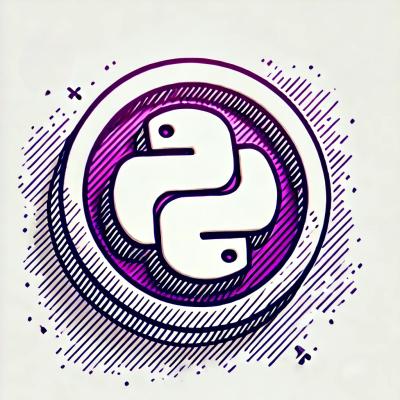
Security News
Python Adopts Standard Lock File Format for Reproducible Installs
Python has adopted a standardized lock file format to improve reproducibility, security, and tool interoperability across the packaging ecosystem.
"abroca" is a python package to provide basic functionality for computing and visualizing the Absolute Between-ROC Area (ABROCA).
The source code is currently hosted on github and Python package at PyPi
# PyPI
pip install abroca
You can find the .ipynb file under example folder. It is a basic example which demonstrates the use of the abroca
package to compute the ABROCA for a simple logistic regression classifier.
#Compute Abroca
slice = compute_abroca(df_test, pred_col = 'pred_proba' , label_col = 'returned',
protected_attr_col = 'Gender', compare_type = 'binary',
n_grid = 10000, plot_slices = True)
The plot is automatically saved to a file and is displayed on-screen. The link to download the data is given in the comments in the example file. Parameters are self explainatory through the example file. Parameter details below.
Reference Paper: Josh Gardner, Christopher Brooks, and Ryan Baker (2019). Evaluating the Fairness of Predictive Student Models Through Slicing Analysis. Proceedings of the 9th International Conference on Learning Analytics and Knowledge (LAK19); March 4-8, 2019; Tempe, AZ, USA. https://doi.org/10.1145/3303772.3303791
If you encounter a clear bug, please file a minimal reproducible example on github, or contact the package maintainers directly (see the package documentation).
FAQs
package for computing and visualizing the Absolute Between-ROC Area (ABROCA)
We found that abroca demonstrated a healthy version release cadence and project activity because the last version was released less than a year ago. It has 1 open source maintainer collaborating on the project.
Did you know?
Socket for GitHub automatically highlights issues in each pull request and monitors the health of all your open source dependencies. Discover the contents of your packages and block harmful activity before you install or update your dependencies.
Security News
Python has adopted a standardized lock file format to improve reproducibility, security, and tool interoperability across the packaging ecosystem.
Security News
OpenGrep has restored fingerprint and metavariable support in JSON and SARIF outputs, making static analysis more effective for CI/CD security automation.
Security News
Security experts warn that recent classification changes obscure the true scope of the NVD backlog as CVE volume hits all-time highs.