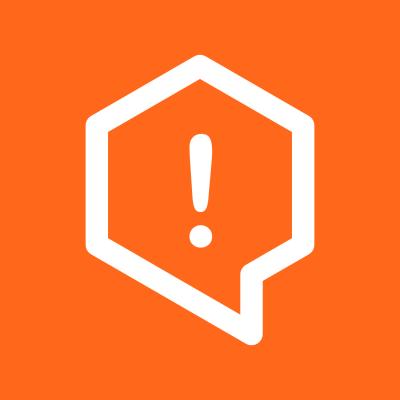
Security News
Fluent Assertions Faces Backlash After Abandoning Open Source Licensing
Fluent Assertions is facing backlash after dropping the Apache license for a commercial model, leaving users blindsided and questioning contributor rights.
Python package for hypothesis testing. Suitable for using in A/B-testing software. Tested for Python >= 3.5. Based on numpy and scipy.
pip install abito
The most powerful tool in this package is the Sample:
import abito as ab
Let's draw some observations from Poisson distribution and initiate Sample instance from them.
import numpy as np
observations = np.random.poisson(1, size=10**6)
sample = ab.sample(observations)
Now we can calculate any statistic in numpy-way.
print(sample.mean())
print(sample.std())
print(sample.quantile(q=[0.05, 0.95]))
To compare with other sample we can use t_test or mann_whitney_u_test:
observations_control = np.random.poisson(1.005, size=10**6)
sample_control = Sample(observations_control)
print(sample.t_test(sample_control))
print(sample.mann_whitney_u_test(sample_control))
Or we can use bootstrap to compare any statistic:
sample.bootstrap_test(sample_control, stat='mean', n_iters=100)
To improve performance, it's better to provide observations in weighted form: unique values + counts. Or, we can compress samples, using built-in method:
sample.reweigh(inplace=True)
sample_control.reweigh(inplace=True)
sample.bootstrap_test(sample_control, stat='mean', n_iters=10000)
Now bootstrap is working lightning-fast. To improve performance further you can set parameter n_threads > 1 to run bootstrapping using multiprocessing.
observations = np.random.normal(100, size=10**8)
sample = ab.sample(observations)
compressed = sample.compress(n_buckets=100, stat='mean')
%timeit sample.std()
%timeit compressed.std()
FAQs
Package for hypothesis testing in A/B-experiments
We found that abito demonstrated a healthy version release cadence and project activity because the last version was released less than a year ago. It has 1 open source maintainer collaborating on the project.
Did you know?
Socket for GitHub automatically highlights issues in each pull request and monitors the health of all your open source dependencies. Discover the contents of your packages and block harmful activity before you install or update your dependencies.
Security News
Fluent Assertions is facing backlash after dropping the Apache license for a commercial model, leaving users blindsided and questioning contributor rights.
Research
Security News
Socket researchers uncover the risks of a malicious Python package targeting Discord developers.
Security News
The UK is proposing a bold ban on ransomware payments by public entities to disrupt cybercrime, protect critical services, and lead global cybersecurity efforts.