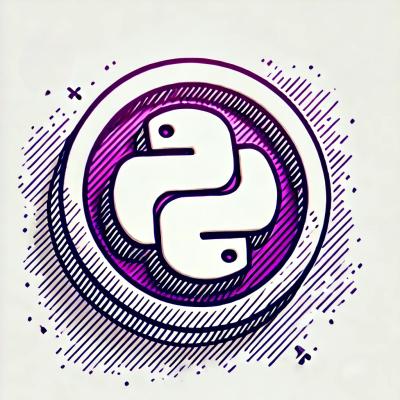
Security News
PyPI Now Supports iOS and Android Wheels for Mobile Python Development
PyPI now supports iOS and Android wheels, making it easier for Python developers to distribute mobile packages.
@precise-finance/sagemaker
Advanced tools
This package provides utilities for training and deploying machine learning models on AWS SageMaker. It supports multiple ML frameworks including PyTorch, TensorFlow, XGBoost, Scikit-learn, and HuggingFace.
This package provides utilities for training and deploying machine learning models on AWS SageMaker. It supports multiple ML frameworks including PyTorch, TensorFlow, XGBoost, Scikit-learn, and HuggingFace.
To install the package, run:
npm install @aws-sdk/client-sagemaker @aws-sdk/client-s3 @aws-sdk/lib-storage archiver
To train a model using SageMaker, you can use the provided classes for each framework. Below are examples for PyTorch and TensorFlow.
import { PyTorchTraining } from './sagemaker-framework-extensions';
import { Logger } from './interfaces';
// Configuration for AWS and SageMaker
const config = {
region: 'us-west-2',
credentials: {
accessKeyId: 'your-access-key-id',
secretAccessKey: 'your-secret-access-key',
},
bucket: 'your-s3-bucket',
role: 'your-sagemaker-role',
service: 'your-service',
model: 'your-model',
};
// Logger for logging messages
const logger: Logger = console;
// Directory containing your training script and other source files
const sourceDir = './path-to-your-source-code';
// Create an instance of PyTorchTraining
const pytorchTraining = new PyTorchTraining(config, sourceDir, logger);
// Framework-specific configuration
const frameworkConfig = {
frameworkVersion: '2.1',
pythonVersion: 'py310',
imageUri: 'your-custom-image-uri', // Optional
};
// Resource configuration for the training job
const resourceConfig = {
instanceCount: 1,
instanceType: 'ml.p3.2xlarge',
volumeSizeGB: 50,
};
// Hyperparameters for the training job
const hyperParameters = {
learningRate: 0.001,
batchSize: 32,
epochs: 10,
};
// Example 1: Using S3 path for input data
const inputDataS3 = {
data: 's3://your-bucket/path-to-your-data',
format: 'application/json',
};
async function trainModel() {
try {
// Start the training job
const metadata = await pytorchTraining.train(
frameworkConfig,
resourceConfig,
hyperParameters,
inputDataS3,
[],
true
);
console.log('Training completed:', metadata);
// Get the training job name from the metadata
const trainingJobName = metadata.trainingJobName;
console.log('Training job name:', trainingJobName);
} catch (error) {
console.error('Training failed:', error);
}
}
trainModel();
import { TensorFlowTraining } from './sagemaker-framework-extensions';
import { Logger } from './interfaces';
// Configuration for AWS and SageMaker
const config = {
region: 'us-west-2',
credentials: {
accessKeyId: 'your-access-key-id',
secretAccessKey: 'your-secret-access-key',
},
bucket: 'your-s3-bucket',
role: 'your-sagemaker-role',
service: 'your-service',
model: 'your-model',
};
// Logger for logging messages
const logger: Logger = console;
// Directory containing your training script and other source files
const sourceDir = './path-to-your-source-code';
// Create an instance of TensorFlowTraining
const tensorflowTraining = new TensorFlowTraining(config, sourceDir, logger);
// Framework-specific configuration
const frameworkConfig = {
frameworkVersion: '2.12',
pythonVersion: 'py310',
imageUri: 'your-custom-image-uri', // Optional
};
// Resource configuration for the training job
const resourceConfig = {
instanceCount: 1,
instanceType: 'ml.p3.2xlarge',
volumeSizeGB: 50,
};
// Hyperparameters for the training job
const hyperParameters = {
learningRate: 0.001,
batchSize: 32,
epochs: 10,
};
// Example 1: Using S3 path for input data
const inputDataS3 = {
data: 's3://your-bucket/path-to-your-data',
format: 'application/json',
};
async function trainModel() {
try {
// Start the training job
const metadata = await tensorflowTraining.train(
frameworkConfig,
resourceConfig,
hyperParameters,
inputDataS3,
[],
true
);
console.log('Training completed:', metadata);
// Get the training job name from the metadata
const trainingJobName = metadata.trainingJobName;
console.log('Training job name:', trainingJobName);
} catch (error) {
console.error('Training failed:', error);
}
}
trainModel();
To deploy a trained model using SageMaker, you can use the provided classes for each framework. Below are examples for PyTorch and TensorFlow.
