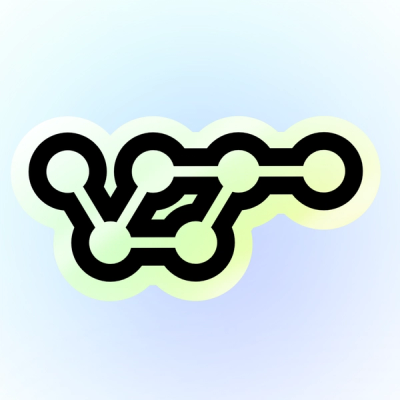
Security News
vlt Debuts New JavaScript Package Manager and Serverless Registry at NodeConf EU
vlt introduced its new package manager and a serverless registry this week, innovating in a space where npm has stagnated.
@stencila/dockter
Advanced tools
✨ Help us choose a better name for this project! ✨
Docker is a useful tool for creating reproducible computing environments. But creating truly reproducible Docker images can be difficult - even if you already know how to write a Dockerfile
.
Dockter makes it easier for researchers to create Docker images for their research projects. Dockter generates a Dockerfile
and builds a image, for your project, based on your source code.
🦄 Dockter is in early development. Features that are not yet implemented are indicated by unicorn emoji. Usually they have a link next to them, like this 🦄 #2, indicating the relevant issue where you can help make the feature a reality. It's readme driven development with calls to action to chase after mythical vaporware creatures! So hip.
Dockter scans your project folder and builds a Docker image for it. If the the folder already has a Dockerfile
, Dockter will build the image from that. If not, Dockter will scan the source code files in the folder and generate one for you. Dockter currently handles R and Python source code (with Node.js support planned). A project can have a mix of these languages.
If the folder contains a R package DESCRIPTION
file then Dockter will install the R packages listed under Imports
into the image. e.g.
Package: myrproject
Version: 1.0.0
Date: 2017-10-01
Imports:
ggplot2
The Package
and Version
fields are required in a DESCRIPTION
file. The Date
field is used to define which CRAN snapshot to use. MRAN daily snapshots began 2014-09-08 so the date should be on or after that.
If the folder does not contain a DESCRIPTION
file then Dockter will scan all the R files (files with the extension .R
or .Rmd
) in the folder for package import or usage statements, like library(package)
and package::function()
, and create a .DESCRIPTION
file for you.
Dockter checks if any of your dependencies (or dependencies of dependencies, or dependencies of...) requires system packages (e.g. libxml-dev
) and installs those too. No more trial and error of build, fail, add dependency, repeat... cycles!
If the folder contains a requirements.txt
file, or a 🦄 #4 Pipfile
, Dockter will copy it into the Docker image and use pip
to install the specified packages.
If the folder does not contain either of those files then Dockter will 🦄 #5 scan all the folder's .py
files for import
statements and create a .requirements.txt
file for you.
If the folder contains a 🦄 #7 package.json
file, Dockter will copy it into the Docker image and use npm
to install the specified packages.
If the folder does not contain a package.json
file, Dockter will 🦄 #8 scan all the folder's .js
files for import
or require
statements and create a .package.json
file for you.
If the folder contains any 🦄 #9 .ipynb
files, Dockter will scan the code cells in those files for any Python import
or R library
statements and extract a list of package dependencies. It will also 🦄 #10 add Jupyter to the built Docker image.
If you have built a Docker image you'll know that it can be frustrating waiting for all your project's dependencies to reinstall when you simply add or remove one of them.
The reason this happens is that, due to Docker's layered filesystem, when you update a requirements file, Docker throws away all the subsequent layers - including the one where you previously installed your dependencies. That means that all those packages need to get reinstalled.
Dockter takes a different approach. It leaves the installation of language packages to the language package managers: Python's pip
, Node.js's npm
, and R's install.packages
. These package managers are good at the job they were designed for - to check which packages need to be updated and to only update them. The result is much faster rebuilds, especially for R packages, which often involve compilation.
Dockter does this by looking for a special # dockter
comment in a Dockerfile
. Instead of throwing away layers, it executes all instructions after this comment in the same layer - thus reusing packages that were previously installed.
Here's a simple motivating example. It's a Python project with a requirements.txt
file which specifies that the project depends upon pandas
which, to ensure reproducibility, is pinned to version 0.23.0
,
pandas==0.23.0
The project also has a Dockerfile
which specifies which Python version we want to use, copies requirements.txt
into the image, and uses pip
to install the packages:
FROM python:3.7.0
COPY requirements.txt .
RUN pip install -r requirements.txt
You can build a Docker image for that project using Docker,
docker build .
