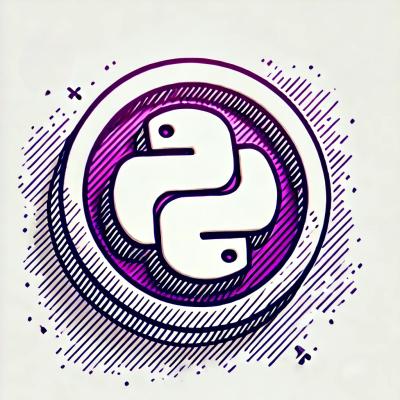
Security News
PyPI Introduces Digital Attestations to Strengthen Python Package Security
PyPI now supports digital attestations, enhancing security and trust by allowing package maintainers to verify the authenticity of Python packages.
retext-keywords
Advanced tools
Keyword extraction with retext.
npm:
$ npm install retext-keywords
[Duo](http://duojs.org/#getting-started):
```javascript
var keywords = require('wooorm/retext-keywords');
var Retext = require('retext');
var keywords = require('retext-keywords');
var retext = new Retext().use(keywords);
retext.parse(
/* First three paragraphs on Term Extraction from Wikipedia:
* http://en.wikipedia.org/wiki/Terminology_extraction */
'Terminology mining, term extraction, term recognition, or ' +
'glossary extraction, is a subtask of information extraction. ' +
'The goal of terminology extraction is to automatically extract ' +
'relevant terms from a given corpus.' +
'\n\n' +
'In the semantic web era, a growing number of communities and ' +
'networked enterprises started to access and interoperate through ' +
'the internet. Modeling these communities and their information ' +
'needs is important for several web applications, like ' +
'topic-driven web crawlers, web services, recommender systems, ' +
'etc. The development of terminology extraction is essential to ' +
'the language industry.' +
'\n\n' +
'One of the first steps to model the knowledge domain of a ' +
'virtual community is to collect a vocabulary of domain-relevant ' +
'terms, constituting the linguistic surface manifestation of ' +
'domain concepts. Several methods to automatically extract ' +
'technical terms from domain-specific document warehouses have ' +
'been described in the literature.' +
'\n\n' +
'Typically, approaches to automatic term extraction make use of ' +
'linguistic processors (part of speech tagging, phrase chunking) ' +
'to extract terminological candidates, i.e. syntactically ' +
'plausible terminological noun phrases, NPs (e.g. compounds ' +
'"credit card", adjective-NPs "local tourist information office", ' +
'and prepositional-NPs "board of directors" - in English, the ' +
'first two constructs are the most frequent). Terminological ' +
'entries are then filtered from the candidate list using ' +
'statistical and machine learning methods. Once filtered, ' +
'because of their low ambiguity and high specificity, these terms ' +
'are particularly useful for conceptualizing a knowledge domain ' +
'or for supporting the creation of a domain ontology. Furthermore, ' +
'terminology extraction is a very useful starting point for ' +
'semantic similarity, knowledge management, human translation ' +
'and machine translation, etc.',
function (err, tree) {
tree.keywords();
/*
* Array[5]
* ├─ 0: Object
* | ├─ stem: "terminolog"
* | ├─ score: 1
* | └─ nodes: Array[7]
* ├─ 1: Object
* | ├─ stem: "term"
* | ├─ score: 1
* | └─ nodes: Array[7]
* ├─ 2: Object
* | ├─ stem: "extract"
* | ├─ score: 1
* | └─ nodes: Array[7]
* ├─ 3: Object
* | ├─ stem: "web"
* | ├─ score: 0.5714285714285714
* | └─ nodes: Array[4]
* └─ 4: Object
* ├─ stem: "domain"
* ├─ score: 0.5714285714285714
* └─ nodes: Array[4]
*/
}
);
Extract keywords, based on the number of times they (nouns) occur in text.
/* See above for an example, and output. */
/* To *not* limit keyword-count: */
tree.keywords({'minimum' : Infinity});
Options:
number
) — Return at least (when possible) minimum
keywords.Results: An array, containing match-objects:
0
and (including) 1
. The first match has a score of 1;WordNode
s.Extract keyphrases, based on the number of times they (one or more nouns) occur in text.
tree.keyphrases();
/*
* Array[6]
* ├─ 0: Object
* | ├─ stems: Array[2]
* | | ├─ 0: "terminolog"
* | | └─ 1: "extract"
* | ├─ score: 1
* | └─ nodes: Array[3]
* ├─ 1: Object
* | ├─ stems: Array[1]
* | | └─ 0: "term"
* | ├─ score: 0.46153846153846156
* | └─ nodes: Array[3]
* ├─ 2: Object
* | ├─ stems: Array[2]
* | | ├─ 0: "term"
* | | └─ 1: "extract"
* | ├─ score: 0.4444444444444444
* | └─ nodes: Array[2]
* ├─ 3: Object
* | ├─ stems: Array[2]
* | | ├─ 0: "knowledg"
* | | └─ 1: "domain"
* | ├─ score: 0.20512820512820512
* | └─ nodes: Array[2]
* └─ 5: Object
* ├─ stems: Array[1]
* | └─ 0: "commun"
* ├─ score: 0.15384615384615385
* └─ nodes: Array[3]
*/
/* To *not* limit phrase-count: */
tree.keyphrases({'minimum' : Infinity});
Options:
number
) — Return at least (when possible) minimum
phrases.Results: An array, containing match-objects:
0
and (including) 1
. The first match has a score of 1;WordNode
s.On a MacBook Air, keywords()
runs about 3,784 op/s on a big section / small article.
A big section (10 paragraphs)
4,026 op/s » Finding keywords
625 op/s » Finding keyphrases
A big article (100 paragraphs)
438 op/s » Finding keywords
59 op/s » Finding keyphrases
FAQs
retext plugin to extract keywords
The npm package retext-keywords receives a total of 582 weekly downloads. As such, retext-keywords popularity was classified as not popular.
We found that retext-keywords demonstrated a healthy version release cadence and project activity because the last version was released less than a year ago. It has 0 open source maintainers collaborating on the project.
Did you know?
Socket for GitHub automatically highlights issues in each pull request and monitors the health of all your open source dependencies. Discover the contents of your packages and block harmful activity before you install or update your dependencies.
Security News
PyPI now supports digital attestations, enhancing security and trust by allowing package maintainers to verify the authenticity of Python packages.
Security News
GitHub removed 27 malicious pull requests attempting to inject harmful code across multiple open source repositories, in another round of low-effort attacks.
Security News
RubyGems.org has added a new "maintainer" role that allows for publishing new versions of gems. This new permission type is aimed at improving security for gem owners and the service overall.