import { PyTorchDeployment } from './deploy';
import { Logger } from './interfaces';
// Configuration for AWS and SageMaker
const config = {
region: 'us-west-2',
credentials: {
accessKeyId: 'your-access-key-id',
secretAccessKey: 'your-secret-access-key',
},
bucket: 'your-s3-bucket',
role: 'your-sagemaker-role',
environmentVariables: {},
};
// Logger for logging messages
const logger: Logger = console;
// Service and model names
const service = 'your-service';
const model = 'your-model';
// Create an instance of PyTorchDeployment
const pytorchDeployment = new PyTorchDeployment(config, logger, service, model);
// Deployment input configuration
const deployInput = {
frameworkVersion: '2.1',
pythonVersion: 'py310',
entryPoint: 'inference.py',
trainingJobName: 'your-training-job-name',
useGpu: true,
};
// Serverless configuration for the deployment
const serverlessConfig = {
memorySizeInMb: 2048,
maxConcurrency: 10,
};
async function deployModel() {
try {
// Deploy the model
const result = await pytorchDeployment.deploy(deployInput, serverlessConfig);
console.log('Deployment completed:', result);
} catch (error) {
console.error('Deployment failed:', error);
}
}
deployModel();
import { TensorFlowDeployment } from './deploy';
import { Logger } from './interfaces';
// Configuration for AWS and SageMaker
const config = {
region: 'us-west-2',
credentials: {
accessKeyId: 'your-access-key-id',
secretAccessKey: 'your-secret-access-key',
},
bucket: 'your-s3-bucket',
role: 'your-sagemaker-role',
environmentVariables: {},
};
// Logger for logging messages
const logger: Logger = console;
// Service and model names
const service = 'your-service';
const model = 'your-model';
// Create an instance of TensorFlowDeployment
const tensorflowDeployment = new TensorFlowDeployment(config, logger, service, model);
// Deployment input configuration
const deployInput = {
frameworkVersion: '2.12',
pythonVersion: 'py310',
entryPoint: 'inference.py',
trainingJobName: 'your-training-job-name',
useGpu: true,
};
// Serverless configuration for the deployment
const serverlessConfig = {
memorySizeInMb: 2048,
maxConcurrency: 10,
};
async function deployModel() {
try {
// Deploy the model
const result = await tensorflowDeployment.deploy(deployInput, serverlessConfig);
console.log('Deployment completed:', result);
} catch (error) {
console.error('Deployment failed:', error);
}
}
deployModel();
This project is licensed under the MIT License.
FAQs
This package provides utilities for training and deploying machine learning models on AWS SageMaker. It supports multiple ML frameworks including PyTorch, TensorFlow, XGBoost, Scikit-learn, and HuggingFace.
We found that @precise-finance/sagemaker demonstrated a healthy version release cadence and project activity because the last version was released less than a year ago. It has 0 open source maintainers collaborating on the project.
Did you know?
Socket for GitHub automatically highlights issues in each pull request and monitors the health of all your open source dependencies. Discover the contents of your packages and block harmful activity before you install or update your dependencies.
Security News
PyPI now supports iOS and Android wheels, making it easier for Python developers to distribute mobile packages.
Security News
Create React App is officially deprecated due to React 19 issues and lack of maintenance—developers should switch to Vite or other modern alternatives.
Security News
Oracle seeks to dismiss fraud claims in the JavaScript trademark dispute, delaying the case and avoiding questions about its right to the name.