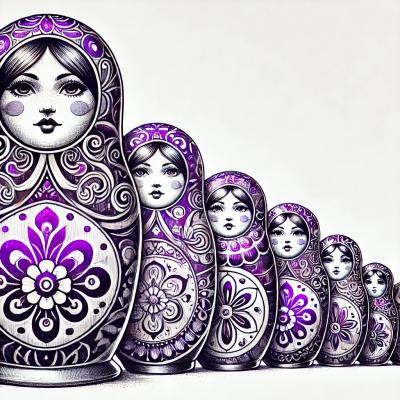
Research
Security News
Threat Actor Exposes Playbook for Exploiting npm to Build Blockchain-Powered Botnets
A threat actor's playbook for exploiting the npm ecosystem was exposed on the dark web, detailing how to build a blockchain-powered botnet.
@tensorflow/tfjs-data
Advanced tools
This repo is under active development and is not production-ready. We are actively developing as an open source project.
TensorFlow.js Data provides simple APIs to load and parse data from disk or over the web in a variety of formats, and to prepare that data for use in machine learning models (e.g. via operations like filter, map, shuffle, and batch).
This project is the JavaScript analogue of tf.data on the Python/C++ side. TF.js Data will match the tf.data API to the extent possible.
To keep track of issues we use the tensorflow/tfjs Github repo with comp:data
tag.
There are two ways to import TensorFlow.js Data
tfjs-data
has peer dependency on tfjs-core, so if you import
@tensorflow/tfjs-data
, you also need to import
@tensorflow/tfjs-core
.Reading a CSV file
import * as tf from '@tensorflow/tfjs';
const csvUrl = 'https://storage.googleapis.com/tfjs-examples/multivariate-linear-regression/data/boston-housing-train.csv';
async function run() {
// We want to predict the column "medv", which represents a median value of a
// home (in $1000s), so we mark it as a label.
const csvDataset = tf.data.csv(
csvUrl, {
columnConfigs: {
medv: {
isLabel: true
}
}
});
// Number of features is the number of column names minus one for the label
// column.
const numOfFeatures = (await csvDataset.columnNames()).length - 1;
// Prepare the Dataset for training.
const flattenedDataset =
csvDataset
.map(({xs, ys}) => {
// Convert xs(features) and ys(labels) from object form (keyed by column
// name) to array form.
return {xs: Object.values(xs), ys: Object.values(ys)};
})
.batch(10);
// Define the model.
const model = tf.sequential();
model.add(tf.layers.dense({
inputShape: [numOfFeatures],
units: 1
}));
model.compile({
optimizer: tf.train.sgd(0.000001),
loss: 'meanSquaredError'
});
// Fit the model using the prepared Dataset
return model.fitDataset(flattenedDataset, {
epochs: 10,
callbacks: {
onEpochEnd: async (epoch, logs) => {
console.log(epoch, logs.loss);
}
}
});
}
run().then(() => console.log('Done'));
FAQs
TensorFlow Data API in JavaScript
The npm package @tensorflow/tfjs-data receives a total of 96,442 weekly downloads. As such, @tensorflow/tfjs-data popularity was classified as popular.
We found that @tensorflow/tfjs-data demonstrated a healthy version release cadence and project activity because the last version was released less than a year ago. It has 10 open source maintainers collaborating on the project.
Did you know?
Socket for GitHub automatically highlights issues in each pull request and monitors the health of all your open source dependencies. Discover the contents of your packages and block harmful activity before you install or update your dependencies.
Research
Security News
A threat actor's playbook for exploiting the npm ecosystem was exposed on the dark web, detailing how to build a blockchain-powered botnet.
Security News
NVD’s backlog surpasses 20,000 CVEs as analysis slows and NIST announces new system updates to address ongoing delays.
Security News
Research
A malicious npm package disguised as a WhatsApp client is exploiting authentication flows with a remote kill switch to exfiltrate data and destroy files.