Sentence-Level Multimodal and Language-Agnostic Representations},
publisher = {arXiv},
year = {2023},
url = {https://arxiv.org/abs/2308.11466},
}
See the CONTRIBUTING file for how to help out.
SONAR code is released under the MIT license (see CODE_LICENSE).
Some of SONAR models are released with the same MIT license, BUT BEWARE, some of them are released under a non commercial license (see NC_MODEL_LICENSE). Please refer to LICENSE for the details.
FAQs
SONAR provides a set of speech and text encoders for multilingual, multimodal semantic embedding.
We found that sonar-space demonstrated a healthy version release cadence and project activity because the last version was released less than a year ago. It has 4 open source maintainers collaborating on the project.
Did you know?
Socket for GitHub automatically highlights issues in each pull request and monitors the health of all your open source dependencies. Discover the contents of your packages and block harmful activity before you install or update your dependencies.
Security News
CAI is a new open source AI framework that automates penetration testing tasks like scanning and exploitation up to 3,600× faster than humans.
Security News
Deno 2.4 brings back bundling, improves dependency updates and telemetry, and makes the runtime more practical for real-world JavaScript projects.
Security News
CVEForecast.org uses machine learning to project a record-breaking surge in vulnerability disclosures in 2025.