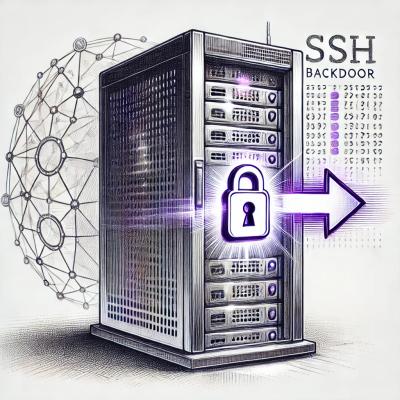
Research
Security News
Malicious npm Packages Inject SSH Backdoors via Typosquatted Libraries
Socket’s threat research team has detected six malicious npm packages typosquatting popular libraries to insert SSH backdoors.
PyTorch Extension Library for organizing tensors in a form of a structured tree of dataclasses, with built-in support for advanced collating mechanisms
PyTorch Extension Library for organizing tensors in a form of a structured tree of dataclasses, with built-in support for advanced collating mechanisms. The batch creation process seamlessly solves issues like: sequences padding, un/flattening variable #objects per example into a single batch dimension, fixing within-example indices to be batch-based indices, auto-creation of sequences & collate masks, and more.
... variable number of sequences per example where the sequence lengths may also be variable; lots of inputs usually gets messy - hard to handle, to name, to move to GPU, to abstract in a (X,Y) fashion ...
pip install tensors-data-class
# TODO: simplify the below example. still use these:
# BatchFlattenedSeq, BatchFlattenedTensor,
# BatchedFlattenedIndicesFlattenedTensor,
# BatchedFlattenedIndicesFlattenedSeq,
# BatchedFlattenedIndicesPseudoRandomPermutationBatchedFlattenedIndicesPseudoRandomPermutation,
# BatchFlattenedPseudoRandomSamplerFromRange
from tensors_data_class import *
@dataclasses.dataclass
class CodeExpressionTokensSequenceInputTensors(TensorsDataClass):
token_type: BatchFlattenedSeq # (nr_expressions_in_batch, batch_max_nr_tokens_in_expr)
kos_token_index: BatchFlattenedTensor # (nr_kos_tokens_in_all_expressions_in_batch,)
identifier_index: BatchedFlattenedIndicesFlattenedTensor # (nr_identifier_tokens_in_all_expressions_in_batch,)
@dataclasses.dataclass
class SymbolsInputTensors(TensorsDataClass):
symbols_identifier_indices: BatchedFlattenedIndicesFlattenedTensor # (nr_symbols_in_batch,); value meaning: identifier batched index
symbols_appearances_symbol_idx: BatchedFlattenedIndicesFlattenedTensor # (nr_symbols_appearances,);
symbols_appearances_expression_token_idx: BatchFlattenedTensor = None # (nr_symbols_appearances,);
symbols_appearances_cfg_expression_idx: BatchedFlattenedIndicesFlattenedTensor = None # (nr_symbols_appearances,);
@dataclasses.dataclass
class CFGPathsInputTensors(TensorsDataClass):
nodes_indices: BatchedFlattenedIndicesFlattenedSeq
edges_types: BatchFlattenedSeq
@dataclasses.dataclass
class CFGPathsNGramsInputTensors(TensorsDataClass):
nodes_indices: BatchedFlattenedIndicesFlattenedSeq
edges_types: BatchFlattenedSeq
@dataclasses.dataclass
class PDGInputTensors(TensorsDataClass):
cfg_nodes_control_kind: Optional[BatchFlattenedTensor] = None # (nr_cfg_nodes_in_batch, )
cfg_nodes_has_expression_mask: Optional[BatchFlattenedTensor] = None # (nr_cfg_nodes_in_batch, )
cfg_nodes_tokenized_expressions: Optional[CodeExpressionTokensSequenceInputTensors] = None
cfg_nodes_random_permutation: Optional[BatchedFlattenedIndicesPseudoRandomPermutation] = None
cfg_control_flow_paths: Optional[CFGPathsInputTensors] = None
cfg_control_flow_paths_ngrams: Optional[Dict[int, CFGPathsNGramsInputTensors]] = None
@dataclasses.dataclass
class IdentifiersInputTensors(TensorsDataClass):
sub_parts_batch: BatchFlattenedTensor # (nr_sub_parts_in_batch, )
identifier_sub_parts_index: BatchedFlattenedIndicesFlattenedSeq # (nr_identifiers_in_batch, batch_max_nr_sub_parts_in_identifier)
identifier_sub_parts_vocab_word_index: BatchFlattenedSeq # (nr_identifiers_in_batch, batch_max_nr_sub_parts_in_identifier)
identifier_sub_parts_hashings: BatchFlattenedSeq # (nr_identifiers_in_batch, batch_max_nr_sub_parts_in_identifier, nr_hashing_features)
sub_parts_obfuscation: BatchFlattenedPseudoRandomSamplerFromRange # (nr_sub_parts_obfuscation_embeddings)
@dataclasses.dataclass
class MethodCodeInputTensors(TensorsDataClass):
example_hash: str
identifiers: IdentifiersInputTensors
symbols: SymbolsInputTensors
method_tokenized_code: Optional[CodeExpressionTokensSequenceInputTensors] = None
pdg: Optional[PDGInputTensors] = None
example1 = MethodCodeInputTensors(...) # TODO: fill example data
example2 = MethodCodeInputTensors(...) # TODO: fill example data
batch = MethodCodeInputTensors.collate([example1, example2])
print(batch)
# TODO: add example for creating a padded-sequence (after applying embedding on the input), unflattening.
FAQs
PyTorch Extension Library for organizing tensors in a form of a structured tree of dataclasses, with built-in support for advanced collating mechanisms
We found that tensors-data-class demonstrated a healthy version release cadence and project activity because the last version was released less than a year ago. It has 1 open source maintainer collaborating on the project.
Did you know?
Socket for GitHub automatically highlights issues in each pull request and monitors the health of all your open source dependencies. Discover the contents of your packages and block harmful activity before you install or update your dependencies.
Research
Security News
Socket’s threat research team has detected six malicious npm packages typosquatting popular libraries to insert SSH backdoors.
Security News
MITRE's 2024 CWE Top 25 highlights critical software vulnerabilities like XSS, SQL Injection, and CSRF, reflecting shifts due to a refined ranking methodology.
Security News
In this segment of the Risky Business podcast, Feross Aboukhadijeh and Patrick Gray discuss the challenges of tracking malware discovered in open source softare.