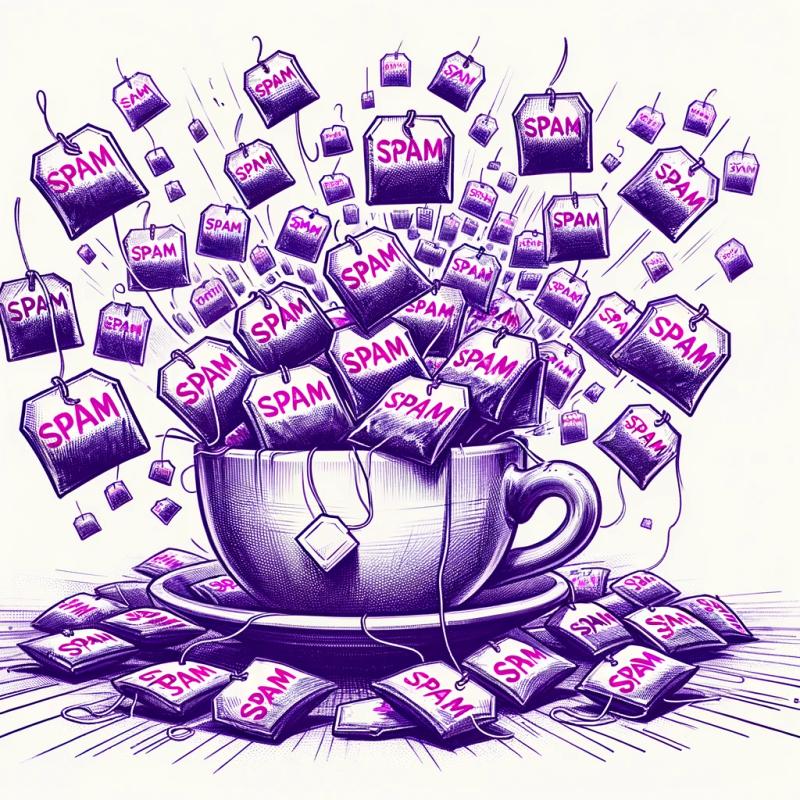
Security News
tea.xyz Spam Plagues npm and RubyGems Package Registries
Tea.xyz, a crypto project aimed at rewarding open source contributions, is once again facing backlash due to an influx of spam packages flooding public package registries.
Open-source tools to analyze, monitor, and debug machine learning model in production.
Readme
An open-source framework to evaluate, test and monitor ML models in production.
Docs | Discord | User Newsletter | Blog | Twitter | Evidently Cloud
Evidently 0.4.0. Self-host an ML Monitoring interface -> QuickStart
Evidently is an open-source Python library for data scientists and ML engineers. It helps evaluate, test, and monitor ML models from validation to production. It works with tabular, text data and embeddings.
Evidently has a modular approach with 3 components on top of the shared metrics
functionality.
Tests perform structured data and ML model checks. They verify a condition and return an explicit pass or fail.
You can create a custom Test Suite from 50+ tests or run a preset (for example, Data Drift or Regression Performance). You can get results as a JSON, Python dictionary, exportable HTML, visual report inside Jupyter notebook, or as Evidently JSON snapshot
.
Tests are best for automated checks. You can integrate them as a pipeline step using tools like Airflow.
Old dashboards API was deprecated in v0.1.59. Here is the migration guide.
Reports calculate various data and ML metrics and render rich visualizations. You can create a custom Report or run a preset to evaluate a specific aspect of the model or data performance. For example, a Data Quality or Classification Performance report.
You can get an HTML report (best for exploratory analysis and debugging), JSON or Python dictionary output (best for logging, documentation or to integrate with BI tools), or as Evidently JSON snapshot
.
This functionality is available from v0.4.0.
You can self-host an ML monitoring dashboard to visualize metrics and test results over time. This functionality sits on top of Reports and Test Suites. You must store their outputs as Evidently JSON snapshots
that serve as a data source for the Evidently Monitoring UI.
You can track 100+ metrics available in Evidently, from the number of nulls to text sentiment and embedding drift.
Evidently is available as a PyPI package. To install it using pip package manager, run:
pip install evidently
Evidently is also available on Anaconda distribution platform. To install Evidently using conda installer, run:
conda install -c conda-forge evidently
Evidently is available as a PyPI package. To install it using pip package manager, run:
pip install evidently
To install Evidently using conda installer, run:
conda install -c conda-forge evidently
This is a simple Hello World example. Head to docs for a complete Quickstart for Reports and Test Suites.