Docker will download the base Python image (if you don't yet have it), download five packages (pandas
and it's four dependencies) and install them. This took over 9 minutes when we ran it.
Now, let's say that we want to get the latest version of pandas
and increment the version in the requirements.txt
file,
pandas==0.23.1
When we do docker build .
again to update the image, Docker notices that the requirements.txt
file has changed and so throws away that layer and all subsequent ones. This means that it will download and install all the necessary packages again, including the ones that we previously installed. For a more contrived illustration of this, simply add a space to one of the lines in the requirements.txt
file and notice how the package install gets repeated all over again.
Now, let's add a special # dockter
comment to the Dockerfile before the COPY
directive,
FROM python:3.7.0
# dockter
COPY requirements.xt .
RUN pip install -r requirements.txt
The comment is ignored by Docker but tells dockter
to run all subsequent instructions in a single filesystem layer,
dockter build .
Now, if you change the requirements.txt
file, instead of reinstalling everything again, pip
will only reinstall what it needs to - the updated pandas
version. The output looks like:
Step 1/1 : FROM python:3.7.0
---> a9d071760c82
Successfully built a9d071760c82
Successfully tagged dockter-5058f1af8388633f609cadb75a75dc9d:system
Dockter 1/2 : COPY requirements.txt requirements.txt
Dockter 2/2 : RUN pip install -r requirements.txt
Collecting pandas==0.23.1 (from -r requirements.txt (line 1))
<snip>
Successfully built pandas
Installing collected packages: pandas
Found existing installation: pandas 0.23.0
Uninstalling pandas-0.23.0:
Successfully uninstalled pandas-0.23.0
Successfully installed pandas-0.23.1
Dockter uses JSON-LD as it's internal data structure. When it parses your project's source code it generates a JSON-LD tree using a vocabularies from schema.org and CodeMeta.
For example, It will parse a Dockerfile
into a schema.org SoftwareSourceCode
node extracting meta-data about the Dockerfile.
Dockter also fetches meta data on your project's dependencies, which could be used to generate a complete software citation for your project.
{
"name": "myproject",
"datePublished": "2017-10-19",
"description": "Regression analysis for my data",
"softwareRequirements": [
{
"description": "\nFunctions to Accompany J. Fox and S. Weisberg,\nAn R Companion to Applied Regression, Third Edition, Sage, in press.",
"name": "car",
"urls": [
"https://r-forge.r-project.org/projects/car/",
"https://CRAN.R-project.org/package=car",
"http://socserv.socsci.mcmaster.ca/jfox/Books/Companion/index.html"
],
"authors": [
{
"name": "John Fox",
"familyNames": [
"Fox"
],
"givenNames": [
"John"
]
},
Dockter is designed to make it easier to get started creating Docker images for your project. But it's also designed not to get in your way or restrict you from using bare Docker. You can easily, and individually, override any of the steps that Dockter takes to build an image.
Code analysis: To stop Dockter doing code analysis and take over specifying your project's package dependencies, just remove the leading '.' from the .DESCRIPTION
, .requirements.txt
or .package.json
file that Dockter generates.
Dockerfile generation: Dockter aims to generate readable Dockerfiles that conform to best practices. They include comments on what each section does and are a good way to start learning how to write your own Dockerfiles. To stop Dockter generating a .Dockerfile
, and start editing it yourself, just rename it to Dockerfile
.
Image build: Dockter manage builds use a special comment in the Dockerfile
, so you can stop using Dockter altogether and build the same image using Docker (it will just take longer if you change you project dependencies).
Dockter is available as pre-compiled, standalone command line tool (CLI), or as a Node.js package. In both cases, if you want to use Dockter to build Docker images, you will need to install Docker if you don't already have it.
To install the latest release of the dockter
command line tool, download dockter-win-x64.zip
for the latest release and place it somewhere on your PATH
.
To install the latest release of the dockter
command line tool to /usr/local/bin
just,
curl -L https://unpkg.com/@stencila/dockter/install-latest-macos.sh | bash
Or, if you'd prefer to do things manually, download dockter-macos-x64.tar.gz
for the latest release and then,
tar xvf dockter-macos-x64.tar.gz
sudo mv -f dockter /usr/local/bin
To install the latest release of the dockter
command line tool to ~/.local/bin/
just,
curl -L https://unpkg.com/@stencila/dockter/install-latest-linux.sh | bash
Or, if you'd prefer to do things manually, or place Dockter elewhere, download dockter-linux-x64.tar.gz
for the latest release and then,
tar xvf dockter-linux-x64.tar.gz
mv -f dockter ~/.local/bin/ # or wherever you like
If you want to integrate Dockter into another application or package, it is also available as a Node.js package :
npm install @stencila/dockter
The command line tool has three primary commands compile
, build
and execute
. To get an overview of the commands available use the --help
option i.e.
dockter --help
To get more detailed help on a particular command, also include the command name e.g
dockter compile --help
The compile
command compiles a project folder into a specification of a software environment. It scans the folder for source code and package requirement files, parses them, and creates an .environ.jsonld
file. This file contains the information needed to build a Docker image for your project.
