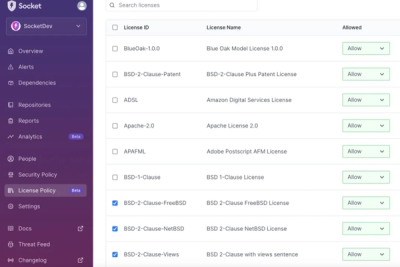
Product
Introducing License Enforcement in Socket
Ensure open-source compliance with Socket’s License Enforcement Beta. Set up your License Policy and secure your software!
Ideally, your machine has a GPU and is running Linux.
First of all, please install anaconda at $HOME/anaconda3/
. To setup the environment for this repository, please run the following commands:
git clone git@github.com:DCalhas/eeg_to_fmri.git
cd eeg_to_fmri
Download cudnn:
wget https://developer.download.nvidia.com/compute/redist/cudnn/v8.0.1/cudnn-11.0-linux-x64-v8.0.1.13.tgz
Run the configuration file:
./config.sh
Please make sure to set the path to the datasets directory correclty. This path is stored in an environment variable, so every time you activate the environment, the variable is set and used in the code as os.environ['EEG_FMRI_DATASETS'].
Testing a new dataset on this framework should not be too difficult. Do the following (in the order you feel most comfortable):
In addition to reading the rest of this section, which helps you setting up your data, you also have available two blog posts:
In this example, we assume your dataset has the following structure (if it has a different structure please interpret the code provided in the next two sections and adapt it):
NEW
| .
| ..
| README.md
└───────EEG
| └───────sub-001
| | | FILE.eeg
| | | FILE.vhdr
| | | FILE.vmrk
| └───────sub-002
| ...
└───────BOLD
└───────sub-001
| | FILE.anat
| | FILE.nii.gz
└───────sub-002
...
Ideally you want this function to return an mne.io.Raw object, that contains the EEG data. In this "tutorial" only this is the only supported option, however do it as you like most.
The inputs of this function are:
for individual in range(getattr(data_utils, "n_individuals_"+dataset)
, that goes through the range of individuals, n_individuals_NEW, you set in the data_utils.py file;path_eeg=os.environ['EEG_FMRI_DATASETS']+dataset_NEW+"/EEG/"
, this may be an optional argument set as path_eeg=os.environ['EEG_FMRI_DATASETS']+dataset_NEW+"/EEG/"
;So given these inputs one can start by listing the directories of your dataset (now this can depend on how you organized the data, we assume that each individual has a folder dedicated to itself and the sorted names of the folders have the individual's folders first and after the auxiliary description ones, e.g. "info" for information about the dataset):
def get_eeg_instance_NEW(individual, path_eeg=os.environ['EEG_FMRI_DATASETS']+dataset_NEW+"/EEG/", task=None,):
individuals = sorted([f for f in listdir(path_eeg) if isdir(join(path_eeg, f))])
individual = individuals[individual]
path=path_eeg+individual+"/"
print(path)#for debug purposes only, please remove this line after function is implemented
The output of the last print (if individuals=["sub-001", "sub-002", ..., "sub-"+data_utils.n_individuals_NEW, ...]
):
/tmp/"+dataset_NEW+"/EEG/sub-001/
Inside the path described above should be a set of files needed to load a eeg brainvision object. If you sort these files, likely
the .vhdr
is the second option:
brainvision_files = sorted([f for f in listdir(path) if isfile(join(path, f))])
vhdr_file = brainvision_files[1]
Now one only needs to return the Brainvision object:
complete_path = path + vhdr_file
return mne.io.read_raw_brainvision(complete_path, preload=True, verbose=0)
In the end get_eeg_instance_NEW is:
def get_eeg_instance_NEW(individual, path_eeg=os.environ['EEG_FMRI_DATASETS']+dataset_NEW+"/", task=None,):
individuals = sorted([f for f in listdir(path_eeg) if isdir(join(path_eeg, f))])
individual = individuals[individual]
path=path_eeg+individual+"/"
brainvision_files = sorted([f for f in listdir(path) if isfile(join(path, f))])
vhdr_file = brainvision_files[1]
complete_path = path + vhdr_file
return mne.io.read_raw_brainvision(complete_path, preload=True, verbose=0)
Next step is to implement the function that retrieves the fMRI recordings of all individuals. We assume each individual's recording is save in an .nii.gz file.
The inputs of this function are:
path_fmri=os.environ['EEG_FMRI_DATASETS']+dataset_NEW+"/BOLD/
, this may be an optional argument set as path_fmri=os.environ['EEG_FMRI_DATASETS']+dataset_NEW+"/BOLD/"
number_individuals=getattr(data_utils, "number_individuals_"+dataset)
;Given the absolute path of the data and the number of individuals one wants to retrieve, we can now start implementing the code. Let's start by listing the individuals and saving it in a list:
def get_individuals_paths_NEW(path_fmri=os.environ['EEG_FMRI_DATASETS']+dataset_NEW+"/BOLD/", resolution_factor=None, number_individuals=None):
fmri_individuals = []#this will be the output of this function
dir_individuals = sorted([f for f in listdir(path_fmri) if isdir(join(path_fmri, f)) and "sub" in path_fmri+f])
print(dir_individuals)
[os.environ['EEG_FMRI_DATASETS']+dataset_NEW+"/BOLD/sub-001", os.environ['EEG_FMRI_DATASETS']+dataset_NEW+"/BOLD/sub-002", ..., os.environ['EEG_FMRI_DATASETS']+dataset_NEW+"/BOLD/sub-"+data_utils.n_individuals_NEW, ...]