Prepare your data as two pandas DataFrames
. The first is your reference data, and the second is current production data. The structure of both datasets should be identical. You need input features only to run some evaluations (e.g., Data Drift). In other cases (e.g., Target Drift, Classification Performance), you need Target and/or Prediction.
After installing the tool, import the Evidently Test Suite and required presets. We'll use a simple toy dataset:
import pandas as pd
from sklearn import datasets
from evidently.test_suite import TestSuite
from evidently.test_preset import DataStabilityTestPreset
from evidently.test_preset import DataQualityTestPreset
iris_data = datasets.load_iris(as_frame='auto')
iris_frame = iris_data.frame
To run the Data Stability Test Suite and display the output in the notebook:
data_stability= TestSuite(tests=[
DataStabilityTestPreset(),
])
data_stability.run(current_data=iris_frame.iloc[:60], reference_data=iris_frame.iloc[60:], column_mapping=None)
data_stability
You can also save an HTML file. You'll need to open it from the destination folder.
data_stability.save_html("file.html")
To get the output as JSON:
data_stability.json()
After installing the tool, import the Evidently Report and required presets:
import pandas as pd
from sklearn import datasets
from evidently.report import Report
from evidently.metric_preset import DataDriftPreset
iris_data = datasets.load_iris(as_frame='auto')
iris_frame = iris_data.frame
To generate the Data Drift report, run:
data_drift_report = Report(metrics=[
DataDriftPreset(),
])
data_drift_report.run(current_data=iris_frame.iloc[:60], reference_data=iris_frame.iloc[60:], column_mapping=None)
data_drift_report
Save the report as HTML. You'll later need to open it from the destination folder.
data_drift_report.save_html("file.html")
To get the output as JSON:
data_drift_report.json()
This will launch a demo project in the Evidently UI. Head to docs for a complete ML Monitoring Quickstart.
Recommended step: create a virtual environment and activate it.
pip install virtualenv
virtualenv venv
source venv/bin/activate
After installing Evidently (pip install evidently
), run the Evidently UI with the demo projects:
evidently ui --demo-projects all
Access Evidently UI service in your browser. Go to the localhost:8000.
We welcome contributions! Read the Guide to learn more.
For more information, refer to a complete Documentation. You can start with the tutorials:
Simple examples on toy datasets to quickly explore what Evidently can do out of the box.
Title | Code example | Tutorial | Contents |
---|---|---|---|
QuickStart Tutorial: ML Monitoring | Example | Tutorial | Pre-built ML monitoring dashboard. |
QuickStart Tutorial: Tests and Reports | Jupyter notebook Colab | Tutorial | Data Stability and custom Test Suites, Data Drift and Target Drift Reports |
Evidently Metric Presets | Jupyter notebook Colab | - | Data Drift, Target Drift, Data Quality, Regression, Classification Reports |
Evidently Metrics | Jupyter notebook Colab | - | All individual Metrics |
Evidently Test Presets | Jupyter notebook Colab | - | NoTargetPerformance, Data Stability, Data Quality, Data Drift Regression, Multi-class Classification, Binary Classification, Binary Classification top-K test suites |
Evidently Tests | Jupyter notebook Colab | - | All individual Tests |
There are more example in the Community Examples repository.
Explore Integrations to see how to integrate Evidently in the prediction pipelines and with other tools.
Explore the How-to guides to understand specific features in Evidently, such as working with text data.
To get updates on new features, integrations and code tutorials, sign up for the Evidently User Newsletter.
If you want to chat and connect, join our Discord community!
FAQs
Open-source tools to analyze, monitor, and debug machine learning model in production.
We found that evidently demonstrated a healthy version release cadence and project activity because the last version was released less than a year ago. It has 2 open source maintainers collaborating on the project.
Did you know?
Socket for GitHub automatically highlights issues in each pull request and monitors the health of all your open source dependencies. Discover the contents of your packages and block harmful activity before you install or update your dependencies.
Security News
Tea.xyz, a crypto project aimed at rewarding open source contributions, is once again facing backlash due to an influx of spam packages flooding public package registries.
Security News
As cyber threats become more autonomous, AI-powered defenses are crucial for businesses to stay ahead of attackers who can exploit software vulnerabilities at scale.
Security News
UnitedHealth Group disclosed that the ransomware attack on Change Healthcare compromised protected health information for millions in the U.S., with estimated costs to the company expected to reach $1 billion.