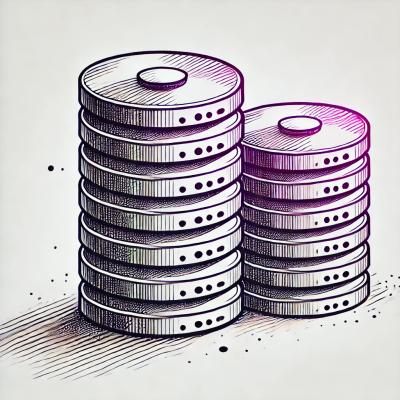
Security News
MCP Community Begins Work on Official MCP Metaregistry
The MCP community is launching an official registry to standardize AI tool discovery and let agents dynamically find and install MCP servers.
permutation-feature-selector
Advanced tools
A package for calculating permutation importance and selecting features.
This library is inspired by the functionality and design of Scikit-learn's permutation importance.
PyPI:https://pypi.org/project/permutation_feature_selector/
To install the Library,
You can install the package directly from PyPI using pip. This is the easiest and most common method:
$ pip install permutation_feature_selector
If you prefer to install from the source or if you want the latest version that may not yet be released on PyPI, you can use the following commands:
$ git clone https://github.com/Itsuki-2822/permutation_feature_selector.git
$ cd permutation_feature_selector
$ python setup.py install
If you are a contributor or if you want to install the latest development version of the library, use the following command to install directly from the GitHub repository:
$ pip install --upgrade git+https://github.com/Itsuki-2822/permutation_feature_selector
The calculation of permutation importance proceeds through the following steps:
Permutation importance is independent of the internal mechanisms of any specific model, which means it does not rely on the evaluation mechanisms specific to models like gradient boosting or decision trees. It can be applied across various predictive models (linear models, decision trees, neural networks, etc.)
import numpy as np
import pandas as pd
from sklearn.model_selection import train_test_split
from sklearn.datasets import load_diabetes
import lightgbm as lgb
import matplotlib.pyplot as plt
from permutation_feature_selector import PermutationFeatureSelector
data = load_diabetes()
X = pd.DataFrame(data.data, columns=data.feature_names)
y = data.target
X_train, X_test, y_train, y_test = train_test_split(X, y, test_size=0.2, random_state=42)
model = lgb.LGBMRegressor()
model.fit(X_train, y_train)
selector = PermutationFeatureSelector(model, X_test, y_test, metric='rmse', n_repeats=30, random_state=42)
# Calculation of permutation importance
perm_importance = selector.calculate_permutation_importance()
print(perm_importance)
# Permutation Importance Plot
selector.plot_permutation_importance()
# Feature selection (e.g., select features with importance at least 1x the average)
chosen_features, chosen_features_df = selector.choose_feat(threshold_method='mean', threshold_value=1.0)
print(chosen_features)
print(chosen_features_df)
FAQs
A package for calculating permutation importance and selecting features.
We found that permutation-feature-selector demonstrated a healthy version release cadence and project activity because the last version was released less than a year ago. It has 1 open source maintainer collaborating on the project.
Did you know?
Socket for GitHub automatically highlights issues in each pull request and monitors the health of all your open source dependencies. Discover the contents of your packages and block harmful activity before you install or update your dependencies.
Security News
The MCP community is launching an official registry to standardize AI tool discovery and let agents dynamically find and install MCP servers.
Research
Security News
Socket uncovers an npm Trojan stealing crypto wallets and BullX credentials via obfuscated code and Telegram exfiltration.
Research
Security News
Malicious npm packages posing as developer tools target macOS Cursor IDE users, stealing credentials and modifying files to gain persistent backdoor access.