For example, let's say your project folder has a single R file, main.R
which uses the R package lubridate
to print out the current time:
lubridate::now()
Let's compile that project and inspect the compiled software environment. Change into the project directory and run the compile
command.
dockter compile
You should find three new files in the folder created by Dockter:
.DESCRIPTION
: A R package description file containing a list of the R packages required and other meta-data
.envrion.jsonld
: A JSON-LD document containing structure meta-data on your project and all of its dependencies
.Dockerfile
: A Dockerfile
generated from .environ.jsonld
Usually, you'll compile and build a Docker image for your project in one step using the build
command. This command runs the compile
command and builds a Docker image from the generated .Dockerfile
(or handwritten Dockerfile
):
dockter build
After the image has finished building you should have a new docker image on your machine, called rdate
:
> docker images
REPOSITORY TAG IMAGE ID CREATED SIZE
rdate latest 545aa877bd8d About a minute ago 766MB
You can use Docker to run the created image. Or use Dockter's execute
command to compile, build and run your image in one step:
> dockter execute
2018-10-23 00:58:39
We 💕 contributions! All contributions: ideas 💡, bug reports 🐛, documentation 🗎, code 💾. See CONTRIBUTING.md for more details.
There are several other projects that create Docker images from source code and/or requirements files including:
alibaba/derrick
jupyter/repo2docker
Gueils/whales
o2r-project/containerit
openshift/source-to-image
ViDA-NYU/reprozip
Dockter is similar to repo2docker
, containerit
, and reprozip
in that it is aimed at researchers doing data analysis (and supports R) whereas most other tools are aimed at software developers (and don't support R). Dockter differs to these projects principally in that it:
performs static code analysis for multiple languages to determine package requirements (🦄 well, right now just R).
uses package databases to determine package system dependencies and generate linked meta-data (containerit
does this for R).
quicker installation of language package dependencies (which can be useful during research projects where dependencies often change).
by default, but optionally, installs Stencila packages so that Stencila client interfaces can execute code in the container.
reprozip
and its extension reprounzip-docker
may be a better choice if you want to share your existing local environment as a Docker image with someone else.
containerit
might suit you better if you only need support for R and don't want managed packaged installation
repo2docker
is likely to be better choice if you want to run Jupyter notebooks or RStudio in your container and don't need source code scanning to detect your requirements
If you don't want to build a Docker image and just want a tool that helps determining the package dependencies of your source code check out:
detective
modulefinder
requirements
Why go to the effort of generating a JSON-LD intermediate representation instead of writing a Dockerfile directly?
Having an intermediate representation of the software environment allows this data to be used for other purposes (e.g. software citations, publishing, archiving). It also allows us to reuse much of this code for build targets other than Docker (e.g. Nix) and sources other than code files (e.g. a GUI).
Why is Dockter a Node.js package?
We've implemented this as a Node.js package for easier integration into Stencila's Node.js based desktop and cloud deployments.
Why is Dockter implemented in Typescript?
We chose Typescript because it's type-checking and type-annotations reduce the number of runtime errors and improves developer experience.
I'd love to help out! Where do I start?
See CONTRIBUTING.md (OK, so this isn't asked that frequently. But it's worth a try eh :woman_shrugging:.)
Dockter was inspired by similar tools for researchers including binder
, repo2docker
and containerit
. It relies on many great open source projects, in particular:
FAQs
A Docker image builder for researchers
The npm package @stencila/dockter receives a total of 12 weekly downloads. As such, @stencila/dockter popularity was classified as not popular.
We found that @stencila/dockter demonstrated a not healthy version release cadence and project activity because the last version was released a year ago. It has 2 open source maintainers collaborating on the project.
Did you know?
Socket for GitHub automatically highlights issues in each pull request and monitors the health of all your open source dependencies. Discover the contents of your packages and block harmful activity before you install or update your dependencies.
Security News
vlt introduced its new package manager and a serverless registry this week, innovating in a space where npm has stagnated.
Security News
Research
The Socket Research Team uncovered a malicious Python package typosquatting the popular 'fabric' SSH library, silently exfiltrating AWS credentials from unsuspecting developers.
Security News
At its inaugural meeting, the JSR Working Group outlined plans for an open governance model and a roadmap to enhance JavaScript package management.