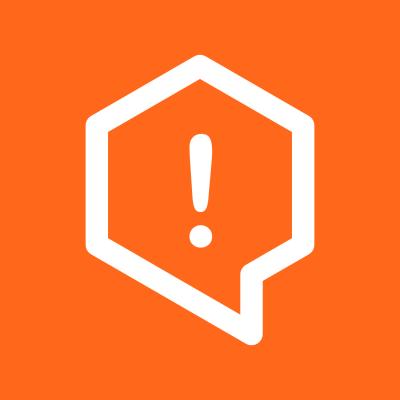
Security News
Fluent Assertions Faces Backlash After Abandoning Open Source Licensing
Fluent Assertions is facing backlash after dropping the Apache license for a commercial model, leaving users blindsided and questioning contributor rights.
Implementation of the classical and extended Short Term Objective Intelligibility in PyTorch. See also Cees Taal's website and the python implementation
pip install torch_stoi
This implementation is intended to be used as a loss function only.
It doesn't replicate the exact behavior of the original metrics
but the results should be close enough that it can be used
as a loss function. See the Notes in the
NegSTOILoss
class.
Quantitative comparison coming soon hopefully :rocket:
import torch
from torch import nn
from torch_stoi import NegSTOILoss
sample_rate = 16000
loss_func = NegSTOILoss(sample_rate=sample_rate)
# Your nnet and optimizer definition here
nnet = nn.Module()
noisy_speech = torch.randn(2, 16000)
clean_speech = torch.randn(2, 16000)
# Estimate clean speech
est_speech = nnet(noisy_speech)
# Compute loss and backward (then step etc...)
loss_batch = loss_func(est_speech, clean_speech)
loss_batch.mean().backward()
Values obtained with the NumPy version (commit 84b1bd8) are compared to the PyTorch version in the following graphs.
Classic STOI measure
Extended STOI measure
Classic STOI measure
Extended STOI measure
16kHz signals used to compare both versions contained a lot of silence, which explains why the match is very bad without VAD.
Coming in the near future
FAQs
Computes Short Term Objective Intelligibility in PyTorch
We found that torch-stoi demonstrated a healthy version release cadence and project activity because the last version was released less than a year ago. It has 1 open source maintainer collaborating on the project.
Did you know?
Socket for GitHub automatically highlights issues in each pull request and monitors the health of all your open source dependencies. Discover the contents of your packages and block harmful activity before you install or update your dependencies.
Security News
Fluent Assertions is facing backlash after dropping the Apache license for a commercial model, leaving users blindsided and questioning contributor rights.
Research
Security News
Socket researchers uncover the risks of a malicious Python package targeting Discord developers.
Security News
The UK is proposing a bold ban on ransomware payments by public entities to disrupt cybercrime, protect critical services, and lead global cybersecurity efforts.