Now we can move on to start the loop, where one iterates over each individuals' directory and loads the recording:
for i in range(number_individuals):
task_file=sorted([f for f in listdir(path_fmri+dir_individuals[i]) if isfile(path_fmri+dir_individuals[i]+f) and task in path_fmri+dir_individuals[i]+f])
print(task_file)
We assume that inside the individuals' folder, you will have an ".nii.gz" file and an additional ".anat" file. When sorted this list will have the ".nii.gz" file in the second place:
[os.environ['EEG_FMRI_DATASETS']+dataset_NEW+"/BOLD/sub-001/FILE.anat", os.environ['EEG_FMRI_DATASETS']+dataset_NEW+"/BOLD/sub-001/FILE.nii.gz"]
Therefore we pick the second file and use the nilearn library to load the image:
file_path= path_fmri+dir_individuals[i]+task_file[1]
fmri_individuals += [image.load_img(file_path)]
return fmri_individuals
In the end, this function is as:
def get_individuals_paths_NEW(path_fmri=os.environ['EEG_FMRI_DATASETS']+dataset_NEW+"/BOLD/", resolution_factor=None, number_individuals=None):
fmri_individuals = []#this will be the output of this function
dir_individuals = sorted([f for f in listdir(path_fmri) if isdir(join(path_fmri, f)) and "sub" in path_fmri+f])
for i in range(number_individuals):
task_file=sorted([f for f in listdir(path_fmri+dir_individuals[i]) if isfile(path_fmri+dir_individuals[i]+f) and task in path_fmri+dir_individuals[i]+f])
file_path= path_fmri+dir_individuals[i]+task_file[1]
fmri_individuals += [image.load_img(file_path)]
return fmri_individuals
Unfortunately, the model only accepts:
For the EEG, we do not have a specified studied solution, just pray that it works.
For the fMRI, we found that mutating the resolution via Discrete Cosine Transform (DCT) spectral domain is a reasonable work around. To do this you only need to add the specified lines to the get_individuals_paths_NEW and a downsample and downsample_shape optional arguments to the function call:
def get_individuals_paths_NEW(path_fmri=os.environ['EEG_FMRI_DATASETS']+dataset_NEW+"/BOLD/", resolution_factor=None, number_individuals=None, downsample=True, downsample_shape=(64,64,30)):
...
if(downsample):
import sys
sys.path.append("..")
from layers import fft
dct=None
idct=None
...
Import the modules to perform the DCT and either add or remove resolutions to fit your data to the desired shapes. Then when you load the image, you should mutate it as:
...
fmri_individuals += [image.load_img(file_path)]
if(downsample):
img = np.swapaxes(np.swapaxes(np.swapaxes(fmri_individuals[-1].get_fdata(), 0, 3), 1,2), 1,3)
if(dct is None and idct is None):
dct = fft.DCT3D(*img.shape[1:])
idct = fft.iDCT3D(*downsample_shape)
fmri_individuals[-1] = image.new_img_like(fmri_individuals[-1],
np.swapaxes(np.swapaxes(np.swapaxes(idct(dct(img).numpy()[:, :downsample_shape[0], :downsample_shape[1], :downsample_shape[2]]).numpy(), 0, 3), 0,2), 0,1))
return fmri_individuals
After this, you should be set to run the code and retrieve the results you desire.
Now you just need to run the main.py file with your dataset identifier given as an argument. Please refer to the documentation, where you will find what you need to give as arguments, an example call is (open shell):
cd eeg_to_fmri/src
conda activate eeg_fmri
mkdir /tmp/eeg_to_fmri
mkdir /tmp/eeg_to_fmri/metrics
python main.py metrics NEW -na_path_eeg ../na_models_eeg/na_specification_2 -na_path_fmri ../na_models_fmri/na_specification_2 -save_metrics -metrics_path /tmp/eeg_to_fmri/metrics
This repository goes along with blog posts done during my PhD course:
We would like to thank everyone at INESC-ID that accompanied the journey throughout my PhD. This work was supported by national funds through Fundação para a Ciência e Tecnologia (FCT), under the Ph.D. Grant SFRH/BD/5762/2020 to David Calhas and INESC-ID pluriannual UIDB/50021/2020.
If you use this software in your work, please cite it using the following metadata:
@article{calhas2022eeg,
title={EEG to fMRI Synthesis Benefits from Attentional Graphs of Electrode Relationships},
author={Calhas, David and Henriques, Rui},
journal={arXiv preprint arXiv:2203.03481},
year={2022}
}
FAQs
Unknown package
We found that eeg-to-fmri demonstrated a healthy version release cadence and project activity because the last version was released less than a year ago. It has 1 open source maintainer collaborating on the project.
Did you know?
Socket for GitHub automatically highlights issues in each pull request and monitors the health of all your open source dependencies. Discover the contents of your packages and block harmful activity before you install or update your dependencies.
Product
Ensure open-source compliance with Socket’s License Enforcement Beta. Set up your License Policy and secure your software!
Product
We're launching a new set of license analysis and compliance features for analyzing, managing, and complying with licenses across a range of supported languages and ecosystems.
Product
We're excited to introduce Socket Optimize, a powerful CLI command to secure open source dependencies with tested, optimized package overrides.