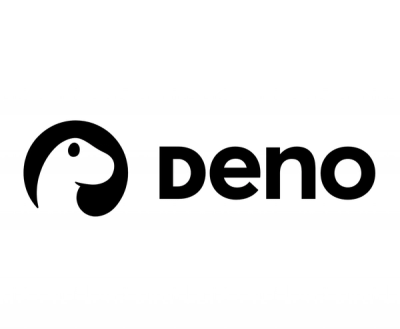
Security News
Deno 2.2 Improves Dependency Management and Expands Node.js Compatibility
Deno 2.2 enhances Node.js compatibility, improves dependency management, adds OpenTelemetry support, and expands linting and task automation for developers.
torchtuples is a small python package for training PyTorch models.
It works equally well for numpy arrays
and torch tensors
.
One of the main benefits of torchtuples is that it handles data in the form of nested tuples (see example below).
torchtuples depends on PyTorch which should be installed from HERE.
Next, torchtuples can be installed with pip:
pip install torchtuples
Or, via conda:
conda install -c conda-forge torchtuples
For the bleeding edge version, install directly from github (consider adding --force-reinstall
):
pip install git+git://github.com/havakv/torchtuples.git
or by cloning the repo:
git clone https://github.com/havakv/torchtuples.git
cd torchtuples
python setup.py install
import torch
from torch import nn
from torchtuples import Model, optim
Make a data set with three sets of covariates x0
, x1
and x2
, and a target y
.
The covariates are structured in a nested tuple x
.
n = 500
x0, x1, x2 = [torch.randn(n, 3) for _ in range(3)]
y = torch.randn(n, 1)
x = (x0, (x0, x1, x2))
Create a simple ReLU net that takes as input the tensor x_tensor
and the tuple x_tuple
. Note that x_tuple
can be of arbitrary length. The tensors in x_tuple
are passed through a layer lin_tuple
, averaged, and concatenated with x_tensor
.
We then pass our new tensor through the layer lin_cat
.
class Net(nn.Module):
def __init__(self):
super().__init__()
self.lin_tuple = nn.Linear(3, 2)
self.lin_cat = nn.Linear(5, 1)
self.relu = nn.ReLU()
def forward(self, x_tensor, x_tuple):
x = [self.relu(self.lin_tuple(xi)) for xi in x_tuple]
x = torch.stack(x).mean(0)
x = torch.cat([x, x_tensor], dim=1)
return self.lin_cat(x)
def predict(self, x_tensor, x_tuple):
x = self.forward(x_tensor, x_tuple)
return torch.sigmoid(x)
We can now fit the model with
model = Model(Net(), nn.MSELoss(), optim.SGD(0.01))
log = model.fit(x, y, batch_size=64, epochs=5)
and make predictions with either the Net.predict
method
preds = model.predict(x)
or with the Net.forward
method
preds = model.predict_net(x)
For more examples, see the examples folder.
FAQs
Training neural networks in PyTorch
We found that torchtuples demonstrated a healthy version release cadence and project activity because the last version was released less than a year ago. It has 1 open source maintainer collaborating on the project.
Did you know?
Socket for GitHub automatically highlights issues in each pull request and monitors the health of all your open source dependencies. Discover the contents of your packages and block harmful activity before you install or update your dependencies.
Security News
Deno 2.2 enhances Node.js compatibility, improves dependency management, adds OpenTelemetry support, and expands linting and task automation for developers.
Security News
React's CRA deprecation announcement sparked community criticism over framework recommendations, leading to quick updates acknowledging build tools like Vite as valid alternatives.
Security News
Ransomware payment rates hit an all-time low in 2024 as law enforcement crackdowns, stronger defenses, and shifting policies make attacks riskier and less profitable.