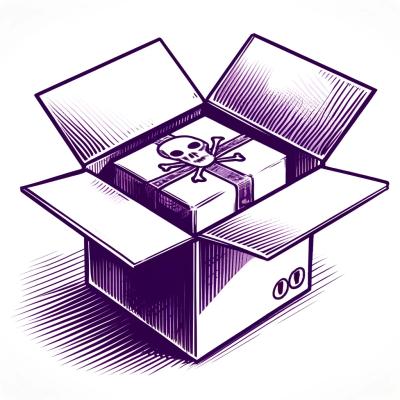
Security News
Research
Data Theft Repackaged: A Case Study in Malicious Wrapper Packages on npm
The Socket Research Team breaks down a malicious wrapper package that uses obfuscation to harvest credentials and exfiltrate sensitive data.
Tutorials | API Documentation | Chat with us on slack! |
---|---|---|
Fugue is a unified interface for distributed computing that lets users execute Python, Pandas, and SQL code on Spark, Dask, and Ray with minimal rewrites.
Fugue is most commonly used for:
To see how Fugue compares to other frameworks like dbt, Arrow, Ibis, PySpark Pandas, see the comparisons
The Fugue API is a collection of functions that are capable of running on Pandas, Spark, Dask, and Ray. The simplest way to use Fugue is the transform()
function. This lets users parallelize the execution of a single function by bringing it to Spark, Dask, or Ray. In the example below, the map_letter_to_food()
function takes in a mapping and applies it on a column. This is just Pandas and Python so far (without Fugue).
import pandas as pd
from typing import Dict
input_df = pd.DataFrame({"id":[0,1,2], "value": (["A", "B", "C"])})
map_dict = {"A": "Apple", "B": "Banana", "C": "Carrot"}
def map_letter_to_food(df: pd.DataFrame, mapping: Dict[str, str]) -> pd.DataFrame:
df["value"] = df["value"].map(mapping)
return df
Now, the map_letter_to_food()
function is brought to the Spark execution engine by invoking the transform()
function of Fugue. The output schema
and params
are passed to the transform()
call. The schema
is needed because it's a requirement for distributed frameworks. A schema of "*"
below means all input columns are in the output.
from pyspark.sql import SparkSession
from fugue import transform
spark = SparkSession.builder.getOrCreate()
sdf = spark.createDataFrame(input_df)
out = transform(sdf,
map_letter_to_food,
schema="*",
params=dict(mapping=map_dict),
)
# out is a Spark DataFrame
out.show()
+---+------+
| id| value|
+---+------+
| 0| Apple|
| 1|Banana|
| 2|Carrot|
+---+------+
from typing import Iterator, Union
from pyspark.sql.types import StructType
from pyspark.sql import DataFrame, SparkSession
spark_session = SparkSession.builder.getOrCreate()
def mapping_wrapper(dfs: Iterator[pd.DataFrame], mapping):
for df in dfs:
yield map_letter_to_food(df, mapping)
def run_map_letter_to_food(input_df: Union[DataFrame, pd.DataFrame], mapping):
# conversion
if isinstance(input_df, pd.DataFrame):
sdf = spark_session.createDataFrame(input_df.copy())
else:
sdf = input_df.copy()
schema = StructType(list(sdf.schema.fields))
return sdf.mapInPandas(lambda dfs: mapping_wrapper(dfs, mapping),
schema=schema)
result = run_map_letter_to_food(input_df, map_dict)
result.show()
This syntax is simpler, cleaner, and more maintainable than the PySpark equivalent. At the same time, no edits were made to the original Pandas-based function to bring it to Spark. It is still usable on Pandas DataFrames. Fugue transform()
also supports Dask and Ray as execution engines alongside the default Pandas-based engine.
The Fugue API has a broader collection of functions that are also compatible with Spark, Dask, and Ray. For example, we can use load()
and save()
to create an end-to-end workflow compatible with Spark, Dask, and Ray. For the full list of functions, see the Top Level API
import fugue.api as fa
def run(engine=None):
with fa.engine_context(engine):
df = fa.load("/path/to/file.parquet")
out = fa.transform(df, map_letter_to_food, schema="*")
fa.save(out, "/path/to/output_file.parquet")
run() # runs on Pandas
run(engine="spark") # runs on Spark
run(engine="dask") # runs on Dask
All functions underneath the context will run on the specified backend. This makes it easy to toggle between local execution, and distributed execution.
FugueSQL is a SQL-based language capable of expressing end-to-end data workflows on top of Pandas, Spark, and Dask. The map_letter_to_food()
function above is used in the SQL expression below. This is how to use a Python-defined function along with the standard SQL SELECT
statement.
from fugue.api import fugue_sql
import json
query = """
SELECT id, value
FROM input_df
TRANSFORM USING map_letter_to_food(mapping={{mapping}}) SCHEMA *
"""
map_dict_str = json.dumps(map_dict)
# returns Pandas DataFrame
fugue_sql(query,mapping=map_dict_str)
# returns Spark DataFrame
fugue_sql(query, mapping=map_dict_str, engine="spark")
Fugue can be installed through pip or conda. For example:
pip install fugue
In order to use Fugue SQL, it is strongly recommended to install the sql
extra:
pip install fugue[sql]
It also has the following installation extras:
For example a common use case is:
pip install "fugue[duckdb,spark]"
Note if you already installed Spark or DuckDB independently, Fugue is able to automatically use them without installing the extras.
The best way to get started with Fugue is to work through the 10 minute tutorials:
For the top level API, see:
The tutorials can also be run in an interactive notebook environment through binder or Docker:
Note it runs slow on binder because the machine on binder isn't powerful enough for a distributed framework such as Spark. Parallel executions can become sequential, so some of the performance comparison examples will not give you the correct numbers.
Alternatively, you should get decent performance by running this Docker image on your own machine:
docker run -p 8888:8888 fugueproject/tutorials:latest
There is an accompanying notebook extension for FugueSQL that lets users use the %%fsql
cell magic. The extension also provides syntax highlighting for FugueSQL cells. It works for both classic notebook and Jupyter Lab. More details can be found in the installation instructions.
By being an abstraction layer, Fugue can be used with a lot of other open-source projects seamlessly.
Python backends:
FugueSQL backends:
Fugue is available as a backend or can integrate with the following projects:
Registered 3rd party extensions (majorly for Fugue SQL) include:
Feel free to message us on Slack. We also have contributing instructions.
View some of our latest conferences presentations and content. For a more complete list, check the Content page in the tutorials.
FAQs
An abstraction layer for distributed computation
We found that fugue demonstrated a healthy version release cadence and project activity because the last version was released less than a year ago. It has 2 open source maintainers collaborating on the project.
Did you know?
Socket for GitHub automatically highlights issues in each pull request and monitors the health of all your open source dependencies. Discover the contents of your packages and block harmful activity before you install or update your dependencies.
Security News
Research
The Socket Research Team breaks down a malicious wrapper package that uses obfuscation to harvest credentials and exfiltrate sensitive data.
Research
Security News
Attackers used a malicious npm package typosquatting a popular ESLint plugin to steal sensitive data, execute commands, and exploit developer systems.
Security News
The Ultralytics' PyPI Package was compromised four times in one weekend through GitHub Actions cache poisoning and failure to rotate previously compromised API tokens.