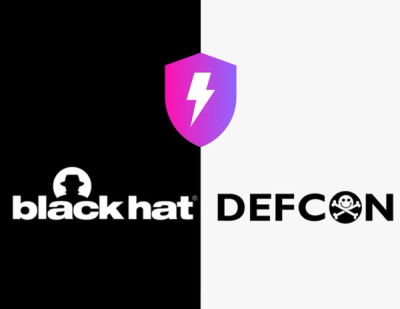
Security News
Meet Socket at Black Hat and DEF CON 2025 in Las Vegas
Meet Socket at Black Hat & DEF CON 2025 for 1:1s, insider security talks at Allegiant Stadium, and a private dinner with top minds in software supply chain security.
Optimize you Theano Models with Adagrad, Hessian Free optimization, or linear updates.
pip3 install gradient-optimizers
See example notebook (TBD) for tutorial.
Two classes GradientModel, and GradientHFModel, for optimizing gradient based models (specifically built with indexed parameters in mind (e.g. for language models))
A gradient model for updating your model with hessian free, adagrad, or linear decay updates.
You will need to define the following attributes, and fill them as appropriate:
# a forward method for getting errors:
projection = self.projection_function(ivector <indices/>)
# a cost function (that takes the result of projection function and labels as input)
# and returns a symbolic differentiable theano variable
self.cost_function(projection, ivector <label/>).sum()
self.params = []
self.indexed_params = set()
self._l2_regularization = True / False
self.store_max_updates = True / False
# set this theano setting
self.theano_mode = "FAST_RUN"
# set this theano setting
self.disconnected_inputs = 'ignore' / None
# if L2 is true store this parameter:
self._l2_regularization_parameter = theano.shared(np.float64(l2_regularization).astype(REAL), name='l2_regularization_parameter')
Upon initialization you must run:
self._select_update_mechanism(update_method_name)
# then to compile this mechanism:
self.create_update_fun()
The update methods expect the input to be of the form:
ivector <indices/>, ivector <labels/>
If this is not the case you can modify them as appropriate.
Implements an symbolic one step of hessian-free [1] optimization that approximates the curvature, requires a _compute_cost method that takes an example as input or a _compute_cost_gradients that returns gradients for each example provided.
Model should have a params property containing symbolic theano variables.
[1] James Martens, ``Deep learning via Hessian-free optimization", ICML 2010
Make sure the following parameters are not tampered with:
self._additional_params
self._num_updates
FAQs
Python package for wrapping gradient optimizers for models in Theano
We found that gradient-optimizers demonstrated a healthy version release cadence and project activity because the last version was released less than a year ago. It has 1 open source maintainer collaborating on the project.
Did you know?
Socket for GitHub automatically highlights issues in each pull request and monitors the health of all your open source dependencies. Discover the contents of your packages and block harmful activity before you install or update your dependencies.
Security News
Meet Socket at Black Hat & DEF CON 2025 for 1:1s, insider security talks at Allegiant Stadium, and a private dinner with top minds in software supply chain security.
Security News
CAI is a new open source AI framework that automates penetration testing tasks like scanning and exploitation up to 3,600× faster than humans.
Security News
Deno 2.4 brings back bundling, improves dependency updates and telemetry, and makes the runtime more practical for real-world JavaScript projects.