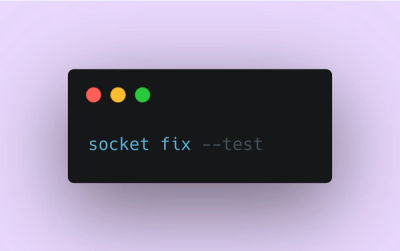
Product
Introducing Socket Fix for Safe, Automated Dependency Upgrades
Automatically fix and test dependency updates with socket fix—a new CLI tool that turns CVE alerts into safe, automated upgrades.
Welcome to SymPT, a Python package designed for performing symbolic perturbative transformations on quantum systems. SymPT leverages the Schrieffer-Wolff Transformation (SWT) and other related techniques to compute effective Hamiltonians in a systematic and efficient manner. The library offers a suite of tools for block-diagonalization, full-diagonalization, and custom transformations, supporting both time-independent and time-dependent systems.
This document provides comprehensive guidance on using SymPT, detailing its key features, algorithms, and implementation. Detailed documentation can be found here.
SymPT is a symbolic perturbative transformation tool built to help researchers and engineers study quantum systems experiencing perturbative interactions. It computes effective Hamiltonians and performs other transformations using techniques based on SWT, FD, and ACE. The package also supports multi-block diagonalizations, with special emphasis on least-action methods.
sympy
, enabling exact symbolic results for quantum systems.Make sure to install SymPT on a new python environment. To do so, you can use the Anaconda distribution platform
conda create -n sympt python
SymPT is available on PyPI for easy installation:
conda activate sympt
pip install sympt
To install SymPT from the source code, clone the repository and install dependencies:
git clone https://github.com/qcode-uni-a/sympt.git
cd SymPT
conda activate sympt
pip install .
SymPT depends on Python 3.8+ and the following libraries:
sympy
: For symbolic computations.numpy
: For matrix operations.matplotlib
: For visualizing results.RDSymbol
and RDBasis
to construct system components.SWT
, FD
, or ACE
).Here we include two example cases of how to use SymPT. Find more within the Examples folder of this project.
from sympt import RDSymbol, RDBasis, EffectiveFrame
# Define symbols
omega = RDSymbol('omega', real=True, positive=True)
g = RDSymbol('g', order=1, real=True)
# Define basis and Hamiltonian
spin = RDBasis(name='sigma', dim=2)
s0, sx, sy, sz = spin.basis
H = omega * sz
V = g * sx
# Setup EffectiveFrame
eff_frame = EffectiveFrame(H, V, subspaces=[spin])
eff_frame.solve(max_order=2, method="SW")
H_eff = eff_frame.get_H(return_form="operator")
display(H_eff)
from sympt import *
from sympy import Rational
# Define symbols
omega = RDSymbol('omega', order=0, real=True)
omega_z = RDSymbol('omega_z', order=0, real=True)
g = RDSymbol('g', order=1, real=True)
# Define basis and operators
spin = RDBasis(name='sigma', dim=2)
s0, sx, sy, sz = spin.basis
a = BosonOp('a')
ad = Dagger(a)
# Define Hamiltonian
H = omega * ad * a + Rational(1,2) * omega_z * sz + g * sx * (a + ad)
mask = Block(fin = sx, inf = a, subspaces=[spin]) # this mask is equivalent to "SW" up to second order
# Solve ACE transformation
eff_frame = EffectiveFrame(H, subspaces=[spin])
eff_frame.solve(max_order=2, method="ACE", mask=mask)
H_eff = eff_frame.get_H(return_form="operator")
display(H_eff)
omega = RDSymbol('omega', order=0, real=True)
spin = RDBasis(name='sigma', dim=2)
.solve()
: Perform the transformation..get_H()
: Retrieve the effective Hamiltonian..rotate()
: Rotate operators into the new frame.mask = Block(fin=a)
Systematically block-diagonalizes the Hamiltonian, focusing on separating diagonal and off-diagonal terms.
Fully diagonalizes the Hamiltonian, eliminating all off-diagonal elements.
Targets specific off-diagonal couplings for elimination, allowing flexible transformations.
display_dict
: Enhanced dictionary printing for Hamiltonians.group_by_operators
: Groups terms by their operator components.get_block_mask
: Simplifies block-off diagonal mask creation.We welcome contributions! Please:
SymPT is licensed under the MIT License. See LICENSE
for details.
FAQs
A solver for perturbative expansions in quantum systems
We found that sympt demonstrated a healthy version release cadence and project activity because the last version was released less than a year ago. It has 1 open source maintainer collaborating on the project.
Did you know?
Socket for GitHub automatically highlights issues in each pull request and monitors the health of all your open source dependencies. Discover the contents of your packages and block harmful activity before you install or update your dependencies.
Product
Automatically fix and test dependency updates with socket fix—a new CLI tool that turns CVE alerts into safe, automated upgrades.
Security News
CISA denies CVE funding issues amid backlash over a new CVE foundation formed by board members, raising concerns about transparency and program governance.
Product
We’re excited to announce a powerful new capability in Socket: historical data and enhanced analytics.