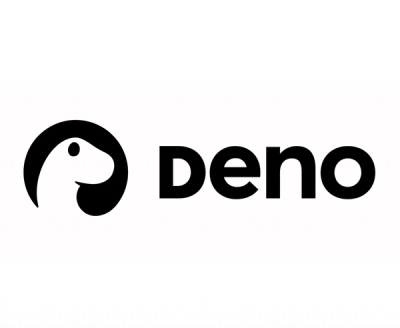
Security News
Deno 2.4 Brings Back deno bundle, Improves Dependency Management and Observability
Deno 2.4 brings back bundling, improves dependency updates and telemetry, and makes the runtime more practical for real-world JavaScript projects.
Toolkit for performing anomaly detection algorithm on 1D time series based on numpy, scipy.
Conventional approaches that based on statistical analysis have been implemented, with mainly two approaches included:
Normal Distribution
Data samples are presumably been generated by normal distribution, and therefore anomalous data points can be targeted by analysing the standard deviation.
Fitting Ansatz
Data samples are fitted by several ansatzs, and in accordance with the residual, anomalous data points can be selected.
Regarding model selections, models are adopted dynamically by performing normal test and by computing the (Akaike/Bayesian) information criterion. By default, the algorithm will first try to fit in the data into normal distribution, if it passed normal test. If this attempt suffers from the loss of convergence or it did not pass normal test from begining, then the algorithm will pass data into the second methods and try to execute all the available fitting ansatzs simultaneously. The best fitting ansatz will be selected by information criterion, and finally the algorithm will pick up anomalous points in accordance with the residual. click here to see all available methods.
Future development will also include methods that are based on deep learning techniques, such as isolation forest, support vector machine, etc.
pip install anko
For current release version please refer to PyPI - anko homepage.
For details about anko API, see the reference documentation.
Run anko_tutorial.ipynb on your local Jupyter Notebook or host on google colab.
from anko.anomaly_detector import AnomalyDetector
agent = AnomalyDetector(t, series)
agent.thres_params["linregress_res"] = 1.5
agent.apply_policies["z_normalization"] = True
agent.apply_policies["info_criterion"] = 'AIC'
for the use of AnomalyDetector.thres_params and AnomalyDetector.apply_policies, please refer to the documentation.
check_result = agent.check()
The type of output check_result is CheckResult, which is basically a dictionary that contains the following attributes:
model: 'increase_step_func'
popt: [220.3243250055105, 249.03846355234577, 74.00000107457113]
perr: [0.4247789247961187, 0.7166253174634686, 0.0]
anomalous_data: [(59, 209)]
residual: [10.050378152592119]
extra_info: ['Info: AnomalyDetector is using z normalization.', 'Info: There are more than 1 discontinuous points detected.']
python -m unittest discover -s test -p '*_test.py'
or simply
make test
FAQs
Toolkit for performing anomaly detection algorithm on time series.
We found that anko demonstrated a healthy version release cadence and project activity because the last version was released less than a year ago. It has 1 open source maintainer collaborating on the project.
Did you know?
Socket for GitHub automatically highlights issues in each pull request and monitors the health of all your open source dependencies. Discover the contents of your packages and block harmful activity before you install or update your dependencies.
Security News
Deno 2.4 brings back bundling, improves dependency updates and telemetry, and makes the runtime more practical for real-world JavaScript projects.
Security News
CVEForecast.org uses machine learning to project a record-breaking surge in vulnerability disclosures in 2025.
Security News
Browserslist-rs now uses static data to reduce binary size by over 1MB, improving memory use and performance for Rust-based frontend tools.