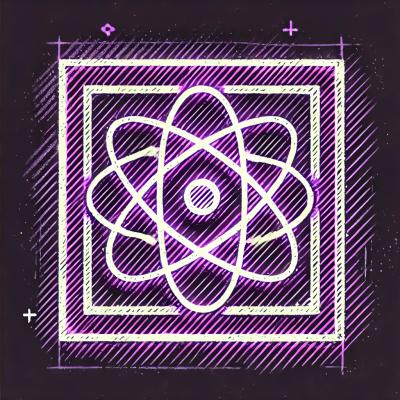
Security News
Create React App Officially Deprecated Amid React 19 Compatibility Issues
Create React App is officially deprecated due to React 19 issues and lack of maintenance—developers should switch to Vite or other modern alternatives.
sysopt
is a python3
framework for component based modelling, simulation and optimisation of continuous time dynamic and control systems.
It allows users to design modular plant and control systems, simulate the trajectory of closed loop systems, and run joint parameter/path optimisation studies.
pip install sysopt
Test problem 3 from Herber and Allison1 provides a minimal example of sysopt
usage.
First, we define some components (plant, and controller), assemble a composite model then setup a optimsation problem for that model and solve it.
from sysopt import Metadata, Composite, SolverContext, PiecewiseConstantSignal, Parameter
from sysopt.modelling.builders import FullStateOutput
from sysopt.blocks import ConstantSignal
k_star = 0.8543 # Known optimal gain.
t_f = 10
# Define the plant
def dxdt(t, x, u, p):
return [x[1], - p[0] * x[0] + u[0]]
def x0(p):
return [0, 0]
plant_metadata = Metadata(inputs=['u'], states=['x', 'v'], parameters=['k'])
plant = FullStateOutput(plant_metadata, dxdt, x0)
# Define the controller
controller = ConstantSignal(['u'], name='Controller')
# Define the Composite system via components and wires
model = Composite(name='Model', components=[plant, controller])
model.declare_outputs(['x', 'v', 'u'])
model.wires = [(controller.outputs, plant.inputs),
(plant.outputs, model.outputs[0:2]),
(controller.outputs, model.outputs[2])]
k = Parameter('k'')
u = PiecewiseConstantSignal('u', 100)
parameters = {
plant.parameters['k']: k,
controller.parameters['u']:u
}
# Setup the joint optimisation problem.
with SolverContext(model=model, t_final=t_f, parameters=parameters) as solver:
y_final = model.outputs(solver.t_final)
cost = -y_final[0]
constraints = [u <= 1, u >= -1,
y_final[1] >= 0, y_final[1] <= 0]
problem = solver.problem(arguments=[k, u],
cost=cost,
subject_to=constraints)
soln = problem.solve(guess=[0, 0])
k_min, u_min = soln.argmin
assert abs(k_min - k_star) < 1e-2
Herber, Daniel R., and James T. Allison. "Nested and simultaneous solution strategies for general combined plant and control design problems." Journal of Mechanical Design 141.1 (2019). ↩
FAQs
Component-based systems modelling library.
We found that sysopt demonstrated a healthy version release cadence and project activity because the last version was released less than a year ago. It has 1 open source maintainer collaborating on the project.
Did you know?
Socket for GitHub automatically highlights issues in each pull request and monitors the health of all your open source dependencies. Discover the contents of your packages and block harmful activity before you install or update your dependencies.
Security News
Create React App is officially deprecated due to React 19 issues and lack of maintenance—developers should switch to Vite or other modern alternatives.
Security News
Oracle seeks to dismiss fraud claims in the JavaScript trademark dispute, delaying the case and avoiding questions about its right to the name.
Security News
The Linux Foundation is warning open source developers that compliance with global sanctions is mandatory, highlighting legal risks and restrictions on contributions.