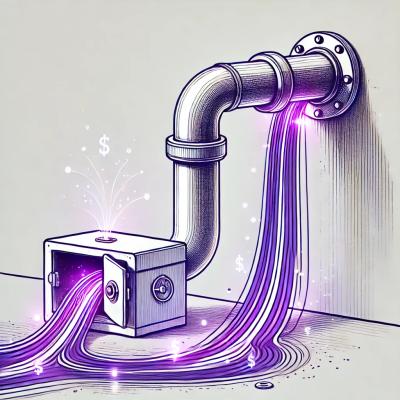
Research
Security News
Malicious npm Package Targets Solana Developers and Hijacks Funds
A malicious npm package targets Solana developers, rerouting funds in 2% of transactions to a hardcoded address.
github.com/RadiusNetworks/lda
Linear Discriminant Analysis is a powerful and commonly used dimensionality reduction and classification technique used in statistics and machine learning. It extracts components from an input dataset in a way that maximizes class separability while minimizing the variance within each class. A key assumption here is that the dataset needs to be normally distributed. In the world of machine learning, LDA can be used as a classifier algorithm, which this library also provides.
Import "github.com/RadiusNetworks/lda"
math
sort
gonum.org/v1/gonum/mat
The library contains a predefined struct LD
that can be used to access LDA methods.
Important note about input data: LDA is a supervised learning technique so all training data needs to be labeled.
The first step would be to make a variable of type LD
and call LinearDiscriminant
by passing in a matrix of input data and an array of labels that correspond to the data. If that call is successful, then you can use other LDA methods, such as Transform
and Predict
.
Example: Iris dataset
// Load your data
// See lda_test.go for an example of loading and pre-processing data
// Create a matrix and fill it with iris data
dataMatrix := mat.NewDense(numberOfRows, numberofColumns, yourDataset)
// Create an array ([]int) of labels for your dataset
var labels []int
for yourLabel := range labelsFromYourDataset {
labels = append(labels, yourLabel)
}
// Instantiate an LD object and call LinearDiscriminant to fit the model and check if input data follows preconditions in the process
var ld LDA.LD
err := ld.LinearDiscriminant(dataMatrix, labels)
if err == nil {
// If the call is successful, you can now use other methods
numDimensions := 2 // number of dimensions to reduce to
result := ld.Transform(dataMatrix, numDimensions)
// We can graph the result of the transformation on an XY plane
PlotLDA(result, labels, "LDA Plot.png")
// We can use the result of the transformation to classify test data
// *See section on method Predict below*
} else {
// handle error
}
...
Predict
Method Predict
takes in a set of data and returns a number (Int), a prediction for what class the set of data would be in. Below is an example of a test that checks if Predict is classifying data correctly.
Example: Iris test data
(See lda_test.go for the complete implementation of this example)
// Create test cases with test data and corresponding labels (classes that you expect the data points to be in)
// Call LinearDiscriminant with the test data and the labels as arguments
// Call Transform
result := ld.Transform(test.data, numDims)
r, _ := test.testPredict.Dims()
for k := 0; k < r; k++ {
c, _ := ld.Predict(test.testPredict.RawRowView(k))
if c != test.wantClass[k] {
t.Errorf("unexpected prediction result %v got:%v, want:%v", k, c, test.wantClass[k])
}
}
values := make([]string, ld.p)
for j := 0; j < ld.n; j++ {
row := result.RawRowView(j)
for k := 0; k < numDims; k++ {
values[k] = fmt.Sprintf("%.4f", row[k])
}
}
}
}
We provide a sample test file that tests both the dimensionality reduction and the classification features of the algorithm. The test uses the famous Iris dataset, which can be found here: https://archive.ics.uci.edu/ml/datasets/Iris
The implementation of the LDA algorithm is based on a Java version provided by https://github.com/haifengl/smile
Additional resources used: https://sebastianraschka.com/Articles/2014_python_lda.html
Created by Andrei Kozyrev (@akozyrev) with the help of Tim Judkins (@b0tn3rd) and Eleanor Nuechterlein (@Eleanor2). Open sourced by Radius Networks.
LDA-go is Apache-2.0 licensed. Contributions are welcome.
FAQs
Unknown package
Did you know?
Socket for GitHub automatically highlights issues in each pull request and monitors the health of all your open source dependencies. Discover the contents of your packages and block harmful activity before you install or update your dependencies.
Research
Security News
A malicious npm package targets Solana developers, rerouting funds in 2% of transactions to a hardcoded address.
Security News
Research
Socket researchers have discovered malicious npm packages targeting crypto developers, stealing credentials and wallet data using spyware delivered through typosquats of popular cryptographic libraries.
Security News
Socket's package search now displays weekly downloads for npm packages, helping developers quickly assess popularity and make more informed decisions.